One fact helps explain modern financial regulation—poor data governance in banking was blamed for the 2008 financial crisis. Regulators argue that the lack of traceability and accountability of financial data led to inadequate risk assessment and management across financial institutions. Starting with the Dodd-Frank Act, Basel III, and numerous later data privacy acts, these acts saw their goal of preventing the repetition of the failure of data systems in 2008. Understanding this fact and investing in data governance services can proactively address compliance needs in the long term. Moreover, further benefits are associated with it, such as increased operational efficiency, better decision-making, stronger data security, and higher customer satisfaction. Effective data governance can also open the way for more innovative and forward-looking banking software development and financial software development.
Let’s examine data governance in banking not just as a long-term solution to regulatory compliance but as a step towards more modern financial services.
The core principles of data governance
Compliance audits are the biggest challenge addressed by data governance in finance. 52% of executives have reported difficulties with compliance audits, and 40% have stated that they failed to comply at least once. While it is the most pressing and visible issue, poor data governance also leads to data breaches, overpaying for custom software integration, user dissatisfaction, data loss, and data leaks. All of which can cause significant financial and reputational damage.
The basic principles of data governance that protect banks from these threats are ensuring the accuracy, security, and effective management of financial data across the institution. These principles include:
- Data quality: Ensuring the accuracy, completeness, and reliability of data throughout its lifecycle.
- Data security: Protecting data from unauthorized access, breaches, and theft through robust security measures and protocols.
- Data privacy: Safeguarding personal and sensitive information in compliance with legal and regulatory requirements, such as GDPR and CCPA.
- Data accessibility: Making data available to authorized users in a timely and efficient manner to support decision-making and operational processes.
- Data lifecycle management: Implementing policies and practices for the creation, storage, archiving, and deletion of data to maintain its relevance and compliance.
- Risk management: Identifying, assessing, and mitigating data management and security risks.
- Stakeholder engagement: Involving key stakeholders in developing and implementing data governance policies and practices to ensure alignment with business objectives.
- Accountability and responsibility: Establishing clear roles and responsibilities for data governance to ensure accountability across the organization.
- Transparency: Maintaining openness about data governance practices, policies, and procedures to build trust among stakeholders and regulatory bodies.
From the IT perspective, data governance in banking involves establishing policies, procedures, and controls for data quality, privacy, and security; implementing data management technologies and systems; and ensuring that data across the organization is consistent, accessible, and properly used. It also involves the adoption of best practices for data architecture, storage, and disaster recovery, as well as the integration of new technologies to improve data analytics and reporting capabilities. Essentially, IT-driven data governance focuses on maximizing the value of data as a strategic asset while minimizing associated risks and costs.
Read more: 7 steps to effective Data Governance strategy
Key components of data governance
Data governance is a crucial component of digital transformation, dramatically improving banks' operations and service delivery. According to McKinsey, an average corporation's employee spends 29% of their time on non-value-added tasks because of poor data availability [1]. Creating a system that guarantees all employees know exactly where to locate the information required for their tasks and who to contact for inquiries or to report issues is fundamental for any further innovation. Let's look at the four key components of data governance in banking and see which business needs they fulfill.
Data quality management
Data quality management is a set of practices aimed at ensuring the accuracy, completeness, timeliness, and reliability of the data. This includes metadata management, essentially data about data, for example its elements, structures, lineage, definitions, and policies.
It is followed by Master Data Management (MDM) and Common Data Model (CDM). The goal of MDM is to provide and maintain a consistent, accurate, and holistic view of an organization's core business entities. It aims to consolidate data from disparate systems, databases, and spreadsheets into a single, coherent system. CDM, on the other hand, focuses on establishing a standardized data schema that can be used across different applications and systems. Its primary goal is to ensure data consistency and interoperability by providing a common understanding of data formats, definitions, and structures.
This centralized approach creates a single version of truth, meaning that all users within the organization have access to the same information and can be confident in its legitimacy. Such consistency is crucial for operations, analytics, and reporting purposes.
Data accountability and traceability
Data stewardship is an important concept in data governance that is crucial for creating a culture of accountability and transparency around data management. Data stewards are intermediaries between IT and business units, ensuring that data quality is up to the established standard. In principle, data stewardship creates actors within the organization who are interested in and can be held accountable for data management. This helps mitigate data-related risks and maximize the value of data assets. Appointing data stewards alone doesn't fulfill the accountability cycle. Real accountability in data governance goes beyond the operational level. It needs senior management's active involvement.
