Companies are flooded with vast amounts of data from various sources, leading to issues like data silos, poor data quality, limited accessibility, inconsistency, and inefficiency. To address these issues, the implementation of data hubs stands out as a crucial solution, offering a structured approach to data management and integration.
The problem gets worse when considering the rapid pace of technological advancement and the increasing importance of data analytics in decision-making. Organizations struggle to integrate, process, and analyze their data effectively, missing valuable insights that could drive competitive advantage. Unlike traditional data warehouses or data lakes, enterprise data hubs offer a flexible and scalable solution for storing, processing, and analyzing data. According to Gartner, 80% of organizations will apply for data warehouse consulting to deploy enterprise data hubs by 2025.
Let's now explore how to implement enterprise data hubs successfully and get maximum value from them.
What is an enterprise data hub?
An enterprise data hub (EDH) is a centralized platform designed to aggregate, store, process, and analyze vast volumes of data from disparate sources within an organization. It is a unified repository that enables organizations to efficiently manage their data assets, regardless of the data's type, format, or source.
Unlike a data lake, which primarily stores raw, unprocessed data, or a data warehouse that holds structured, processed data for specific analytical purposes, an EDH integrates both functionalities with enhanced data management and analytics capabilities. It provides not only storage but also advanced processing and analytical capabilities, enabling more flexible and comprehensive data management and utilization.
At its core, an EDH functions as a robust data management solution that addresses the challenges associated with the proliferation of data sources, data fragmentation, and data silos within organizations. The data hubs provide a comprehensive and holistic view of an organization's data landscape by consolidating data from various systems, applications, and sources into a single, centralized hub.
Let’s take a look at the examples of the urgency of implementing an EDH:
- If different departments or teams maintain copies of the same data;
- If an organization relies on data from diverse sources;
- If there is a lack of proper data governance processes;
- If data volumes grow exponentially;
- If organizations seek to derive actionable insights from their data, such as predictive; analytics, machine learning, or real-time analytics.
Read more: Enterprise data warehouse: From raw data to unified analytics
Components of EDH architecture
To understand a data hub comprehensively, it's essential to break down the data transmission process into distinct layers, each responsible for specific tasks. Let's delve into each layer and explore the key functions it fulfills:
- Data ingestion: This is the entry point for data coming into the hub from various sources, such as internal systems, external partners, and cloud services. The data ingestion process handles the initial collection and preliminary data processing, ensuring it's in a usable format for further analysis.
- Data storage: At the core of the data hub is a scalable and secure storage system that accommodates both structured and unstructured data. This layer often includes a combination of data warehouses, data lakes, and NoSQL databases, providing a flexible environment for storing vast information.
- Data integration: The data integration layer of an EDH is responsible for ingesting, processing, and transforming data from diverse sources into a standardized format suitable for analysis and consumption. This layer may encompass data ingestion tools, Extract-Transform-Load processes, data pipelines, and data integration frameworks to streamline data movement into the EDH.
- Data processing: This layer applies more advanced processing and transformation to the data. It includes batch processing for large datasets and stream processing for real-time data flows. The processing layer is crucial for data cleansing, normalization, and enrichment, preparing the data for analysis.
- Data management and governance: This aspect of the architecture ensures data quality, security, and compliance. It includes tools and policies for data lifecycle management, metadata management, access control, and data lineage tracking, helping organizations maintain data integrity and adhere to regulatory standards.
- Analytics and reporting: Leveraging the processed and managed data, this layer provides business intelligence tools, analytics platforms, and reporting solutions. It enables users to generate insights through dashboards, visualizations, and advanced analytics techniques like machine learning and predictive modeling.
Key benefits of adopting an enterprise data hub
Let's review some key benefits of integrating a data hub into your business infrastructure.
Centralized data management and accessibility
One of the key benefits of implementing an EDH is the ability to centralize data management, leading to improved accessibility and visibility of organizational data assets. By consolidating data from different sources into a single repository, data hubs provide users with a unified view of data, making it easier to locate, access, and analyze information. Beyond that, data hubs often include robust metadata management capabilities, allowing users to understand the context and lineage of data.
