The agricultural industry is confronting a novel set of challenges, including climate change, fluctuations in supply and demand, workforce lockdowns, and supply chain disruptions. At the same time, there is consensus among industry stakeholders that the current situation requires a more robust supply chain system. Farmers must utilize cutting-edge technologies to enhance resilience and reduce potential risks.
Within this context, Big Data analytics emerges as a transformative tool. Leveraging vast data can solve problems by enhancing forecasting accuracy and boosting operational efficiency. However, a few questions need to be addressed for successful implementation:
- What are the precise benefits of Big Data in agriculture? Understanding these advantages is crucial to making informed decisions about integrating this technology into daily operations.
- What are the key challenges in adopting Big Data analytics in the agricultural industry? Identifying potential roadblocks early on can help prepare strategies to overcome them, ensuring a smoother transition.
- How can these challenges be overcome? Formulating concrete strategies for dealing with these hurdles is paramount to successfully adopting and effectively utilizing Big Data analytics in the sector.
By providing clear and comprehensive answers to these questions, we can pave the way for the agriculture industry to fully benefit from the potential of Big Data analytics and transform its operations in this challenging pandemic scenario.
Key benefits of big data and data science in agriculture:
Big data and data science are progressively becoming the bedrock of modern agriculture, offering numerous advantages that help optimize productivity and efficiency.
- Enhanced yield and production forecasting: Through predictive analytics, Big Data allows for more accurate yield and production forecasts, enabling farmers to plan and manage their resources better, thus maximizing productivity.
- Expedited delivery: By analyzing logistics and supply chain data, stakeholders can facilitate faster delivery of goods to distribution centers and, subsequently, to consumers, enhancing the efficiency of the supply chain.
- Real-time decision making: Big data analytics facilitates real-time decisions and alerts, as data from fields and equipment are continuously analyzed. It allows for immediate intervention when required, minimizing potential losses.
- Predictive maintenance: Data science helps predict the likelihood of equipment failure, allowing for preventive maintenance. This proactive approach enhances the lifespan of farming equipment and reduces downtime.
- Energy efficiency: Big data helps optimize energy use. Through smart metrics and data analytics capabilities, water and electricity usage can be significantly reduced, leading to more sustainable farming practices.
- Data-driven decision-making: Decision-making based on production and business performance data allows for strategic planning and resource allocation, contributing to increased profitability.
- Optimized farming practices: Big data helps identify correlations between field conditions, weather, and commodity data, enabling more efficient irrigation, fertilization, and harvesting of crops.
- Demand prediction: By predicting the demand for seeds, fertilizers, and animal feed, Big Data enables agribusiness suppliers to prepare for demand spikes, ensuring a consistent supply.
- Innovative pricing programs: Big data analytics can devise new pricing programs that align demand with supply. This becomes particularly relevant when product demand is strongly correlated with commodity pricing.
- Food waste reduction: Approximately 20% to 30% of food is wasted today at various supply chain stages. Big data can help reduce this wastage, potentially saving between $155–405 billion annually by 2030.
- Cost savings and business opportunities: According to research from Tufts University, more innovative farming practices facilitated by Big Data could generate around $2.3 trillion in annual cost savings and business opportunities, with $250 billion of those savings attributed to AI and data analytics
- Supply chain management: Big data enhances supply chain management by allowing farmers to trace their products throughout the supply chain. In turn, this enables retailers, distributors, and other key stakeholders to offer products and services that better meet the needs of the agricultural market.
Big Data in agriculture: Best practices
Several critical factors need to be considered for agritech providers keen on harnessing the potential of Big Data.
Continuous monitoring
Farmers require technologies that facilitate constant monitoring of vital parameters that directly impact yield and profitability. Wireless sensors and variable rate technologies enable farmers to track important agricultural parameters like temperature, weather conditions, nitrate content, soil quality, plant health, and weed and pest detection.
The following are key data sources that can drive value from Big Data in agriculture:
- Traditional enterprise data: Data from operational systems can provide valuable insights into day-to-day activities and overall productivity.
- Sensor data: This includes data from farm field sensors (such as temperature, humidity, rainfall, and sunlight), farm equipment sensors (from tractors, plows, and harvesters), and sensor data from harvested goods and livestock delivery vehicles (from farms to processing facilities).
- Trade and financial data: Commodities' trade and financial forecast data can offer a broader picture of market trends.
- Weather data: Information about weather conditions can significantly influence farming decisions.
- Genomic research data: Animal and plant genomics research data can provide valuable breeding and disease management insights.
- Drone-collected data: Drones can collect real-time data on various farm parameters, offering a bird's eye view of the entire operation.
- Geospatial and satellite data: These data sources can be used for predicting crop yields and identifying potential problem areas on the farm.
Ensure data accuracy
Ensuring the accuracy of the data collected is paramount. Technologies that can accurately collect and store data (aerial mapping, field harvesting, weather conditions, chemical detection, etc.) are essential in making agritech solutions more reliable. These technologies are vital in providing farmers with accurate data for informed decision-making.
