Businesses face difficulties in accurately predicting demand due to changing market trends and evolving consumer preferences. The problem is further complicated by the need to optimize stock management, improve prediction accuracy for promotional campaigns, and implement innovative strategies to minimize waste, both physical and digital. Accurate demand forecasting and planning facilitate organizations to allocate resources effectively, resulting in cost savings and increased revenue opportunities.
The solution is advanced demand planning forecasts powered by Artificial Intelligence services. Let's explore how AI demand forecasting can change your business operations, enhance customer experience, and drive sustainable growth.
How AI demand forecasting differs from traditional forecasting methods
Traditional forecasting methods often cannot incorporate contextual factors such as market trends, customer behavior, or external influences, leading to less accurate forecasts. These methods assume that historical patterns will continue unchanged in the future. They may encounter difficulty handling large volumes of data or complex patterns, limiting their applicability.
In contrast, AI forecasting leverages advanced machine learning algorithms that can process vast amounts of data quickly and identify intricate patterns and trends. AI models swiftly incorporate sudden changes, such as unexpected product surges or disruptions in operations, into updated forecasts.
A typical demand forecasting solution utilizes various data types to predict future demand accurately. These data include:
- Historical sales data;
- Seasonal trends;
- Economic conditions;
- Competitor activity;
- Real-time data, such as website traffic and social media engagement.
Competitive edge of AI in demand forecasting
Let's explore how AI enables more accurate predictions, efficient resource allocation, and proactive decision-making.
Improved demand forecasting and planning
Businesses make informed predictions about future demand patterns, drawing on historical data, market trends, and other factors. Using advanced algorithms, businesses can identify patterns and correlations within the data to generate precise forecasts. Also, this enables businesses to grasp the impact of promotions on demand, facilitating more strategic planning and execution of marketing campaigns. As a result, the likelihood of overstocking or stockouts is minimized, leading to fewer returns and cancellations.
AI forecasting provides businesses with several key benefits:
- Providing timely and reliable product availability;
- Minimizing instances of stockouts or overstocking;
- Enhancing responsiveness to changing customer demands;
- Anticipating and catering to seasonal peaks or trends effectively.
Optimized stocks
Demand forecasting plays a crucial role in optimizing inventory management for businesses. It enables accurate demand prediction, ensuring the right amount of stock is available at the right time and place. This capability helps businesses avoid the pitfalls of overstocking, which ties up capital and incurs storage costs, as well as understocking, which leads to lost sales and unhappy customers.
Reduced waste
AI demand forecasting significantly reduces business waste by aligning production and inventory levels with actual demand. This alignment helps prevent overproduction and excess inventory, thereby minimizing the amount of unsold or expired goods contributing to waste. Not only does this strategy save businesses from incurring disposal costs, but it also contributes significantly to sustainability initiatives by reducing their environmental footprint.
Increased revenue and enhanced profit margins
Enterprises can drive revenue growth and profit margin enhancement by providing precise predictions of customer demand. This accuracy allows businesses to optimize their inventory levels.
Reducing stockouts entails minimizing instances where products are unavailable when customers want to purchase them. Consequently, with products readily available when customers seek them, businesses can seize sales opportunities and boost revenue streams.
Use cases of AI demand forecasting
AI for demand forecasting use cases exemplify its versatile applications across various industries. Keep reading to discover versatile applications across healthcare, retail, finance, supply chain, and manufacturing industries.
Retail
Retailers rely on AI solutions, whose multifaceted applications span from demand forecasting and inventory optimization to personalized recommendations and dynamic pricing strategies:
- Demand forecasting. AI algorithms analyze historical data and external factors to predict demand, reducing stockouts and excess inventory accurately.
- Inventory optimization. Integrating AI-driven forecasts with inventory systems minimizes carrying costs and ensures optimal stock levels.
- Market trends monitoring. AI monitors economic indicators, weather patterns, and social trends to adjust predictions accordingly.
- Dynamic pricing optimization. AI adjusts prices based on demand fluctuations and market variables, maximizing revenue and responsiveness.
Supply chain
AI impacts supply chain management through its diverse applications, optimizing various aspects from demand forecasting to transportation planning. According to Gartner, demand forecasting is the most widely used AI application in supply chain planning, with 45% of companies currently using it and 43% planning to adopt AI-powered demand forecasting within two years.
- Demand forecasting. Logistics companies utilize AI-driven demand forecasting models to optimize transportation networks, customs clearance processes and shipping routes, enabling efficient route optimization and resource allocation for timely deliveries.
- Production planning. Electronics manufacturers rely on AI tools to analyze historical order data and supplier performance, facilitating collaboration and streamlining production planning.
- Reverse logistics. AI-powered analytics models predict future returns, optimizing reverse logistics processes and managing returned inventory more effectively.
Manufacturing
Manufacturers leverage AI across key operational areas to streamline processes and adapt to changing customer needs.
- Waste reduction. AI-driven forecasting minimizes waste by aligning production levels with actual demand, optimizing resource utilization
- Seasonal demand fluctuations. AI models analyze historical sales data across seasons to forecast seasonal demand fluctuations accurately, allowing manufacturers to optimize production schedules and staffing resources efficiently.
- Dynamic pricing strategies. AI integrates market conditions, competitor pricing, and historical sales data to recommend optimal pricing strategies, maximizing revenue by aligning prices with current market dynamics and customer demand.
- Product demand forecasting. AI leverages data on customized product orders and customer preferences to forecast demand for specific configurations.
