Deep learning in the manufacturing industry is a cutting-edge technology that is promised to revolutionize production processes. By leveraging the power of Artificial Intelligence (AI) and Machine Learning (ML), deep learning can help manufacturers optimize their products, automate quality control tasks, predict maintenance needs ahead of time, and more.
In this article, we'll discuss deep learning, explore its potential benefits for manufacturing operations and look at examples of how it's utilized. We'll also cover some common challenges associated with implementing deep learning solutions in the industrial setting. This way, you’ll have all the information needed to make an informed decision about incorporating these technologies into your business strategy.
What is deep learning in the manufacturing industry?
Deep learning is a subset of Machine Learning that utilizes neural networks with multiple layers to learn from and process large amounts of data. It is widely used in computer vision, natural language processing, speech recognition, etc.
By applying advanced image and signal recognition techniques to manufacturing, deep learning can detect and classify product defects early and, subsequently, improve final product quality. Using historical data, deep learning models detect objects or anomalies in images or videos captured by production line cameras. Manufacturers can identify defects quickly and accurately without any manual inspection.
In addition, deep learning models can predict when equipment is likely to fail and schedule maintenance and repairs in advance, reducing downtime and extending equipment life. Sensor readings can be analyzed over time to predict when components need service. It also enables the optimization of production processes and the scheduling of resources to improve efficiency.
Moreover, deep learning requires less feature engineering than traditional Machine Learning techniques. This method is more accurate than others at detecting complex patterns in large datasets. Additionally, deep neural networks generalize well. When a model is trained on one dataset, it can easily be applied to another similar dataset without additional training.
However, deep learning can pose some challenges. For neural networks to learn effectively, they need to be trained with enough labeled data. They must also ensure accuracy with noisy input signals and manage the computational costs associated with running complex neural networks on GPUs. If AI solutions are deployed into production environments, there may also be legal considerations that depend on the country's laws.
Read more: Machine Learning vs Deep Learning: Which one to choose?
Benefits of using deep learning for manufacturing processes
Deep learning is a powerful tool for manufacturers that offers improved efficiency and accuracy. By leveraging the power of deep learning algorithms, manufacturers can gain better insights into their production process and optimize it to reduce costs while increasing customer satisfaction.
- Improved efficiency. One of the main benefits of deep learning in manufacturing is its potential to drive significant improvements in efficiency and productivity. The ability to analyze vast amounts of data and detect patterns that would be difficult or impossible for humans to discern is among the crucial use cases of deep learning. Thus, these models can help to optimize production processes, reduce downtime, and improve the overall performance of manufacturing systems. It allows manufacturers to achieve greater output with fewer resources, thereby increasing their competitiveness in the market.
- Quality improvement. Another key advantage of deep learning in manufacturing is its ability to improve the quality of products. Through image and signal recognition, an industrial deep learning model can be trained to detect and classify defects early in the production process. This process enables manufacturers to take corrective action and prevent defects from reaching the customer. It improves product quality, increases customer satisfaction, and reduces the need for warranty claims and returns.
- Cost reduction. A third benefit of deep learning for manufacturing is its ability to decrease expenses. By predicting when equipment is likely to fail and scheduling maintenance and repairs in advance, manufacturers can reduce the cost of downtime and prolong the life of their equipment. Additionally, by optimizing production processes and detecting defects early, manufacturers can reduce the amount of scrap material produced and increase the yield of high-quality products, leading to significant cost savings.
Deep learning applications in manufacturing
Deep Learning has a range of applications in the manufacturing industry. Let’s briefly consider a few examples, such as quality control, predictive maintenance, and product optimization. These are specific deep learning applications in manufacturing that can bring a competitive edge.
Quality control
Deep learning-based image recognition and object detection algorithms can be used to inspect and classify products for defects. For example, a deep learning model can be trained on a dataset of images of products with and without defects to detect inaccuracies in new images of products. Once a defect is detected, the model can be trained to classify it into different types, such as scratches, dents, misalignment, etc. It can help to reduce the amount of scrap material produced and improve overall product quality. Once the model is deployed, it can be used to analyze products' images at different production stages, such as after assembly, painting, packaging, and shipping. In addition to visual inspection, deep learning can also be applied to other types of signals, such as audio or vibration, to detect defects that are not visible to the human eye.
Predictive maintenance
Deep learning can also predict equipment failures. Deep learning models identify patterns that indicate when equipment is likely to fail by analyzing sensor data from machinery. It allows manufacturers to schedule maintenance and repairs in advance, avoiding costly downtime and prolonging the life of the equipment. Advanced applications can be based on combining multiple sensor data types like temperature, vibrations, pressure, or velocity. The model can predict the remaining useful life of the equipment and proactively schedule maintenance based on that.