Scalability to handle large volumes of data
Data hubs are designed to scale horizontally, allowing them to handle growing data volumes and diverse data types efficiently. Leveraging distributed computing and storage technologies, data hubs can seamlessly expand to accommodate increased data ingestion rates, storage capacity, and processing capabilities. This scalability ensures organizations can effectively manage and analyze their data as they grow without experiencing performance bottlenecks or disruptions.
Improved data quality and consistency
Data hubs incorporate data quality management features, such as data profiling, cleansing, and standardization, to ensure that data is accurate, consistent, and reliable. By implementing data quality controls within the hub, organizations can identify and rectify data errors, inconsistencies, and redundancies, thereby enhancing the overall quality of their data assets.
Enhanced data governance
Effective data governance and security are essential for organizations to comply with regulatory requirements, protect sensitive information, and mitigate data-related risks. EDHs offer a range of governance and security measures to ensure the integrity, confidentiality, and availability of data. These measures may include access controls, encryption, authentication mechanisms, audit trails, and data masking techniques.
Get your 7-step guide to building a robust data governance strategy
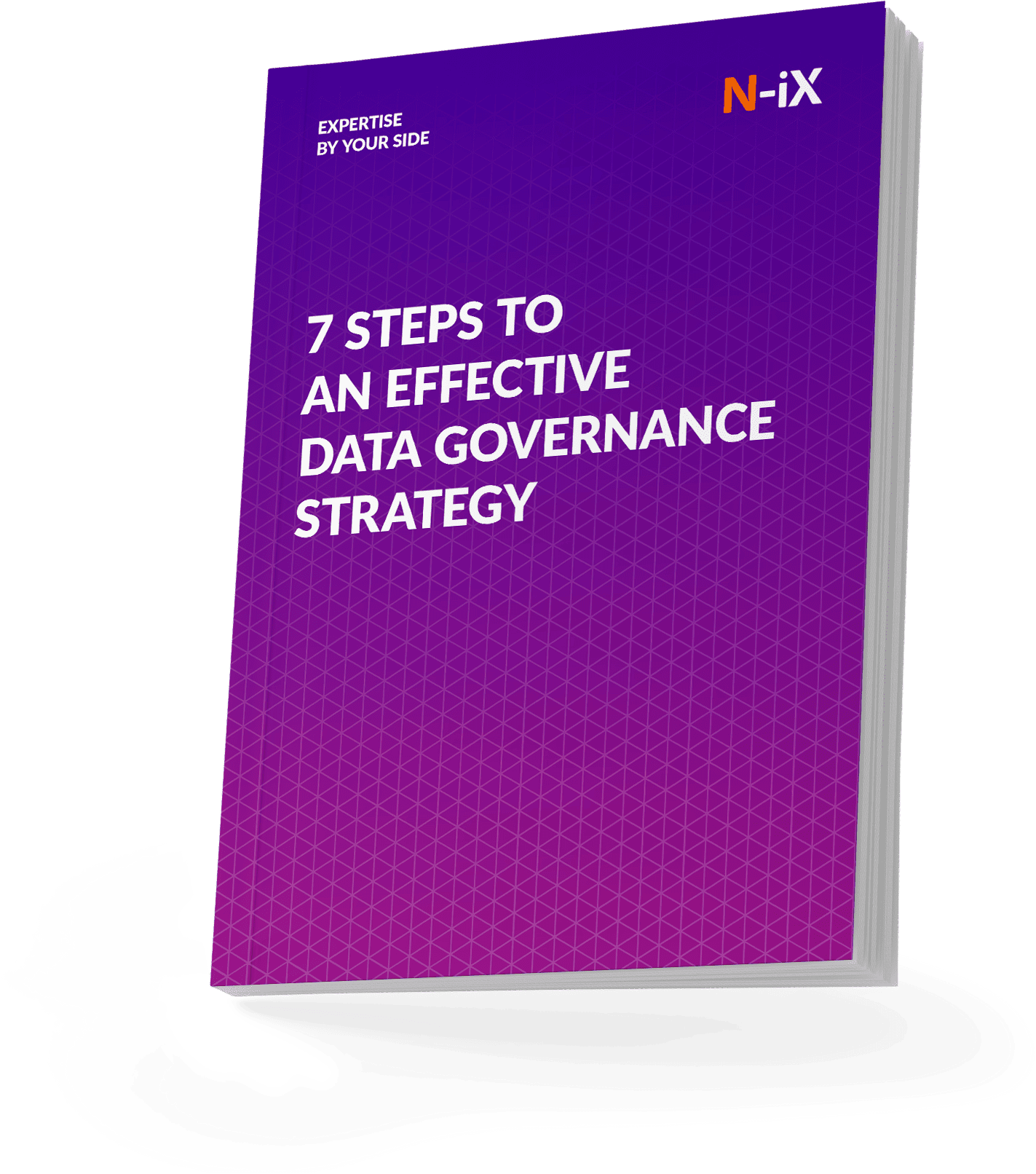
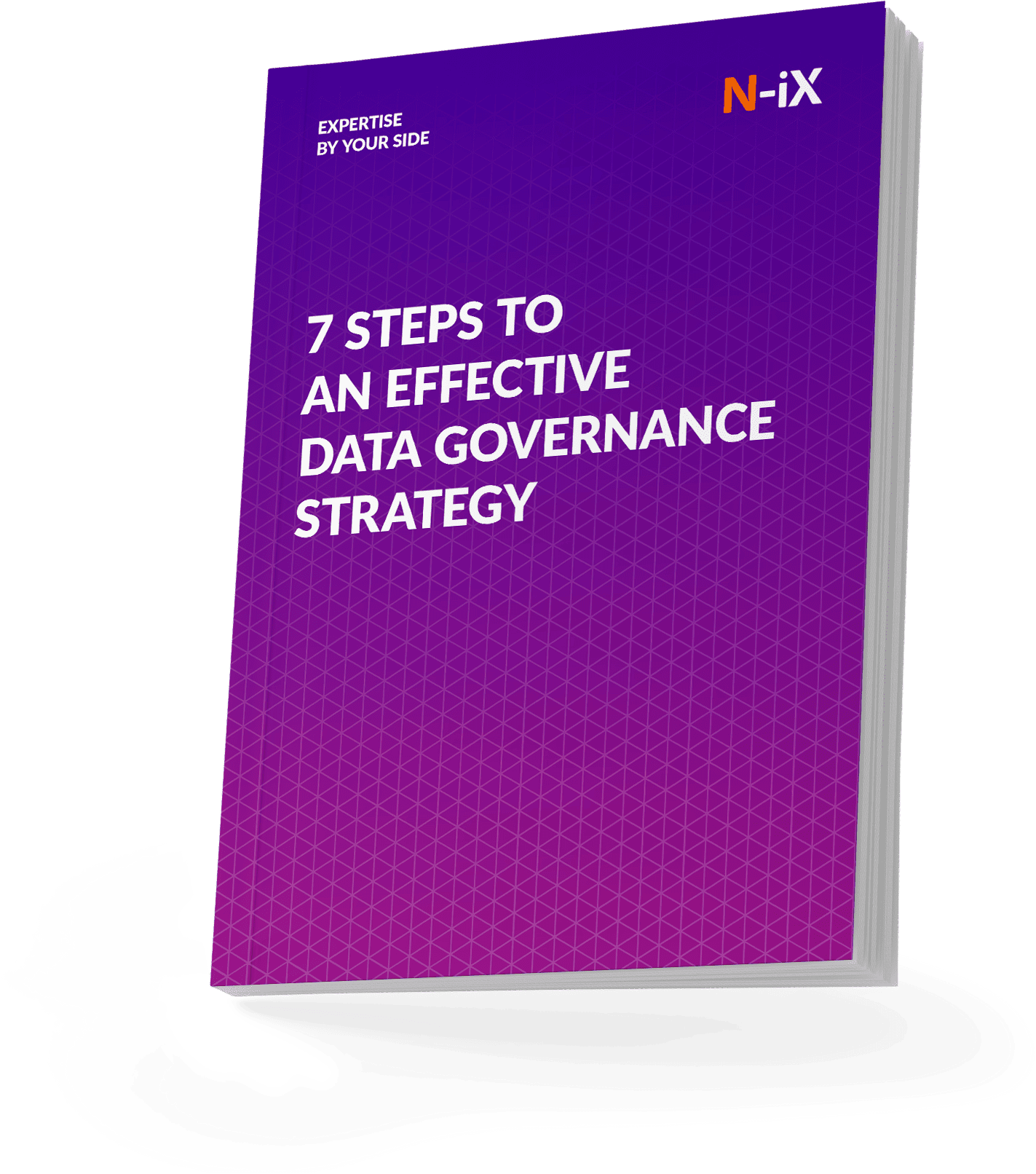
Success!
