You should have heard about Netflix, Microsoft, Zoom, Honda being attacked by hackers recently. Within the last year, the nature of fraud changed a lot. Attackers have changed the type of victims they target, shifting from individuals and small businesses to major corporations. They exploit the disruption the coronavirus has caused and find new security vulnerabilities many companies are now exposed to.
Traditional fraud detection methods don’t work in the new reality. As criminals’ attacks become more sophisticated, the approaches to fraud risk management should also evolve. Discover major types of fraud events that can threaten your business and learn how you can improve fraud detection with big data analytics and machine learning.
Are you prepared for new fraud risks?
According to the report from the Association of Certified Fraud Examiners (ACFE), an average fraud costs an organization more than $1.5M. But the direct and indirect financial burden is only part of the overall impact that a security attack has on a business. Fraud goes beyond financial losses and hampers customer experience, damages company reputation, brings about operational failures, etc.
Since the outset of the pandemic, fraud risks have increased for all companies in every industry. The results of the survey by ACFE reveal that 79% of respondents said they have seen an increase in the overall level of fraud (compared to 77% in August and 68% in May), and 38% noted that this increase has been significant. In 2021, this trend continues to persist.
One of the reasons for the sudden growth of fraud is that employees use their personal devices (phones, tablets, or laptops) to access corporate information. Working from home does not ensure the same level of cybersecurity as an office environment as home Wi-Fi networks are much easier to attack. Also, employees very often do not run an antivirus or anti-malware scan regularly, use weak passwords, forget to connect to VPN, apply online tools that are not approved by the company, etc.
Other reasons, why incidents of fraud are on the rise is that businesses have suddenly moved to the public cloud and increased their usage of IoT devices without dedicating proper attention to security. Due to large online data transactions, weak security controls, inefficient fraud prevention tools, companies across all industries are now experiencing a wide range of fraud events.
How big data analytics and machine learning can help you in fraud prevention
- Save costs and maximize revenue
- Offer better customer experience
- Speed up fraud analysis eliminating manual work
- Identify fraud before it occurs
- Ensure a high fraud detection rate removing false positives
- Help identify and fix weaknesses in the system or business flow.
Many companies across industries have already experienced the benefits of detecting fraud with data analytics and ML. For example, Lebara, a leading UK mobile virtual network operator, has managed to identify and block unlimited SIM cards that were used by fraudsters to call their paid numbers. Deutsche Bank uses big data and AI to improve a KYC process, identify money laundering, prevent credit card fraud, etc. In healthcare, ML&AI are also increasingly utilized to mitigate such fraud risks as fake claims, claims duplication, billing for medically unnecessary tests, fake diagnosis, etc. A healthcare insurance company Santéclair now detects fraud cases three times more effectively after applying data and advanced algorithms.
What stops companies from using big data analytics and machine learning in fraud detection
Legacy approaches to fraud prevention that depend on rule-based methods, siloed data, and manual processes don’t bring tangible results. By contrast, fraud detection with big data analytics and machine learning allows companies to detect, prevent, predict, and remediate fraud quickly and more efficiently.
Frauds can range from simple, easily detectable non-payments to complex crimes like identity theft, e-skimming, Denial of service (DDos) attacks, man-in-the-middle attacks, etc. Companies find it difficult to detect and prevent fraud. In fact, few businesses regularly perform fraud risk assessment and have an established strategy to fraud prevention. Only 56% of organisations conducted an investigation of their worst incident, according to PwC's Global Economic Crime and Fraud Survey 2020. And here come the main reasons why so many companies still do not implement big data analytics and ML for fraud detection company-wide:
- Unsuccessful experience: due to insufficient data, rule-based methods, lack of expertise, etc. , the results of fraud analysis may be not representative and not worth the effort and resources allocated. Companies that once failed to achieve a high fraud detection rate and instead identified only many false positives are reluctant to undertake a new approach and use big data analytics and machine learning in fraud detection.
- Changing fraud patterns: fraudulent schemes are constantly evolving with the development of new tools and technology. Old models that worked in the past may quickly become outdated. Security experts may simply get lost in the sea of tech trends and security tendencies.
- Lack of skilled security professionals: It is challenging to undertake fraud detection with big data analytics and machine learning without a central team of experts or a reliable partner. Demand for cybersecurity skills grows every year. According to BLS, cybersecurity jobs will grow by 31% through 2029. Finding the right security experts is difficult. Companies all over the world report a lack of cybersecurity experts.
- High costs: Companies don’t rush into allocating a lot of money on cybersecurity, as they have concerns about its vague results. But as studies show those who invest in fraud prevention incur lower costs when fraud is experienced. For example, according to the recent survey by PWC, companies with a dedicated fraud program spent 42% less on response and 17% less on remediation costs than those companies with no program in place.
Those companies that hesitate to invest in big data analytics and machine learning for fraud detection will always be one step behind the fraudsters. To reduce the number of false alarms, prevent malicious activity, and be able to quickly investigate fraud, companies need to tap into advanced methods of fraud detection and prevention based on big data and ML.