The sophistication and complexity of the accountability and management structures around data governance depend on the data they will govern. Banks are considered to be enterprises with the highest level of data complexity with an additional challenge of regulatory maneuvers. However, the governance infrastructure's exact scale varies with the bank's size. For instance, a global banking giant typically establishes a high-level data governance council, often including C-suite executives, to guide governance initiatives. This setup involves advanced automation for managing data, with every piece of metadata meticulously cataloged in an enterprise-wide dictionary or data catalog and data stewards at every data source.
Data architecture and integration
Data architecture is the set of underlying systems and structures that store, manage, and facilitate the flow of data within the organization. Effective data architecture ensures that data is consistent, accessible, and structured in a way that supports operational and analytical processes. Integration mechanisms also play a critical role in ensuring that data across different sources and systems can be effectively combined and utilized.
In data management, there are many data architecture classifications; financial institutions often prioritize the development of data warehouses. These are sophisticated systems designed to organize data not just by content but by relevance and frequency of access. This means data is structured to optimize retrieval times and analytical efficiency. However, the plan for such an architecture is not a one-size-fits-all. For example, should it be a one-, two-, or three-tier architecture?
Creating the optimal data architecture is crucial in the banking sector. It will allow to maximize the utility of the vast data banks hold, enhancing ability to generate insights, ensure compliance, and ultimately deliver superior value to customers and stakeholders. Additionally, a well-designed data architecture supports the scaling of data operations and integration of new technologies, enabling banks to adapt to changing market demands.
Read more: Enterprise data warehouse: From raw data to unified analytics
Data lifecycle management
Data lifecycle management encompasses the processes and policies involved in managing the flow of an organization's data from creation or acquisition to retirement and disposal. It includes defining how data is collected, processed, stored, archived, backed up, and protected at each stage, ensuring data quality, accessibility, and compliance.
Integrating various data streams to form a unified and comprehensive view is essential for a deep understanding of processes, such as the customer lifecycle. This holistic approach enables the extraction of valuable insights, which can be leveraged to tailor personalized solutions that mitigate customer churn and enhance the bank's ability to identify and capitalize on cross-selling opportunities. Executives whose institutions have implemented data analytics and personalization report almost a threefold increase in the ability to cross-sell and attract new customers [2]. Taking advantage of personalization can significantly elevate a financial institution's status among financial innovators and enhance customer loyalty.
Read more: Enhancing services with ML-powered churn prediction calculation
Data privacy and security
The need for data security and privacy is a common thread across all listed components. The Federal Reserve Bank reports that roughly 75% of all less-than-satisfactory supervisory findings were linked to governance and cybersecurity issues [4]. Modern financial risks arise not in asset management but in data management. Banks have to safeguard against breaches, leaks, and losses. This is achieved through robust cybersecurity measures, data encryption, access controls, and regular security audits. Here are some examples of data security tools:
- Data classification tools help categorize data based on its sensitivity and relevance to privacy regulations.
- Access control systems ensure that only authorized personnel can access sensitive data. They employ measures such as user authentication, role-based access control (RBAC), and least privilege principles to minimize the risk of unauthorized data exposure.
- Encryption and Data Masking obscure specific data within a database, hiding sensitive information from users without the necessary access rights.
- IDPS (Intrusion Detection and Prevention Systems) can help detect and prevent breaches by identifying suspicious activities that could indicate a security threat.
Together, these systems form a comprehensive approach to data security within the broader context of data governance.
WHITE PAPER
Stay ahead in banking: explore top 6 tech trends shaping the industry in 2024.
