Gaining a decisive competitive advantage in ecommerce is about making the customer experience ever more convenient, smooth, and entertaining. Natural Language Processing (NLP) is precisely a technology in retail software for understanding customers' needs, requests, and barriers to a flawless shopping experience. Unsurprisingly, NLP in ecommerce is a powerful tool for improving customer satisfaction and boosting communication efficiency. However, as generative AI continues gaining traction, it may be challenging to separate proven effective use cases from those yet to mature. Let’s look at the top five most developed and potent use cases of NLP in ecommerce.
Five most impactful use cases of NLP
While compiling the list, we considered the technological advancement of a specific use case and its potential to deliver substantial benefits to businesses and consumers. According to Gartner, NLP generative technology (not just understanding but replying back) will approach its maximum potential in 2-5 years [1]. It is significantly ahead of all other applications of AI excluding computer vision. At this point, it is possible to say that these five use cases are becoming strongly integrated into the retail industry:
Chatbot customer service
NLP-powered chatbots are the number one use case of NLP in ecommerce. Compared to previous generations, they are far better at understanding and processing human language to answer questions and solve problems. While more complex issues and nuances in human communication still pose challenges, the current NLP technology is highly effective for a broad range of customer service tasks in ecommerce.
Key benefits of this use case of NLP in ecommerce include significantly lowering operational costs, minimizing the risk of human error, and instantly analyzing all the necessary data. A seamless and helpful customer service experience is vital, as 94% of customers [2] claim that a positive experience is a significant reason to return. Additionally, people are simply embarrassed to ask “stupid questions” of a real person and would much prefer that they be handled by a bot [3] even if they need help.
The technology behind NLP in chatbots varies widely, ranging from simple rule-based systems to more sophisticated AI-driven models that learn and adapt from interactions. Rule-based chatbots operate on predefined pathways and responses, suitable for handling straightforward and predictable queries. On the other hand, AI chatbots employ machine learning and deep learning to understand context, make decisions, and learn from past interactions to improve their responses over time.
Enhanced semantic NLP search
Semantic search uses NLP in ecommerce to analyze and grasp a user's query's intent and contextual meaning rather than simply matching keywords. This way, customers are more likely to find exactly what they're looking for, even if their query phrasing isn't exact or uses colloquial language.
Search technology is one of the biggest funnels in ecommerce: 69% of customers bypass browsing and go directly to the search function, and 80% will abandon the website if they can't find what they need through search on the first try [4]. A semantic search function may not retain all 80% of the customers, as some may search for items the vendor does not stock. However, it will successfully retain a substantial portion of those customers who simply need to be accurately matched with the available products.
As with chatbots, NLP search technology varies in complexity. Some systems use basic synonym replacement techniques to broaden the search scope. More advanced models are based on deep learning technology and are able to grasp subtle nuances in language, understand complex queries, and anticipate related products or concepts.
Semantic search technology powered by NLP is quite advanced in many sectors, including ecommerce. Companies like Amazon and Google have been refining these technologies for years, making them highly effective in understanding and processing natural language queries to improve the shopping experience.
SEO content optimization
NLP’s ability to process human language is also beneficial for SEO optimization on the website, in product descriptions, and more. It can analyze keywords, phrases, and the context within which they are used and suggest how to optimize content to match user queries, thereby improving search engine rankings. Furthermore, NLP can assess content quality, suggest keywords based on search trends, and generate readable and engaging content that aligns with SEO best practices.
Keyword extraction, sentiment analysis, topic modeling, and language generation are some key NLP techniques utilized in SEO. As for maturity, NLP technologies have significantly advanced in recent years, particularly with the development of models like BERT by Google. While still evolving, these tools are already highly effective and widely adopted in the ecommerce sector for enhancing SEO strategies.
Engagement
NLP radically democratizes the cost of dialogue between brands and consumers on social media platforms. NLP systems can analyze customer inquiries, sentiments, and preferences, allowing brands to offer personalized engagement at scale. This can include answering comments or inquiries, planning thematic content, or automating announcements. This efficient management of high-volume interactions ensures customers feel acknowledged and appreciated, fostering brand loyalty and trust. Naturally, a model can't replace a creative team with their unique style and humor. However, for more formal businesses with streamlined interaction guidelines, it can significantly enhance efficiency.
Sentiment analysis
The churn rate in ecommerce is very high: more than 70% of customers never come back [5]. This suggests that retailers have much to gain by understanding the reasons behind it. Sentiment analysis through NLP is a powerful tool for understanding customer emotions and opinions from large volumes of text data. This data typically includes product reviews, social media posts, customer feedback, and discussion forums. This enables brands to gain insights into customer satisfaction and product reception, which can drive strategic decisions such as product enhancements, targeted marketing campaigns, and improved customer service approaches.