How to implement big data analytics and machine learning in fraud prevention
-
Get a clear vision of your solution
Before collecting and analyzing data, you need to understand under what circumstances machine learning and big data analytics in fraud detection would be advantageous to your business, what results you want to achieve, how many resources you need to allocate, and what will be your ROI. Thus, you need to conduct a Discovery Phase, which will help you validate your ideas and back your assumptions with actual numbers.
-
Develop a proper big data engineering ecosystem
It allows you to collect, integrate, store, and process huge amounts of data from numerous siloed data sources. You can use tools like Dataflow, Apache Beam, AWS Glue, or Spark to start with big data analysis faster and build your data lake and data warehouse solutions with ease.
-
Prepare your data
Big Data used in fraud analysis come from different sources: web proxies, firewalls, authentication systems, transaction processing systems, payment and billing systems, databases, business applications, etc. You need to undertake data engineering and convert raw data into prepared data. Further, you need to perform feature engineering and tune the prepared data to create the features you will further feed to the learning algorithm to build models. It is important to focus on data quality and quantity, run ETL procedures (extract, transform, and load) on the newly created ecosystem. The bigger and cleaner your training dataset is, the more accurate results you will achieve with machine learning in fraud detection and prevention. Besides data transformation and technical clean-up, data scientists may need to refine the data further to make it suitable for a specific business case.
-
Choose a robust machine learning solution
You can build a scalable and efficient machine learning solution on your own or use third-party ML solutions. Tech giants like Google, Microsoft, Amazon, and IBM sell machine learning software as a service. You can choose between Amazon SageMaker, Amazon ML, Google Cloud AI Platform, Azure Machine Learning Studio, or IBM Watson Machine Learning that provide complete packages with various tools to create and deploy ML models.
-
Apply appropriate algorithms and models
There is no universal machine learning algorithm that will suit all your business cases. Thus, you should constantly fine-tune models before applying them to different business cases and retrain them with new data. There are two types of machine learning approaches that are often applied to detect and prevent fraud: unsupervised and supervised machine learning. They can be used independently or be combined to build more sophisticated algorithms. In unsupervised learning, the data is not labeled. You only have input data and no corresponding output variables. K-Means clustering, Singular value decomposition (SVD), Apriori are the most well-known algorithms used in unsupervised learning. In supervised learning, machines are trained using "labeled" training data, and on the basis of that data, machines predict the output. XGBoost, k-nearest neighbors (KNN), decision trees, random forests are the most popular algorithms used in supervised learning for fraud detection and prevention.
-
Visualize results to get valuable insights
Decision-makers should always get the information they need quickly to be able to make well-informed decisions. It is essential to build interactive dashboards so your data can be easily accessed and analyzed. Thus, you need to apply powerful BI tools to see what's happening behind the scenes and get you clues on how to improve future performance.
How N-iX can help you succeed with fraud detection using machine learning and big data analytics
- N-iX boasts a pool of 2,200+ tech experts ready to support you in every stage of your project.
- N-iX experts have solid expertise in Big Data engineering, Data Science, ML&AI, BI, Cloud, DevOps, and Security.
- N-iX has been repeatedly recognized by CRN among the leading solution providers in North America in their ratings, including Solution Provider 500 and CRN Fast Growth 150.
- N-iX has been recognized by ISG as a Rising Star in data engineering services for the UK market and positioned in the Product Challengers Quadrant both in the data science and data Infrastructure & cloud integration services.
- Our professionals have experience with Apache Spark and Hadoop ecosystems.
- N-iX specialists have a proven track record working with cloud-based tools such as Snowflake, EMR, Dataproc, Cloud Composer, BigQuery, Synapse Analytics, Azure Data Factory, Databricks.
- Our experts work with such big data tools as Amazon Redshift, Hive, Athena for querying data and apply Kafka, AWS Kinesis or Apache Pulsar for real-time big data streaming.
- We have delivered big data and ML solutions for a leading inflight internet provider Gogo, a multinational MVNO Lebara, a British fintech company RateSetter, and many Fortune 500 companies.
- The company complies with international regulations, including ISO 27001:2013, PCI DSS, ISO 9001:2015, GDPR, and HIPAA, so your data will always be safe.
- N-iX is trusted in the global tech market: the company has been listed among the top software development providers by Clutch, in the Global Outsourcing 100 by IAOP for 5 consecutive years, recognized by GSA UK 2019 Awards, included in top software development companies by GoodFirms.co, and others.
- N-iX is a certified AWS Advanced Consulting Partner, a Microsoft gold certified partner, a Google Cloud Platform Partner, an Opentext Services silver partner, and a SAP partner.
Have a question?
Speak to an expert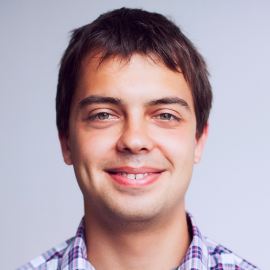