Finance
Finance and banking institutions use AI across critical functions to optimize operations and meet the evolving needs of customers:
- Market trend forecasting. AI predicts market trends and fluctuations to inform investment decisions and risk management strategies.
- Credit risk assessment. AI assesses borrower data to forecast credit risk and inform lending decisions, helping financial institutions assess and manage various types of risk.
- ATM and branch optimization. It analyzes transaction data and foot traffic patterns to enhance operational efficiency at ATMs and branches, enabling proactive resource allocation for staffing and cash availability.
Healthcare
In the healthcare sector, demand forecasting can transform patient care and resource management.
- Predicting patient admission rates. AI algorithms employ historical patient data and disease prevalence to forecast future admission rates accurately.
- Resource allocation. Leveraging AI-driven demand forecasts, healthcare providers optimize the allocation of resources such as hospital beds and medical equipment.
- Medical supply inventory. Demand forecasting enables healthcare organizations to predict demand for medical supplies accurately.
- Medication demand forecasting. Analyzing prescription data and vaccination trends to anticipate demand for medications and vaccines, optimizing inventory and distribution.
Implementing an AI for demand forecasting
Implementation involves several key steps and considerations to ensure its effectiveness and successful integration into existing systems:
Ensuring data quality
High-quality data ensures that the foundation upon which forecasting models are built remains solid and dependable.
Businesses draw upon a diverse array of data sources to glean insights into consumer behavior, market trends, and external influences. Historical sales data offers invaluable glimpses into past demand patterns and seasonal fluctuations. Market trends provide context for evolving consumer preferences and competitor activities. External factors, ranging from economic indicators to weather patterns, offer additional insight, enabling businesses to anticipate and adapt to changing demand dynamics.
Employ various preprocessing techniques to refine and enhance their datasets
- Normalization ensures that numerical features are scaled uniformly, preventing biases and discrepancies in the model;
- Feature engineering involves crafting or transforming new features to extract maximum predictive value from the data;
- Data cleaning involves identifying and rectifying errors, inconsistencies, and missing values that could compromise the integrity of the forecasting model;
- Outlier detection and removal help ensure the model remains robust and resilient against anomalies.
N-iX leverages state-of-the-art data preprocessing and management techniques to ensure your AI in demand forecasting models are built on high-quality, reliable data. This approach enhances model accuracy and robustness, ensuring our clients can make confident, data-driven decisions.
Choosing an AI model
An AI model is a mathematical representation of the relationships between input variables and the target variable, which in this case is future demand. These models encompass various techniques, including machine learning algorithms such as regression, neural networks, and ensemble methods, each offering unique strengths in capturing complex patterns within the data.
Once trained, the AI model can predict new, unseen data, providing valuable insights into future demand trends. However, the performance of the model is not static and requires ongoing monitoring and optimization to maintain its accuracy. Businesses must regularly evaluate the model's performance against real-world outcomes, identify areas for improvement, and fine-tune the model accordingly.
Remember: AI modeling is the selection of features or input variables that best capture the factors influencing demand. This process involves careful consideration of various data sources, including historical sales data, market trends, and external factors such as economic indicators and weather patterns.
Leveraging our two decades of expertise in AI and machine learning, N-iX designs and deploys scalable AI models tailored to your unique business needs, ensuring that your demand forecasting is not only accurate but also adaptable to market changes.
Scaling efficiently
A scalable AI forecasting system can accommodate fluctuations in data volume, processing requirements, and user demand without sacrificing performance or reliability. This encompasses the agility to incorporate new data sources, refine forecasting models, and adjust strategies in response to changing market dynamics or organizational priorities.
Even the most advanced AI algorithms will only be able to deliver accurate predictions if they are fed with poor-quality data or lack contextual understanding of the business environment. Therefore, organizations must prioritize data governance, quality management, and domain knowledge acquisition as integral components of their AI initiatives.
N-iX excels in creating scalable AI solutions that grow with your business. Our approach ensures that your demand forecasting capabilities can easily expand and adapt, maintaining high performance regardless of data volume or operational scale.
Managing interpretability
Achieving interpretability involves several strategies and techniques. One approach is to use interpretable models, such as linear regression or decision trees, which provide clear and easily understandable explanations for their predictions. Another approach is to employ model-agnostic techniques, such as feature importance analysis or partial dependence plots, which can be applied to any machine learning model to explain its behavior.
Furthermore, organizations can enhance interpretability and explainability by providing context-specific explanations tailored to the needs of different stakeholders. This may involve visualizations, natural language explanations, or interactive dashboards that enable users to explore and understand the factors influencing the model's predictions in real time.
With N-iX, you gain not just up-to-date AI solutions but also a transparent, understandable system. We emphasize interpretability in our AI models, enabling your teams to make informed decisions based on clear, actionable insights from our advanced forecasting systems.
Why talk to N-iX experts about AI demand forecasting implementation
- N-iX has a strong track record with over 30 completed AI projects in various verticals and over 200 data, AI, and ML experts on board.
- Our services range from AI and Machine Learning, Data Analytics, Cloud Solutions, Product Discovery, Software QA & Testing to Embedded Software & IoT.
- N-iX has been listed among the world’s top 100 outsourcing service providers and consultants by IAOP, and we are CRN’s 2022 Solution Provider 500 leader.
Have a question?
Speak to an expert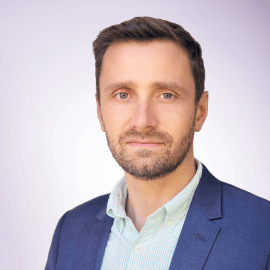