Basic sentiment analysis models categorize sentiments into positive, negative, and neutral groups. In contrast, more advanced systems use machine learning algorithms to recognize subtle emotional undertones and contextual meanings. They can thus evaluate which elements of the customer experience are most and least positively rated.
The maturity of sentiment analysis technology varies; basic sentiment analysis is mature and widely implemented across various platforms. However, more sophisticated analysis requiring deep contextual understanding or detecting sarcasm and irony so common on social media is still under development and refinement.
Read more: 15 NLP companies that can help build your own solution
N-iX’s experience implementing NLP solutions
On the market for over 21 years of experience, N-iX has successfully implemented NLP in various projects across different industries. We have created several conversational AI solutions that enhance customer experience and engagement by providing quick and accurate responses to inquiries. N-iX developed a sales assistant chatbot powered by the OpenAI API for a global software engineering services provider. We also ensured the security of sensitive data, protected the sales assistant from malicious activity, and implemented robust firewalls.
Read more: Improving user experience and client engagement in IT with generative AI
In another successful partnership, N-iX experts helped a Global Fortune 100 multinational engineering and technology company to develop an NLP search and categorization engine for their logistics platform, radically increasing the speed of item classification and location.
Read more: Driving logistics efficiency with industrial Machine Learning
References:
- Gartner Hype Cycle for generative AI, 2023
- AI-Driven Business Optimization in Consumer Goods, PSFK 2023
- Chatbots and Virtual Shopping Assistants: How Generative AI Enhances Customer Support, Ecommerce Germany 2024
- Nosto
- Real-time Customer Lifetime Value (CLV) Benchmark Report, Omniconvert
WHITE PAPER
Unleash the power of Generative AI in ecommerce—explore top use cases in our white paper!
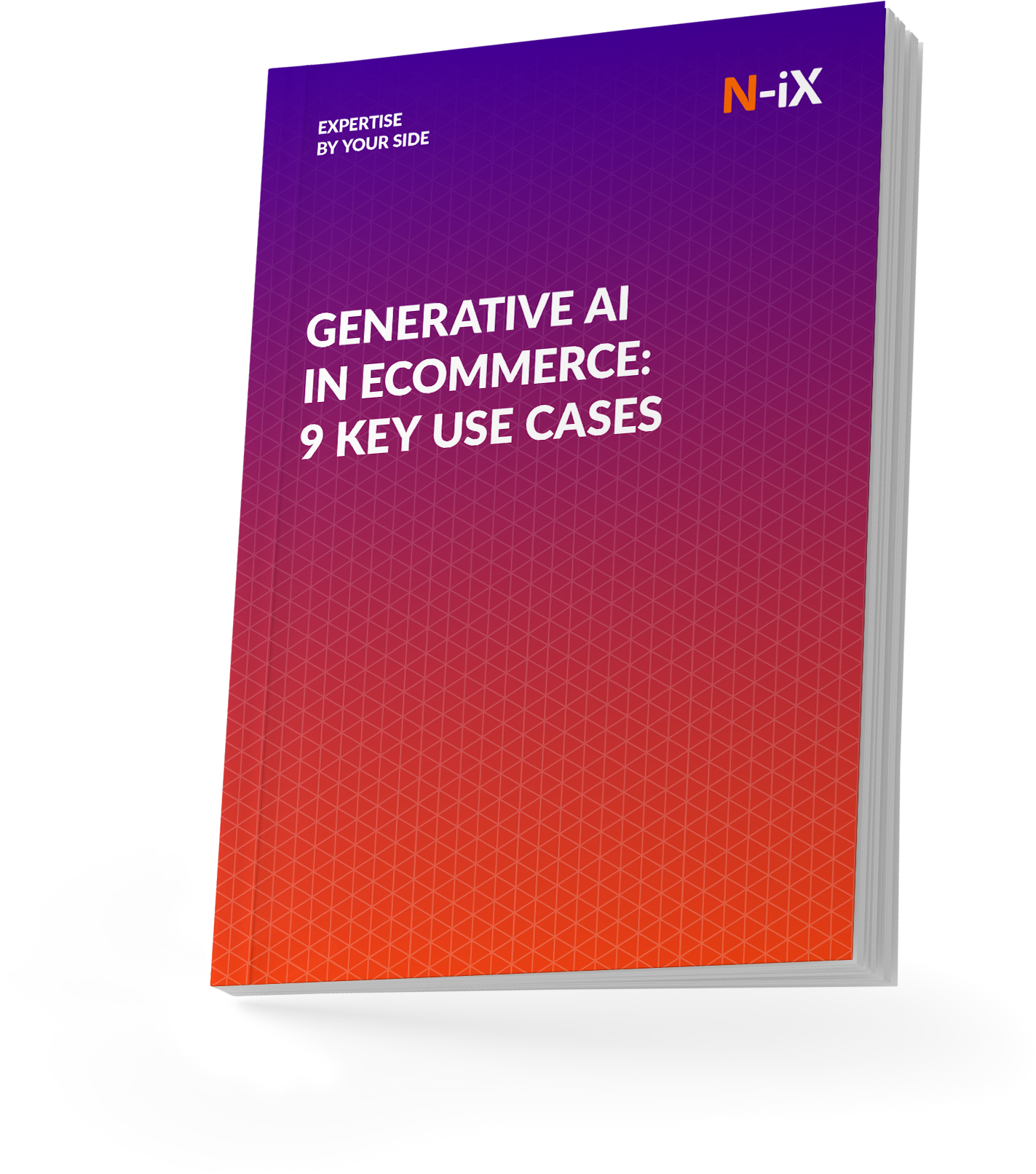
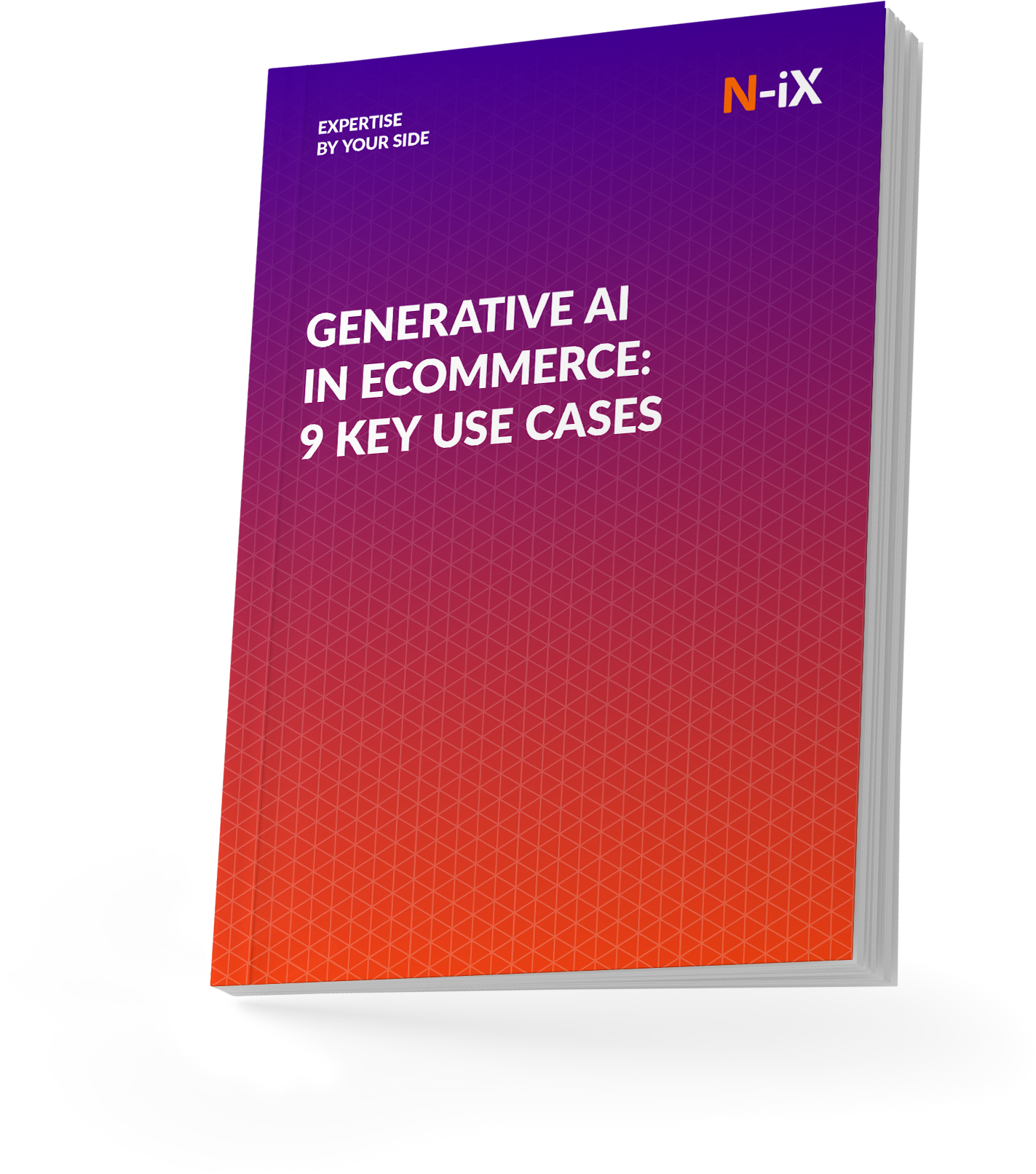
Success!