Capitalize automation
Increasing the automation of crop cultivation and livestock production systems is another vital factor. Automated tools and equipment such as robotics and drones facilitate precision agriculture and enable continuous data collection and analytics. This can lead to more efficient farming practices, reduced waste, and increased productivity.
Leveraging Big Data for agritech business: A strategic approach
1. Define clear business KPIs and estimate ROI
The first step towards making Big Data work for your agritech business case involves defining KPIs and estimating ROI. The rationale is to determine whether your Big Data solution will be feasible and profitable for your agricultural venture. The Discovery Phase will help you assess different scenarios, enabling you to select the Big Data analytics system most suited to your needs. This phase provides the necessary deliverables to kick-start the implementation phase efficiently.
2. Ensure effective Big Data engineering
The success of a Big Data analytics project in the agritech sector relies heavily on the following elements:
- Suitable data sources: Choose data sources wisely, as they form the backbone of your analytics. From weather data to farm equipment sensor data, ensure that these sources provide relevant, reliable, and comprehensive information for your business.
- Orchestrated ecosystem: Develop an orchestrated ecosystem of platforms that collects data from hundreds of disparate sources. This will help create a unified view of your operations, leading to better insights and decision-making.
- Data preprocessing: Focus on cleaning, aggregating, and preprocessing the data to make it fit for a specific business case. This step is crucial to transform raw data into valuable information that can be used for actionable insights.
- Data Science or Machine Learning models: In some instances, applying Data Science or Machine Learning models to your preprocessed data can enable predictive capabilities. These models can provide foresight, helping your business adapt to trends and changes, thereby gaining a competitive edge.
Challenges of Big Data development in agriculture
Challenge #1: Poor Data Quality
The success of Big Data in agriculture is predicated on the availability of clean, valid, and complete data. Any discrepancies in the dataset may lead to misleading results, impacting your operational decisions adversely.
Solution:
It's advisable to adhere to the following best practices to ensure high-quality data:
- Suitable data sources: Opt for reliable data sources and conduct continuous data checks to ensure their integrity.
- Data preparation: Emphasize data preparation and cleaning to make it fit for analysis.
- Automated checks: Establish automated checks for all incremental data pipelines to maintain data consistency and accuracy.
- Data governance: Implement and master data management solutions to enhance data quality.
Challenge #2: Scarcity of Big Data Skills
Accurate results in Big Data projects require appropriate data collection, structuring, and cleaning. This leads to an increasing demand for skilled Big Data developers. For instance, Germany hosts over 30,000 professional data scientists and Big Data engineers, yet top talent is often hard to secure due to the high demand.
Solution:
Many tech companies are now seeking Big Data analytics partners across the globe, including Eastern Europe. This region boasts a robust talent pool of over 150,000 data scientists and Big Data engineers, particularly in Poland and Ukraine.
Choosing Big Data experts
When outsourcing Big Data development, consider the following:
- Talent pool: Choose a location known for a broad talent pool to ensure access to skilled professionals
- Expert vendor: Opt for a Big Data development vendor with expertise in Big Data Engineering, Data Science, Machine Learning, Business Intelligence, Cloud, DevOps, and Security
- Specialized Skills: Hire Big Data developers skilled in:
- The Hadoop ecosystem and Apache Spark for large data storage and processing;
- Cloud-based tools such as Snowflake, EMR, Dataprots, Cloud Composer, BigQuery, Synapse Analytics, DataFactory, DataBricks;
- SQL/NoSQL databases and Big Data tools such as RedShift, Hive, and Athena for querying data;
- Maintenance of MapReduce Java code and the ability to rewrite it using more recent Spark technology.
- Proficient in languages like Scala, Python, and Java.
- Kubernetes constructs for building Big Data CI/CD pipelines.
- Real-time Big Data streaming tools like Kafka, AWS Kinesis, or Apache Pulsar.
Why choose N-iX for Big Data in agriculture:
- N-iX is a proven leader in Big Data solutions, boasting a team of more than 200 specialists with an extensive track record in delivering complex projects;
- The company’s Big Data specialists are proficient in programming languages, including Python, Scala, Java, C/C++, and SQL. They also demonstrate expertise in Apache Spark, Flink, Beam frameworks, and other Big Data tools such as Hadoop, Pig, and Hive;
- We offer technology consulting and the implementation of intelligent AI and ML-powered solutions such as predictive analytics, customer behavior analysis, risk allocation and mitigation, and predictive maintenance.
Have a question?
Speak to an expert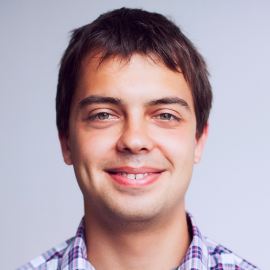