Generative AI solutions can address the labor costs inherent in this services-heavy industry and ensure the sustainability of healthcare organizations. AI systems generate new insights, predict outcomes, and even create solutions to complex medical challenges by leveraging advanced algorithms and vast amounts of data. For instance, generative AI can assist in optimizing treatment plans for patients with chronic conditions, predicting disease progression, or even personalizing medication regimens based on individual genetic profiles.
While current applications primarily focus on cost-saving and automation in back-office operations, the ultimate goal is improving healthcare professionals' and patients’ experience.
Let's explore applications of generative AI in healthcare through real-world examples.
What is generative AI in healthcare?
Generative AI presents the capabilities of more accurate diagnoses, personalized treatment plans, and drug discovery. While certain operations in healthcare, such as managing relationships with healthcare systems, often necessitate a human touch, these processes can be augmented by generative AI.
Core administrative and corporate functions and interactions with patients and healthcare providers typically involve sorting through extensive logs and data, a labor-intensive manual task. Generative AI can summarize this data, regardless of volume, freeing up valuable time.
According to a recent report, the global generative AI in the healthcare market is projected to reach $21B by 2032. As a result, in the near future, stakeholders such as insurance companies, hospital administrators, and leaders within physician groups are poised to integrate generative AI across various aspects of healthcare delivery and management, from patient care to administrative processes.
Generative AI in healthcare: 8 use cases
Through advanced algorithms and data analysis, generative AI holds promise in addressing critical challenges across various domains. Below are several key use cases where generative AI can make significant contributions:
Medical imaging analysis
Generative AI technology is increasingly used to assist radiologists in accurately and swiftly identifying and diagnosing diseases from X-rays, MRIs, and CT scans.
These models trained on diverse patient data identify early signs of various health conditions, including skin and lung cancers, Alzheimer's disease, and diabetic retinopathy, uncover biomarkers indicative of specific disorders, and predict disease progression. Generative AI not only speeds up the diagnostic process but also improves accuracy, leading to earlier detection and better patient outcomes.
Thirty-five researchers introduced a pancreatic cancer detection technology (PANDA). With the help of AI in CT screening CT, PANDA achieved higher accuracy rates in identifying pancreatic cancer than the average radiologist. With a proper CT scan analysis accuracy exceeding 92.9% for cancer-positive cases and 99.9% for non-cancer cases, PANDA presents a cost-effective method for analyzing large groups of asymptomatic patients.
Drug discovery and development
Generative AI is used in pharmaceutical research to enhance drug discovery and development processes. AI models can generate chemical compounds with desired properties by analyzing molecular structures and biological data, such as efficacy and safety profiles.
It enables efficient analysis of extensive datasets, swiftly identifying promising specimens for clinical trials, optimizing molecular structures, and predicting potential side effects and interactions.
The clinical drug development process remains intricate and time-consuming, often taking 12-15 years and costing billions of dollars. Thanks to generative AI, the development timeline for pulmonary fibrosis drugs was reduced to 30 months for the pre-clinical phase.
Another example is Atomwise, which expedites the identification of potential compounds for various diseases by predicting drug candidates. Artificial Intelligence proposed 40,000 novel potential chemical compounds within a mere six hours.
Personalized medicine
Generative AI synthesizes large volumes of patient data, including electronic health records, genomic information, and clinical notes, to create personalized treatment plans.
By analyzing diverse datasets, generative AI algorithms can identify patterns, predict disease trajectories, and recommend optimal treatment strategies based on a patient's characteristics and medical history. This personalized approach to healthcare maximizes treatment efficacy while minimizing adverse effects, leading to improved patient outcomes.
Generative AI processes wearable device data to identify trends and anomalies and monitor vital health indicators such as heart rate variability and blood glucose levels., facilitating early intervention and personalized treatment plans.
Clinical trial optimization
Generative AI-enabled technology can streamline health insurance processes, particularly prior authorization and claims processing, which are typically time-intensive and costly for private payers. Current prior authorization verification processes take an average of ten days.
This technology converts unstructured data into structured formats, enabling near-real-time benefits verification. This includes accurately calculating out-of-pocket costs by incorporating healthcare providers' contracted rates, patients' specific benefits, and other relevant factors.
