Why telecoms need Big Data Analytics
Telecoms are facing a highly saturated mobile market and intense competition. To gain a competitive advantage and develop a strong customer value proposition, forward-thinking players are increasingly adopting telecom analytics, Big Data, and Data Science. With the natural abundance of data in daily telecom operations, exploring enterprise big data solutions becomes a crucial step in this transformation.
Source: GSMA Intelligence, 2019
In the face of such a vicious battle, only those with a competitive advantage (other than reduced price) will succeed, while others will end up as completely commoditized utility service providers.
To stop losing money, get a cutting edge over the competition, and develop a strong customer value proposition, most forward-thinking players choose to adopt telecom analytics, Big Data, and Data Science. And it is only logical as large quantities of data are a natural part of daily telecom operations, and they are going up. For example, the amount of data that is transferred through AT&T's wireless network has increased 470,000% since 2007.
Therefore there are many telecoms that have already adopted Big Data and Telecom Analytics such as Lebara, AT&T, CenturyLink, China Mobile, Spectrum, Sprint, Swisscom, Telia, T-Mobile, and Vodafone.
And there are even more telecom businesses that are planning to do so. The telecom industry is expected to invest $36.7 billion annually in AI-related software, hardware, and services by 2025, according to Thractica. According to the Global telecommunications study: navigating the road to 2020, “Improve big data & analytics capabilities” was rated number four, with nearly 40% of respondents selecting it as a priority when they were asked how they are going to boost their organizational agility.
Benefits Big Data and Predictive Analytics can bring to telecoms:
- Making smarter investment decisions
- Visibility into the profitability of different departments
- Reducing fraud
- Improved risk management
- Increased sales
- Smoother network operation
- Enhanced customer experience and reduced churn rate
- Cutting off operations that drain the budget
- Making the operations more efficient
- Increased average revenue per user
What are the most promising use cases of Big Data and telecom analytics?
Reducing fraud
Telecom fraud is a key reason for lost revenue in the telecom industry. For example, the annual cost of telecommunications subscription fraud is estimated to be up to more than $12B, while others foresee it to be between 3 and 10 percent of the operators’ gross revenues. To tackle this problem, telecoms use fraud detection systems that are based on data mining algorithms to tag fraudulent customers and suspicious behavior in almost real-time.
Another highly threatening fraud for telecoms is international revenue share fraud (IRSF). For example, according to the Communications Fraud Control Association (CFCA), the telecom industry lost around $4B to international revenue share fraud (IRSF). IRSF is caused by the artificial inflation of traffic or traffic pumping to the premium-rate numbers in the world. The fraud is performed by hackers or fraudsters who generate traffic and PRN (Premium Rate Number) aggregators who obtain a range of fraudulent premium-rate numbers.
N-iX helps telecoms to solve this problem and avert risks by building IRSF detection systems. The systems use Machine Learning algorithms to drop/block up to 3000 calls and save 1M annually.
Network operations monitoring and management
Network operations monitoring and management is one of the strategic use cases for Mobile Network Operators (MNOs). According to Thractica research, network operations monitoring and management are estimated to represent 61% of the sector’s AI spending between 2016 and 2025. And it is only logical as predictive analytics helps forecast traffic patterns and peak period routing, and therefore helps to ensure the smooth running of network operations. For example, telecoms can predict the periods of heaviest network usage arising from video streaming and alleviate congestion during these periods. As a result, telecoms can reduce their planned capital expenditures by 15 percent.
It also helps to predict weather impacts on the infrastructure ( for example, AT&T created a special Climate Change Analysis Tool). What’s more, it goes a long way towards guiding workflows, optimizing the allocation of technicians, and providing real-time updates on the network.
Improving customer experience
Another crucial use case of Big Data and predictive analytics in telecom is enhancing the customer experience. For example, marketing departments of telecoms use clustering and unsupervised machine learning to segment the audience and subsequently target individual customers. Developing a library of over 50 offers and then setting up a mechanism for rapidly launching and measuring the related campaigns, the telecom can reduce churn by 10–15% over the following 18 months.
Contact centers of telecoms can also use predictive analytics based on customer information, technical logs, internal knowledge articles, service tickets, and input from experts to speed up call resolution and improve customer experience.
Churn prediction
For churn prediction, telecoms use the data on customers that have already churned and their behavior before the churn happened to forecast the churn of the existing customers and take effective preventive actions. This is done with the supervised machine learning (we teach the model that users who are about to churn can be spotted by certain predictors and characteristics). Telecoms can collect and use their own data or use an available dataset on the IBM’s website as well as on other pages like Kaggle: Telecom Customer Churn Dataset (the raw dataset contains more than 7,000 entries).
Other profitable use cases of Telecom Analytics include:
- Dynamic pricing to limit congestion and increase profits
- Bundle allocation to increase sales
- Marketing expenditures optimization to help marketing managers and product managers take decisions based on what works and what does not.
- Monitoring the profitability of different departments and the company overall to help the financial department take data-driven decisions.
However, adopting Telecom Analytics, Big Data, and Data Science doesn’t necessarily mean benefiting from it. The key to success lies in the effective utilization of these technologies. For more comprehensive insights and services in this domain, telecoms can explore a broad range of data analytics services to capitalize on their data potential fully.