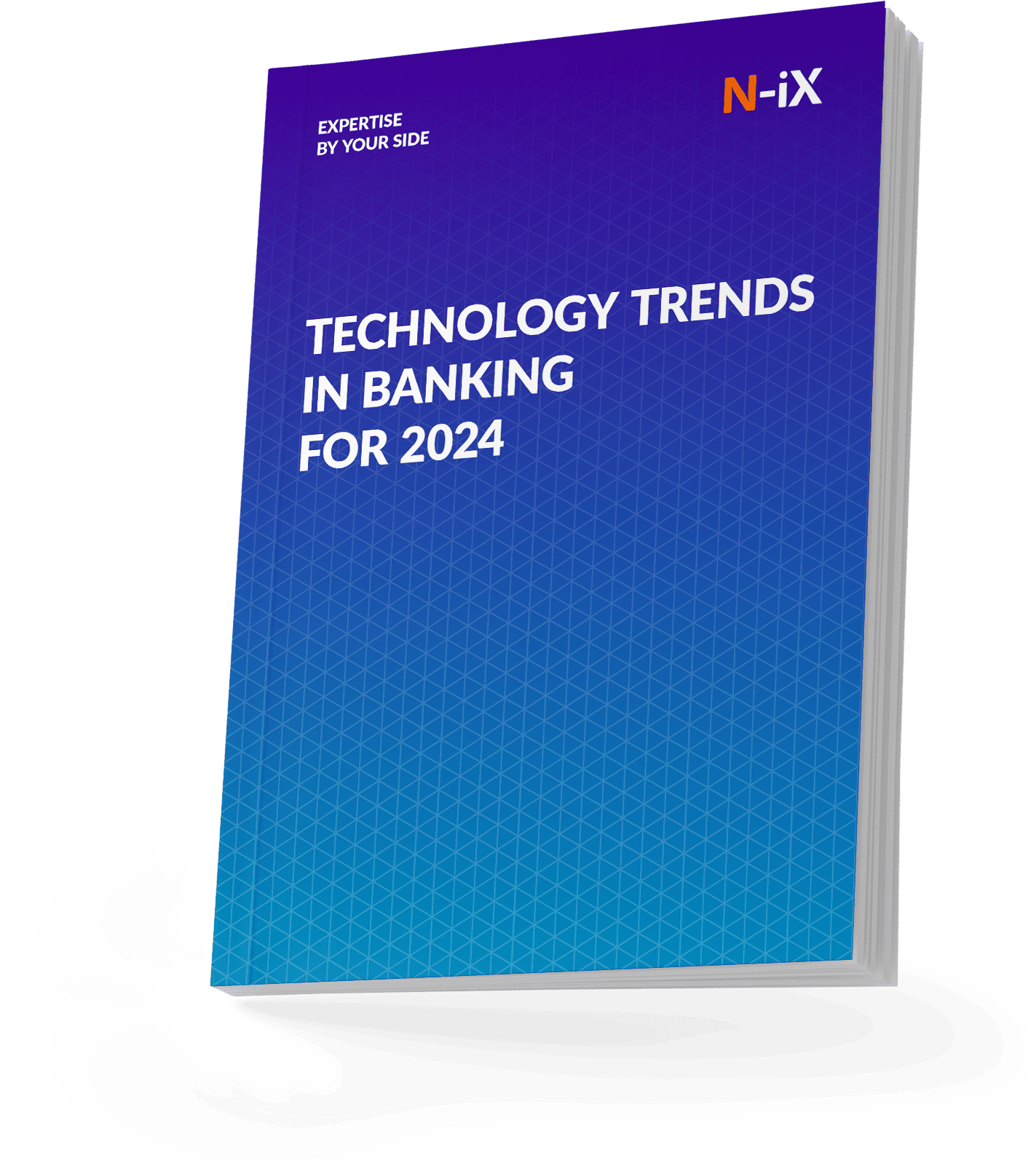
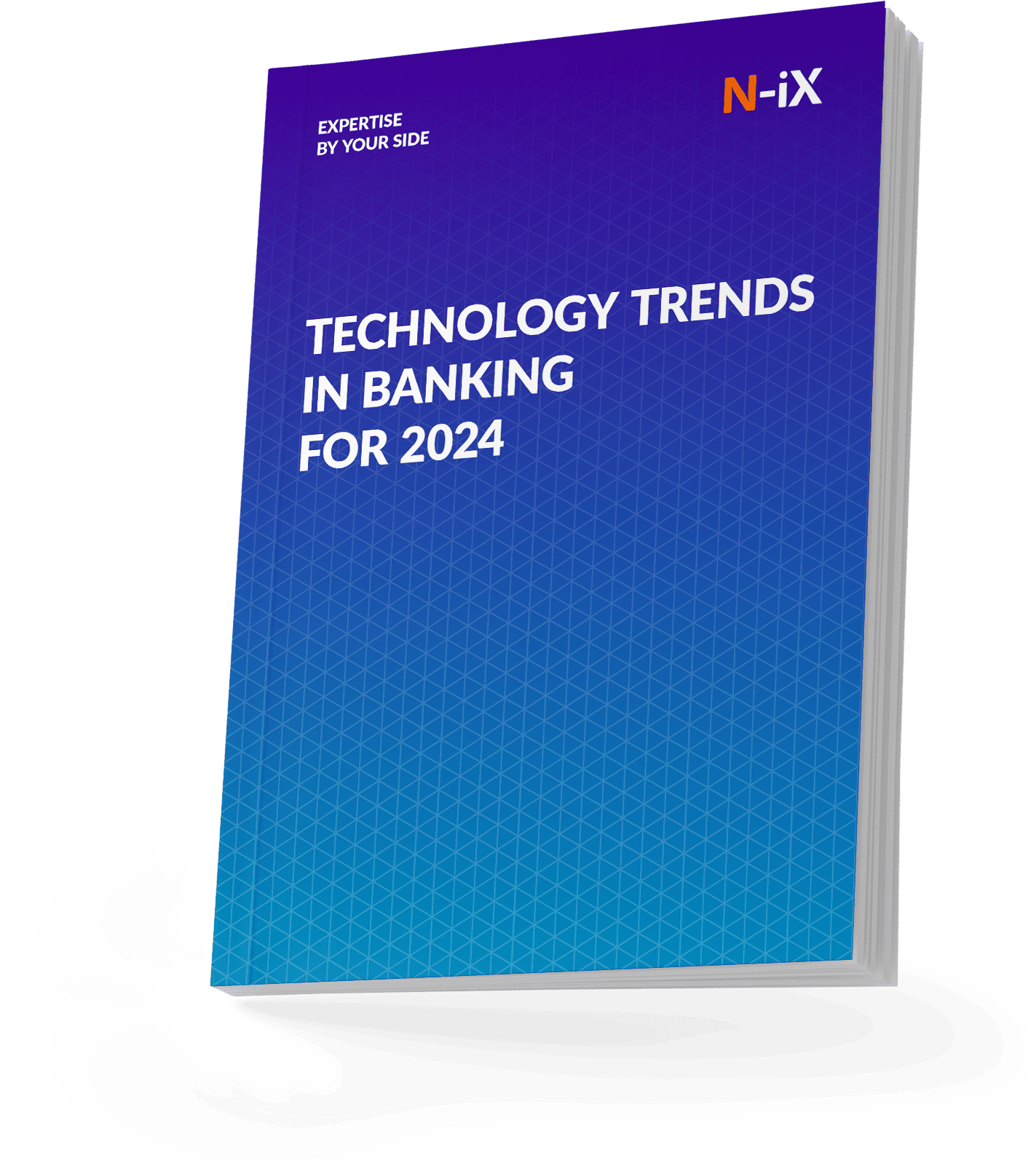
Success!
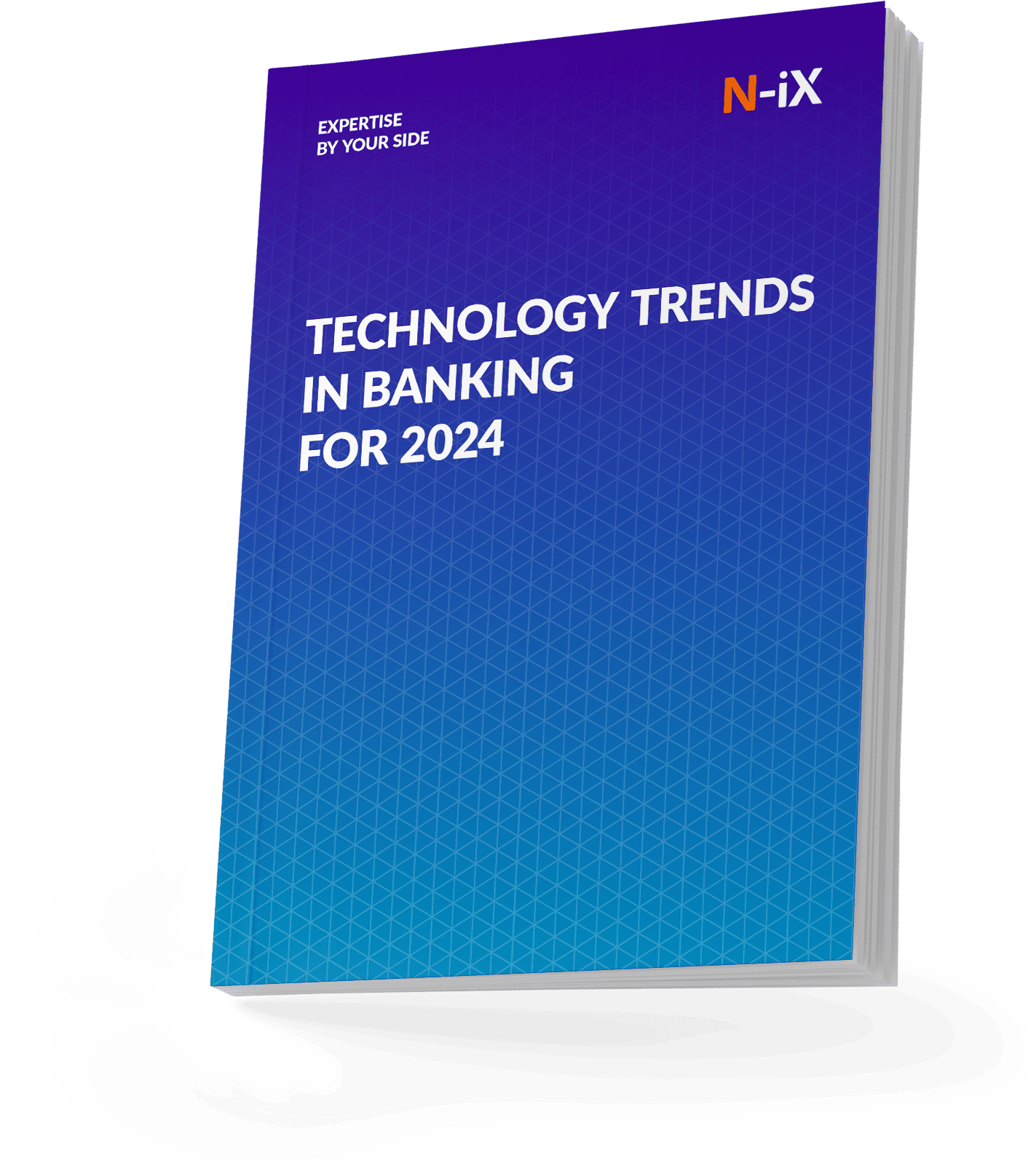
How N-iX helps build data governance in banking
N-iX collaborated with an established fintech company to fortify its cybersecurity through the implementation of robust data governance measures. Employing our expertise in data management and security protocols, N-iX crafted a tailored strategy to regulate data access, usage, and storage, ensuring compliance with industry standards and regulations. Our client gained better control and understanding of its data assets, reducing possible risks and weaknesses. This joint effort improved the company's cybersecurity and also increased trust among its stakeholders.
Read more: Driving growth in e-commerce with a comprehensive data analytics solution
Wrap up