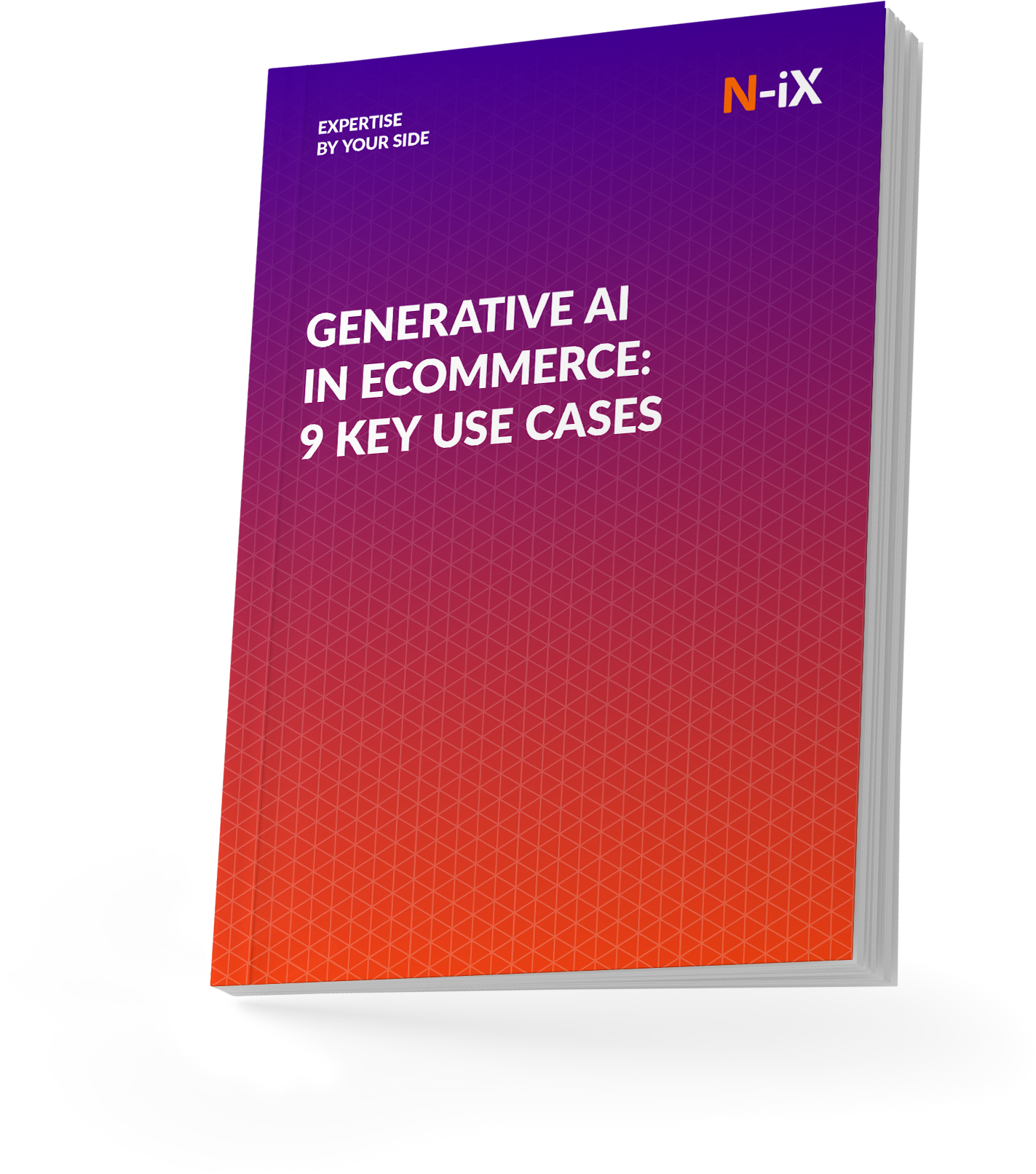