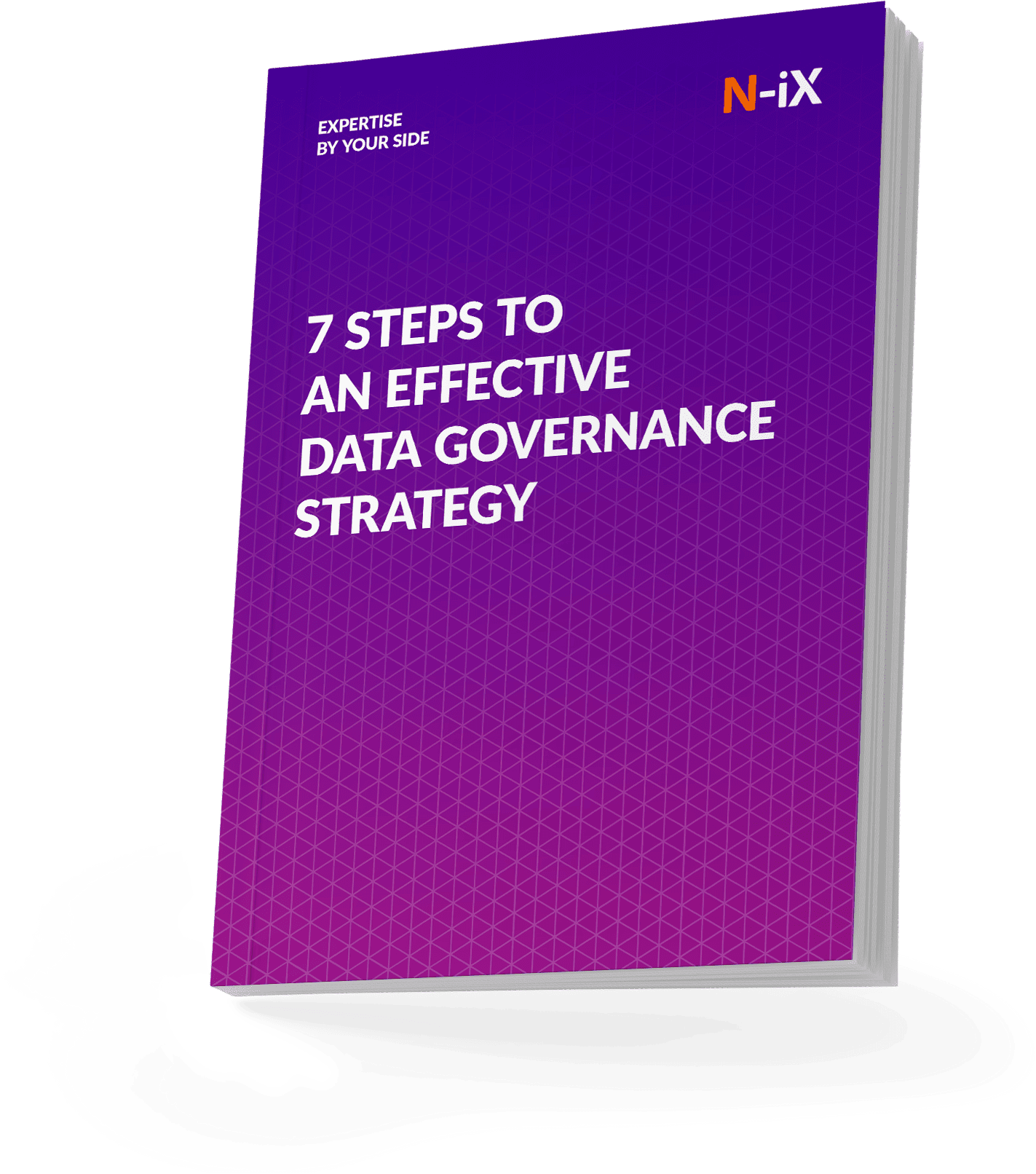
Facilitates advanced analytics
One of the primary objectives of EDH is to enable advanced analytics and insights generation. By integrating data from diverse sources and providing a unified view of organizational data, data hubs empower data scientists and analysts to perform complex analytics, predictive modeling, and machine learning algorithms. This enables organizations to uncover valuable insights, identify trends, and make data-driven decisions to drive innovation, optimize operations, and gain competitive advantage.