Streamlined healthcare operations
Beyond clinical applications, generative AI offers significant potential for reducing administrative burdens in healthcare settings. According to Accenture, AI can potentially enhance 40% of healthcare providers' working hours.
So, how does generative AI enhance operational efficiency?
-
Transcribing and summarizing patient consultations, filling EHR fields, and generating clinical documentation.
-
Optimizing appointment scheduling by analyzing patient needs and doctor availability.
-
Creating personalized appointment reminders, follow-up emails and reviewing medical insurance claims to predict rejections.
-
Composing patient feedback surveys, analyzing responses, billing, and providing insights to enhance care delivery.
A study published in Mayo Clinic Proceedings demonstrated how surgeons utilized an LLM to produce high-quality notes meeting clinical standards in mere seconds, typically consuming seven minutes. This represents an 84-fold increase in efficiency, delivering satisfactory outcomes for patients and physicians alike.
Virtual assistants and chatbots
Virtual health assistants offer patients convenient access to healthcare services through conversational interactions. Integrated with major electronic health record systems, AI chatbots allow patients to schedule, reschedule, join waitlists, or cancel appointments without relying on human schedulers.
Virtual assistants employ AI algorithms to deliver personalized medication reminders and adherence alerts to patients. It supports medication adherence and compliance by sending timely notifications via mobile apps or smart devices, reducing the risk of treatment interruptions and adverse outcomes.
For instance, consider a scenario where a patient seeks approval from their doctor to engage in a new physical activity following a recent arthritis diagnosis. When faced with conflicting online advice based on disease progression and age, an AI chatbot could respond, considering their medical history.
Read more: Generative AI use cases and applications
Restoration of lost capabilities
AI offers promising solutions for restoring lost capabilities, such as speech or movement, by interpreting signals from the brain or nerves. This advancement has the potential to revolutionize neurotechnology and rehabilitation in healthcare.
Bioelectronic medicine researchers implant microchips into the brains of individuals with tetraplegia and use AI algorithms to reconnect the brain with the spine. This digital bridge has enabled paralyzed individuals to regain movement in their hands and experience sensations again.
Researchers at the GrapheneX-UTS have developed a system that enables paralyzed individuals to communicate by decoding their silent thoughts into text. This technology is life-changing for individuals who have lost the ability to speak due to illness or injury and holds potential applications in human-machine communication, such as controlling bionic hands.
Medical training and simulations
Generative AI facilitates lifelike simulations of diverse health scenarios, empowering medical professionals and students with risk-free training opportunities. Through AI-powered training and treatment simulations, healthcare professionals can practice new skills and enhance their knowledge interactively. These technologies are often integrated with VR/AR headsets to simulate real-life experiences further.
Western Michigan University has incorporated simulations into its medical studies curriculum, offering students over 100 hours of simulation training. These simulations provide realistic scenarios of patients facing common medical situations and exhibiting specific symptoms. Moreover, students receive feedback from professors at the university's Simulation Center after each simulated medical intervention.
Challenges of implementing generative AI in healthcare
From data privacy concerns to compliance requirements, healthcare organizations must address various hurdles. In this section, we delve into the key challenges of integrating generative AI into healthcare systems.
Potential biases
The performance of AI models lies in the quality and representativeness of the data used for training. If the dataset is not diverse and inclusive, it can lead to biases against underrepresented groups. Generative AI tools, which rely on vast amounts of data from patient records, can inherit such biases.
To address this, healthcare organizations must implement rigorous protocols for algorithm development, validation, and ongoing testing algorithms for biases and diversify training data.
Data privacy
Generative AI in healthcare relies on vast amounts of data, including sensitive patient information, to train models and generate insights. As a result, data privacy and security concerns are significant challenges.
To safeguard patient privacy, healthcare organizations must adhere to strict data protection regulations, implementing encryption, access controls, and auditing mechanisms to protect data from unauthorized access or breaches. Furthermore, healthcare providers must establish clear policies for data sharing and ensure that data used for AI purposes is anonymized or de-identified whenever possible to minimize privacy risks.