The image of banks stockpiling heaps of gold, oblivious to the treasures they possess, may be an exaggeration. However, the metaphor fittingly reflects the state of data utilization within the banking sector. The industry sits atop a treasure trove of underutilized data, which has the potential to significantly enhance employee productivity, improve customer services, and facilitate informed decision-making. The journey towards comprehensive data governance is not merely a pathway to regulatory compliance but a strategic prerequisite to unlocking the full spectrum of idle opportunities lost in the heaps of abundance.
Why choose N-iX for building comprehensive data governance in banking?
N-iX is a trusted partner for building comprehensive data governance in banking:
- N-iX has a robust team of over 300 data governance consultants and engineers who have successfully completed dozens of data projects and helped multiple organizations establish effective data governance.
- N-iX establishes frictionless cooperation between your teams with data catalogs and a clear distinction of who can access and use which data.
- N-iX ensures that all data security regulations, such as GDPR, or PCI DSS, are met across your organization.
- N-iX has over 150 certified data and cloud experts and is an official partner of all major cloud service providers.
- N-iX has received many industry recognitions, such as a “Rising star in data engineering” by ISG or a spot in the Global Outsourcing 100.
- N-iX complies with established service quality and data protection standards, such as GDPR, HIPAA, PCI DSS, ISO 9001:2015, and ISO 27001:2013.
References:
- Designing data governance that delivers value, McKinsey
- Unlocking Hyper-Personalization At Hyper-Scale, Forrester 2023
- Digital Banking Ebook, Finshape 2023
- Supervision and Regulation Report, FRB 2023
Have a question?
Speak to an expert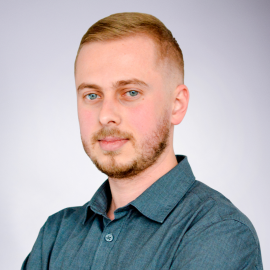