Product optimization
For instance, an industrial deep learning model can be trained on a dataset of images of products and associated quality scores and then used to predict the quality of new product images. By identifying low-quality products early in the production process, manufacturers can take corrective action to improve the quality of the final product. Additionally, deep learning can be used to optimize the scheduling of resources, such as machines and personnel. For example, a model can be trained on historical data of production processes to predict the optimal scheduling of machines and personnel to minimize downtime and maximize productivity.
Deep learning can be used to optimize manufacturing processes, from predicting defects and optimizing supply chains to automating visual inspection. Despite its potential, companies should address a few challenges before deep learning can be successfully implemented in the manufacturing industry.
Read more: The what, why, and how of machine learning and deep learning
Challenges faced when implementing deep learning in manufacturing
Some of the most potent challenges of deep learning for manufacturing are data collection, model training, and cost considerations. Let’s dive deeper into each of these challenges to understand how to tackle them.
- Data collection and storage. One of the biggest challenges in deep learning implementation is collecting enough data to train the model. Manufacturing processes can produce vast amounts of data that must be collected, stored, and organized to create an effective training dataset. It requires significant resources and expertise in data management. This information must also be kept secure from unauthorized access or manipulation.
- Model training and deployment. Once the necessary datasets have been gathered, they can be used to train a deep learning model that will be able to predict outcomes based on input parameters accurately. This process requires specialized knowledge of Machine Learning algorithms and computing power for training large models with complex architectures. Furthermore, after training, these models must then be deployed into production environments where they interact with real-world systems to make predictions about future events or behaviors.
- Cost considerations. Deep learning projects require significant investments, both upfront (data collection) and ongoing (model maintenance). Companies should carefully consider their budget when deciding whether or not to pursue such projects, as they may quickly become cost-prohibitive if not managed properly over time.
Companies should seek out experienced partners specializing in deep learning solutions for manufacturing processes to overcome these challenges. For instance, partnering with N-iX allows companies to benefit from the expertise while minimizing costs associated with implementing such projects internally. The N-iX Big Data team has 10+ years of expertise in data-related services with a robust team of 200+ specialists. The company’s specialists have a solid background in Big Data and AI/ML, allowing them to deliver from-scratch solutions to manufacturing industry innovators.
Deep learning in action: Redflex
Redflex is a leading supplier of digital traffic enforcement systems, such as automatic number plate recognition (ANPR) and average speed. The client, looking to expand its presence in the UK and Europe, needed to develop a next-generation solution for traffic management that adhered to the rules of different countries. Utilizing cameras placed on specific road segments, the client wanted to detect cars, speed, color, and size, and identify related anomalies.
In collaboration with the client's team from Australia, N-iX focused on several tasks: person detection to capture distracted driving behaviors and seat belt fastness/violation classification. Using computer vision and deep learning, N-iX created a proof of concept (PoC) system with 88% accuracy for detecting seat belt usage. This solution supports the client's strategy for making the roads safer and smarter.
Value delivered:
- Releasing the PoC system with 88% accuracy for detecting seat belt usage;
- Working on the PoC to capture distracted driving behaviors, with person detection accuracy at over 91% for night and day images.
Read more: Increasing market reach with traffic management and computer vision
Wrap-up
Deep learning has become an increasingly popular technology in the manufacturing industry, offering a range of benefits to businesses. By leveraging deep learning algorithms and models, manufacturers can automate processes, reduce costs, improve product quality and increase efficiency. Businesses can utilize deep learning capabilities to improve quality control, predictive maintenance, and product optimization. While there are challenges, these obstacles can be overcome with the right approach and a trusted vendor by your side.
Why choose N-iX for a deep-learning-powered manufacturing project?
- N-iX is one of the leading Big Data vendors, with 10+ years of proven expertise in data-related services;
- N-iX has a team of 200+ data specialists with expertise in Big Data, AI/ML, legacy migration, Data Science, and Data Analytics, among the few;
- We offer technology consulting and implementation of intelligent solutions powered by AI and ML, such as deep learning, predictive analytics, customer behavior analysis, risk allocation and mitigation, and predictive maintenance;
- Among N-iX clients in Big Data services are Orbus Software, Gogo, Lebara, AVL, cleverbridge, and Fortune 500 companies;
- N-iX has been repeatedly recognized by CRN among the leading solutions providers in North America, as proven by Solution Provider 500 and CRN Fast Growth 150 ratings;
- The company complies with industry regulations and standards, including PCI DSS, ISO 9001, ISO 27001, and GDPR.
Have a question?
Speak to an expert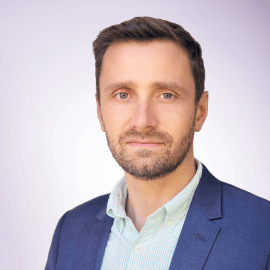