Compliance requirements
The deployment of generative AI in healthcare is subject to regulatory scrutiny and compliance requirements. Healthcare organizations must navigate complex regulatory frameworks to ensure that AI solutions adhere to industry standards and guidelines, obtain regulatory approvals for AI algorithms, adhere to medical device regulations (such as FDA approval), and comply with data protection laws.
Integration with existing healthcare systems
Healthcare organizations often operate with legacy systems that may not be compatible with AI technologies. Moreover, AI implementation can disrupt established workflows and processes, leading to resistance from healthcare professionals.
Organizations must thoroughly assess their IT infrastructure, identify integration points for AI solutions, ensure interoperability between AI systems and existing healthcare IT systems and provide training and support to staff members to familiarize them with AI tools and workflows.
Accuracy concerns
As generative AI models continue to evolve, they may occasionally produce incorrect results, a phenomenon known as hallucination in AI. In critical areas such as cancer diagnosis, the accuracy of AI recommendations is vital. Healthcare providers must prioritize transparency in their AI models and emphasize the importance of human review of generated outputs to ensure accuracy.
However, navigating these challenges can be daunting without the right expertise and support. At N-iX, with over 30 successful AI projects delivered and a team of 200+ certified experts in data science and AI, we specialize in overcoming such hurdles effectively.
Our Generative AI consulting approach follows a step-by-step process to validate feasibility, find technical solutions, verify technology compatibility, and implement clear project plans.
Read more: AI trends that will redefine 2024
Final thoughts
As generative AI advances, we can expect to see even greater integration of AI technologies into healthcare systems worldwide. This includes advancements in medical image analysis, virtual assistants, and personalized medicine, among other areas.
Effective integration of generative AI into healthcare relies on carefully balancing its potential benefits with inherent risks. Leaders must thoroughly assess each potential application, comparing its advantages against potential hazards.
82% of healthcare providers have already implemented or plan to implement governance and oversight structures specifically for Generative AI. Optimize operations and enhance patient care through generative AI—contact N-iX today.
With a team of over 2,200 professionals spanning 25 countries across Europe and the Americas, N-iX delivers expert solutions in cloud computing, data analytics, embedded software, IoT, AI, machine learning, and various other tech domains.
N-iX has collaborated with leading tech companies implementing generative AI solutions to address complex challenges. At N-iX, our diverse portfolio of healthcare solutions encompasses full-cycle software product development for healthcare, biotech, and life science sectors. From developing sophisticated Machine Learning algorithms to integrating AI libraries like TensorFlow and Caffe, we enable predictive analytics and personalized medicine powered by generative AI.
Have a question?
Speak to an expert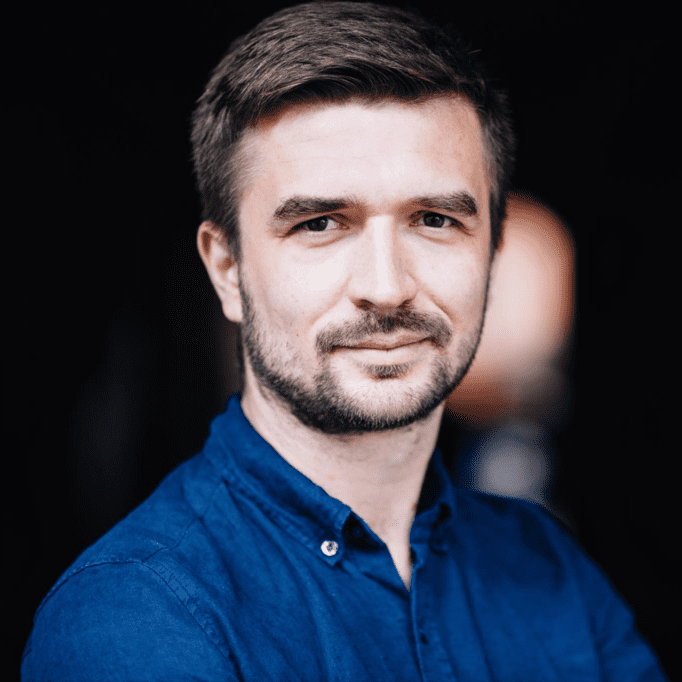