Both generative AI and predictive AI drive innovation across various industries–be it healthcare, finance, or retail. Both wield enormous transformative potential, yet they serve different purposes and offer unique advantages depending on the application.
Generative AI excels at generating new content or simulating human speech. Predictive AI, on the other hand, shines in analyzing existing data to forecast future events, thereby enabling informed decision-making, cost reduction, and risk mitigation.
So, how do you determine which Artificial Intelligence approach is the right fit for your business objectives? Let’s discover each type's functionalities, strengths, and ideal use cases. Whether you're a startup aiming to disrupt the market or an established enterprise looking to adopt generative AI, understanding the nuanced differences between generative AI and predictive AI could be the key to unlocking your next wave of growth.
Generative AI vs predictive AI: Purpose and functionalities
Generative AI and predictive AI are two branches of AI that serve distinct purposes, but both hold significant value in modern technology applications.
At a high level, generative AI is focused on creating new content or data that wasn't in the original dataset. It can create anything from written text to images and music, simulating human-like creativity. For instance, Generative Adversarial Networks (GANs) can produce realistic images, while models like GPT-4, Claude, or Bard are designed to generate human-like text.
Meanwhile, predictive AI aims to forecast outcomes based on existing data. These models analyze historical data to make accurate predictions about future events. For example, predictive algorithms are commonly used in stock market forecasting, weather prediction, and even in predicting customer behavior in retail.
While generative AI excels at creating new, original content, its applications can be broader, from entertainment to simulating scenarios for training purposes. For example:
- Generative AI could be used in the automotive industry to create virtual environments to test autonomous vehicles.
- Predictive AI, conversely, is essential for making data-driven decisions. For instance, in healthcare, it can be used to predict the likelihood of disease outbreaks, thus enabling preventative measures.
Although both types are grounded in data analysis, they differ in the direction of their focus: Generative AI works 'forward' to create something new. In contrast, predictive AI works 'backward' to understand what has already occurred and make educated forecasts. Let’s take a closer look at the use case comparison.
Generative AI vs predictive AI use cases
The use cases for generative AI and predictive AI are as diverse as they are impactful, cutting across various industries and operational needs. Regarding generative AI use cases, which we’ve discussed in a dedicated article, they span the types of inputs and industries. The graph below shows the primary use cases of generative AI from the angle of an application layer.
Industry-wise, the following applications are worth to be highlighted:
- Content creation in marketing. Generative AI can produce unique and engaging marketing content, such as blog posts or social media updates.
- Drug discovery. In pharmaceuticals, generative models can design new molecular structures for potential medicines.
- Virtual training environments. For sectors like aviation or healthcare, generative AI can create realistic simulation scenarios for training. These virtual environments offer safe, cost-effective ways to train professionals in complex tasks.
- Personalized customer interactions. Generative AI can craft personalized email responses or customer service messages based on individual user behavior and history.
- Automated code generation. In software development, generative models can write snippets of code or programs based on specific requirements, allowing for 45% faster software development with generative AI coding support.
Predictive AI, in contrast, is primarily used for forecasting and decision-making. Some of the most fascinating predictive AI use cases are:
- Demand forecasting in retail. Supply chain managers face various challenges, including supplier unpredictability and logistics disruptions. According to Gartner’s report, 60% of chief supply chain officers are expected to make faster, more accurate, and consistent decisions. Thus, predictive AI helps retailers anticipate customer demand for specific products, enabling them to manage inventory more efficiently. Demand forecasting reduces stockouts and overstocking.
- Healthcare risk assessment. In healthcare, predictive models can forecast the likelihood of disease outbreaks or patient readmissions. This approach allows for preventative measures and optimized resource allocation.
- Fraud detection in finance. AI algorithms can analyze transaction patterns to identify potentially fraudulent activities. Early detection allows companies to take preventative actions, reducing financial loss.
- Energy consumption prediction. For utility companies, predictive AI can forecast energy usage patterns. This helps in optimizing grid operations and promoting energy-saving initiatives to consumers.
- Customer churn prediction. In subscription-based services, predictive models can identify customers most likely to churn. Businesses can then implement retention strategies targeting these high-risk customers.
- Predictive maintenance in manufacturing. AI models can assist in forecasting when machinery is likely to fail, allowing for maintenance before a costly breakdown occurs. This increases operational efficiency and extends the machinery's lifespan.
- Weather forecasting for agriculture. Predictive models can forecast weather conditions, helping farmers make informed decisions about irrigation and harvesting.
- Natural disaster prediction. AI can analyze environmental data to predict the likelihood of natural disasters like earthquakes or hurricanes. Early warnings can enable better preparedness, potentially saving lives and reducing property damage.
It's also worth noting that these two types of AI often complement each other in hybrid applications. Thus, the real transformative power often lies in integrating Generative and predictive AI to solve complex, multifaceted problems.