Here are a few sobering stats by McKinsey:
When we plotted the performance figures for the 80 companies (exhibit), we found that in a few of them, big data had a sizable impact on profits, exceeding 10 percent. Many had incremental profits of 0 to 5 percent, and a few experienced negative returns.
So why do some succeed and others fail when adopting Big Data analytics in telecom?
In the same survey, those that experienced negative returns stated as the key reasons for such results: poor quality of data, lack of specialists, and inability to scale up their big data activities.
How N-iX helps telecoms adopt Big data analytics
Establishing clear business KPIs
In spite of all the tangible benefits of telecom analytics, telecoms often have a hard time extracting the real value from Big Data, Data Science, and Machine Learning. Time and again, they face such challenges as the lack of business KPIs. This, in turn, results in unrealistic estimates and drains budgets. It is not enough to have a suitable software infrastructure in place (although that would be a good start). It takes a clear vision and solid technical talent. You need to have a clear understanding of how it is going to help your business objectives. This is a task for data engineers and data scientists. They investigate the business case and help you formulate viable KPIs and make realistic estimates.
In each business case, you may need a different approach and different expertise. In some cases, you may need to focus on Big Data engineering and Business Intelligence to produce almost real-time and lucid reports and monitor the profitability of different departments or prevent fraud. In other cases, you will need to apply algorithms to the already collected and publicly open set of data as some companies do for predicting churn rate. Or, you may need to combine Big Data engineering and applying the ML models to the collected and preprocessed dataset as companies often do for preventive maintenance or audience segmentation.
Each business case of telecom analytics needs to be considered separately after defining your key directions and your strengths that are set to be your differentiation strategy.
Ensuring robust Big Data engineering and effective Data Science
Whatever strategy you use, the data is the key here. The larger the dataset is, and the cleaner the data is, the more accurate the results are.
Any Big Data Analytics project is about collecting a great amount of data from dispersed sources, cleaning, aggregating, preprocessing it, in some cases, applying Data Science or Machine Learning models, and visualizing the insights.
However, the biggest part of any Big Data analytics project comes down to building an orchestrated ecosystem of platforms that collect siloed data from hundreds of sources like CRMs, connected devices, web logs, reporting software, and more.
And before applying any algorithms or visualizing it, you need to have the data appropriately structured and cleaned up. Only then, you can further turn that data into insights. In fact, ETL (extracting, transforming, and loading) and further cleaning of the data account for around 80% of any Big Data analytics time.
Effective Big Data engineering is a foundation of actionable and accurate business insights in any industry
For example, Gogo, a global inflight connectivity provider, teamed up with N-iX to perform a complete transition of Gogo solutions to the cloud and build a unified data platform based on AWS that aggregates all the data from different sources using Spark. We’ve helped the client to migrate their not scalable expensive on-premise data solutions to the cloud, optimize operations, streamline the system of predicting failures and replacing devices (reducing the number of not-fault-founds by 8 times), and improve the customer experience based on the big data analysis results.
In some business cases, generating almost real-time reports is vital for taking effective business decisions, without any delays. That’s why many businesses, including telecoms, invest in building Data Lakes that allow storing both structured and unstructured data and real-time data streaming.
For this purpose, Lebara and N-iX Big Data engineers have been working on building a Data Lake. That’s vital for the financial department to take timely decisions, without 2-3 months’ delays. Also, it helps to track the churn rate and performance of marketing campaigns and promotions. On top of that, N-iX Big Data engineers provide data collection and analysis of all payments, including disputes, chargebacks, and payment fraud.
Another important application of a Data Lake is almost real-time tracking of the bandwidth usage.
Lebara like all MVNOs( Mobile Virtual Network Operators) does not own the wireless network infrastructure and therefore buys the messages, voice minutes and data from MNOs (Mobile Network Operators). If the ‘package’ is underused within the month - the unused bandwidth goes to waste, and when the ‘package’ is overused by MVNO users, MVNO’s are forced to pay large fines to MNOs(Mobile Network Operators). Therefore, a guessing game here can cost great losses, and the ability to track almost-real time the dynamics of the currently used bandwidth helps to save a lot.
Streamlining Business Intelligence development and reporting
Besides collecting and processing large amounts of data, it’s extremely important to produce intelligible, timely and lucid reports that provide different departments of telecoms with actionable insights.
Lebara has partnered with N-iX to optimize their telecom analytics and BI development. The reports are sent to end-users of different Lebara’s departments in 5 countries. A sales department uses them to see how the department performs; a financial department - to see what is going on with the client base and whether the business generates profits or not; a marketing department - to see how marketing campaigns perform. Also, the BI reports are used for providing the specific info to different state institutions (police, for example), as it is defined by the state laws.
Have a question?
Speak to an expert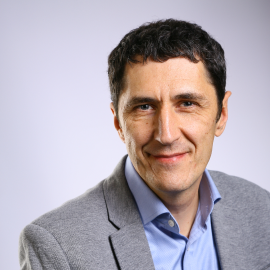