Enables agility in data operations
Data hubs offer agility by providing a flexible and adaptable infrastructure for changing data needs and use cases. With features such as data virtualization, schema-on-read, and self-service data provisioning, data hubs empower users to access and manipulate data quickly, experiment with new ideas, and iterate on analytics projects, thereby accelerating time-to-insight and driving business agility.
5 use cases of enterprise data hub
Let’s explore the applications of implementing data hubs across various industries.
Customer analytics and personalization
With an EDH, organizations can perform advanced analytics to segment customers based on various criteria, such as purchase history, browsing behavior, demographics, and geographic location. These insights can then be leveraged to personalize marketing messages, offers, and product recommendations.
For example, an ecommerce company can use an EDH to analyze customer purchase history and browsing behavior to identify trends and patterns. Based on this analysis, the company can personalize product recommendations and promotional offers for individual customers, increasing sales and customer satisfaction.
Operational efficiency
Organizations can analyze operational data in real time to identify bottlenecks, inefficiencies, and areas for optimization. For example, a manufacturing company can use an EDH to monitor production processes, identify equipment failures or downtime, and optimize production schedules to minimize costs and maximize efficiency.
Furthermore, an EDH can enable predictive analytics and machine learning algorithms to forecast demand, optimize inventory levels, and streamline logistics operations.
Fraud detection
Fraud detection is a critical use case of EDH implementation, especially in industries such as banking, insurance, and finance. Companies can leverage advanced analytics and machine learning algorithms to detect anomalies, patterns, and trends indicative of fraudulent behavior.
Furthermore, an EDH can enable organizations to perform comprehensive risk assessments by analyzing data from internal and external sources, such as economic indicators, regulatory compliance data, and market trends.
Supply chain optimization
Data hubs offer valuable capabilities for supply chain optimization by centralizing and analyzing data from various supply chain systems, including inventory management, logistics, procurement, and supplier relationships. It includes:
- perform advanced analytics to optimize inventory levels;
- streamline procurement processes;
- enhance logistics operations;
- track and monitor the movement of goods across the supply chain in real time.
Predictive maintenance
Predictive maintenance in industries such as manufacturing, energy, and utilities, where the efficient operation of assets is essential for business success. With an EDH, organizations can analyze historical and real-time data to identify patterns, anomalies, and trends indicative of equipment failures or maintenance needs. Organizations can schedule maintenance activities proactively, minimize unplanned downtime, and optimize asset utilization by predicting equipment failures before they occur.
Final thoughts
EDH offers a myriad of benefits, ranging from enhancing performance and reducing costs on existing systems to empowering users with self-service analytical capabilities and facilitating the development of data-driven applications. However, navigating the complexities of EDH implementation requires expertise and experience. At N-iX, we specialize in designing and implementing robust data solutions tailored to meet each client's unique needs.
- With over 200 data and AI specialists, N-iX has completed more than 60 data science, data analytics, cloud solutions, and AI/ML implementations;
- Our capabilities span various facets of data services, including data analysis, statistical analysis, predictive analytics, causal analysis, data visualization, reporting, and data mining;
- Having 22 years of experience, we have a proven track record of success with industry-leading enterprises, including Lebara, Gogo, Cleverbridge, and numerous Fortune 500 companies;
- N-iX partners with leading technology providers like AWS, Microsoft, Google Cloud, and Oracle;
- We have been recognized as a rising star in data engineering by Information Services Group (ISG);
- N-iX prioritizes data security, ensuring compliance with international standards such as GDPR, HIPAA, ISO 27001:2013, ISO 9001:2015, and PCI DSS.
Have a question?
Speak to an expert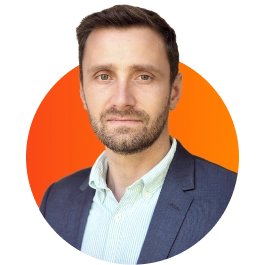