Generative AI vs predictive AI limitations
Both AI solutions have limitations that can impact their effectiveness and applicability. For generative AI enterprise solutions, one primary concern is the quality of the generated content. While these algorithms can create new data or content, they can sometimes produce unrealistic or nonsensical outputs. This is especially concerning in business decision-making scenarios, where inaccuracies can have severe consequences.
Predictive AI, on the other hand, is heavily reliant on the quality and quantity of historical data. The predictive model will likely produce inaccurate or misleading results if the existing data is biased or incomplete. Moreover, these models often struggle with predicting 'black swan' events that have never occurred in the past. The predictive models can also be complex and opaque, making it difficult to interpret their decisions—a drawback often referred to as the "black box" problem.
Additionally, both types of AI are vulnerable to overfitting, where the model performs exceptionally well on the training data but poorly on new, unseen data. This issue can undermine the generalizability and utility of the AI model in real-world applications. Furthermore, both approaches require careful data management, as problems like data leakage can compromise the model's integrity.
Predictive AI and generative AI implementation: Success stories
Are you interested in the significant impact of generative AI and predictive AI? We are preparing to share case studies that serve as benchmarks for operational efficiency and customer engagement.
First, we will discuss how our Data team has assisted Gogo in improving in-flight broadband connectivity using predictive maintenance AI. Following that, we will discuss our work with Cleverbridge, focusing on how we used generative AI to strengthen their customer retention strategy.
Please continue reading to learn how these companies, in partnership with N-iX, have turned challenges into long-term success.
Implementation of predictive maintenance AI for Gogo
Gogo, a leading global provider of in-flight broadband Internet with over 20 years of experience and over 1,000 employees, offers superior technology and best-in-class service.
Gogo faced issues with its satellite antennas often malfunctioning, leading to penalties and a need to enhance the quality of in-flight Internet.
Predictive maintenance models:
Using Data Science and Machine Learning algorithms, our Data team developed models to forecast satellite antenna failures. The designed solution:
- Provided clear reasoning for recommendations;
- Facilitated accurate failure predictions (over 90%) 20-30 days in advance.
Value delivered:
- Cost efficiency. Cloud migration reduced expenses on licenses and on-premises servers and optimized resource management.
- Reduced failures. Implementing predictive maintenance led to a 75% decrease in no-fault-found cases, saving money by avoiding unnecessary servicing.
- Advanced failure prediction. Ensured timely servicing during optimal periods, like when planes were not scheduled for flights.
- Failure mitigation. Identified and addressed typical failure factors, such as issues caused by antifreeze fluid, by implementing additional protective measures.
Gogo's adoption of predictive maintenance AI has minimized costs and significantly enhanced the reliability and efficiency of its in-flight broadband services.
Read more: Big Data analytics for improved maintenance and flawless operation of the in-flight internet
Automation of marketing interventions with generative AI for cleverbridge
Cleverbridge, based in Germany, is a distinguished client specializing in e-commerce, subscription management, and monetization of digital products across various industries. Their platform consolidates solutions for billing, customer experience enhancement, and global compliance.
Cleverbridge needed to lower its customer attrition rate and increase Customer Lifetime Value (CLTV)
Objectives:
- Predict subscription cancellations using Machine Learning (ML);
- Tailor communication strategies;
- Utilize generative AI for custom marketing content creation.
Our solution:
- Customized ML system. Developed an ML solution specifically for Cleverbridge, ensuring seamless integration.
- Multi-tenant solution. Predicts subscription cancellations and recommends effective communication strategies using AI.
- MLOps integration. Facilitates ongoing support and integration.
- Automated marketing responses. Assisted in automating responses based on churn propensity, enhancing retention.
- Data-driven methodology. Expedited content creation and reduced manual efforts.
- Personalized interactions. Resulted in more individualized and efficient customer engagement.
Value delivered:
- Improved efficiency. By leveraging AI and ML, Cleverbridge's marketing became more agile, minimizing manual dependencies.
- Increased personalization. The solution reflected the core values of Cleverbridge and N-iX by ensuring tailored and effective communication.
- Retention improvement. Automation and data-driven strategies led to a noticeable enhancement in customer retention.
Our partnership with Cleverbridge in implementing Machine Learning and AI for marketing addressed the challenge of customer attrition. It elevated the quality of communication, reflecting both companies' commitment to innovation and customer-centric solutions.
Bottom line
Adopting predictive and generative AI goes beyond simply keeping pace with the competition—it opens doors to leadership and groundbreaking innovation. At N-iX, we're not just a vendor but your strategic partner in navigating this technological frontier. Leveraging our deep AI and Machine Learning knowledge, we empower your business to transform obstacles into sustainable value, streamlined operations, and enhanced revenue.
Have a question?
Speak to an expert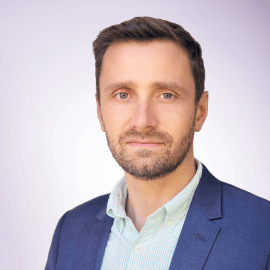