Choosing between data mesh and data fabric architectures is like selecting the backbone for your organization's data strategy. These aren't just industry jargon; they are frameworks that can significantly impact how you collect, manage, and derive insights from data.
Whether scaling a startup or an established enterprise, the right architecture within the broader context of data warehouse consulting can be the linchpin of your data operations. Should you consider using the data fabric architecture instead of a traditional, more decentralized data mesh? What is the more specific difference between the two? How do you make an informed architectural decision that aligns with your business objectives? Let’s unpack both paradigms' unique features, advantages, and challenges.
What is a data mesh?
Data mesh is an innovative approach to data architecture and platform thinking that aims to solve the challenges associated with managing large-scale, complex data in a distributed manner. It addresses the limitations of traditional centralized data architectures by promoting decentralization and domain-oriented ownership.
- Decentralization: Unlike traditional data lakes or warehouses that centralize data management, data mesh advocates for a decentralized approach. In this setup, data is managed and owned by cross-functional, domain-oriented teams within the organization. This alleviates the bottlenecks often encountered in centralized systems.
- Domain-oriented ownership: One of the cornerstone principles of data mesh is that each business domain, such as sales, marketing, or engineering, owns its data and treats it as a product. These teams are responsible for their data's quality, governance, and discoverability. It contrasts traditional methods where a centralized data team would assume these responsibilities.
- Self-serve data infrastructure: Data mesh encourages a self-serve data infrastructure where teams can easily access and consume data from other domains. This promotes collaboration and makes it easier for data to be utilized across different parts of the organization.
- Scalability: The architecture is designed to be inherently scalable. Since each domain is responsible for its data, scaling becomes a more linear and manageable task. Domains can scale their data independently, making it easier for organizations to handle growing data volumes efficiently.
- Governance: Given that data is treated as a product, there is a heightened focus on ensuring data quality, governance, and compliance. Metadata and data cataloging are vital in this setup, ensuring data is easily discoverable and usable.
By adopting data mesh, organizations aim to create a more flexible, scalable, and responsive data architecture that can adapt to rapidly changing business needs and growing data volumes. It offers a way to break down data silos, improve data quality, and make data a shared asset across an enterprise.
Read more: Data mesh: all you need to know about distributed data delivery
How is data fabric different?
Data fabric is an architectural concept designed to manage, integrate, and utilize data across an organization. It provides a unified, integrated layer that enables seamless data access and sharing across disparate systems, formats, and storage solutions.
- Integration: One of the primary goals of data fabric is to integrate data from various sources, whether cloud-based storage, on-premises databases, or third-party APIs. The architecture creates a cohesive data environment, facilitating better business intelligence and analytics.
- Centralized management: Unlike data mesh's decentralized model, data fabric often involves centralized governance and metadata management. This ensures uniform data quality, governance, and security across the organization.
- Data access and movement: Data fabric simplifies data access and movement across the organization. It provides capabilities like data transformation, real-time streaming, and batch processing to ensure data is available where and when needed.
- Technology agnostic: One of the strengths of a data fabric architecture is its technology-agnostic nature. It is generally designed to work with multiple data storage solutions, data formats, and processing engines, making it easier for organizations to adopt new technologies and tools.
- Integrations: In the data mesh vs data fabric debate, data mesh emphasizes domain-oriented data ownership. In turn, data fabric focuses more on the technological underpinnings. It often uses a mix of databases, data lakes, and other storage solutions to create a unified data infrastructure.
Data fabric is the backbone for various data activities within an enterprise, including analytics, Data Science, and real-time data operations. By providing a unified data environment, Data fabric allows for more efficient data operations and enables organizations to glean more value from their data assets. It is particularly beneficial for businesses with various data types and sources that need a unified, secure, and well-governed approach to manage them all.
Explore more about: Data fabric vs data lake: What is the difference?
Data mesh vs data fabric: Main differences
Both data mesh and fabric architectures serve as frameworks for a seamless, integrated data experience across complicated, distributed systems. However, their approaches differ in several fundamental ways.
- Firstly, data mesh adopts a product-centric view of data as its core design principle. This approach ensures that data is managed, maintained, and provisioned like any other product within the organization. On the flip side, data fabric utilizes automation for tasks like discovering, connecting, and delivering data assets, all based on a comprehensive metadata foundation, often represented by a knowledge graph.
- Secondly, the governance models in the two architectures are different. In a data mesh, domain or product owners are responsible for establishing initial requirements for data products, ensuring a more hands-on, domain-specific approach to data management. Data fabric leans more on automation and metadata to cater to data consumer needs, with less emphasis on human-led governance.
- Thirdly, the organizational requirements also vary significantly. Unlike data fabric, data mesh requires technological changes and demands organizational structure and culture shift. It places the responsibility of data product delivery onto domain teams, potentially adding to their existing workload. Additionally, it promotes the decentralization of data roles, such as data scientists and engineers, embedding them within domain or product teams.
In summary, while both data mesh and data fabric offer robust architectures for data management, they do so with differing philosophies and requirements. If you navigate the intricate decision between these two frameworks, a seasoned data service provider like N-iX can be invaluable. With extensive experience in data warehouse consulting, N-iX can guide you through the complexities of adopting either architecture, ensuring that your choice meets technical specifications and aligns with your broader business goals.
Data mesh vs data fabric: Key adoption questions
Making your mind in the data mesh vs data fabric architecture debate requires a thorough evaluation of your organization's specific needs, goals, and existing infrastructure. Here are the key questions to consider:
Organizational structure and culture
- Is your organization more centralized or decentralized?
A centralized organization might find it easier to adopt a data fabric due to its inherent centralized governance. On the other hand, data mesh thrives in decentralized settings where cross-functional teams can independently manage and own their data.
- How mature is your organization in terms of data culture?
Data mesh requires a strong data culture where teams are data-savvy and can take on the responsibility of treating data as a product. If your organization is still building its data capabilities, the centralized expertise in a data fabric model might be more suitable.
Data volume and complexity
- What is the scale and complexity of your data?
Data mesh is often ideal for continuously growing large datasets, as its architecture is designed for horizontal scalability. Data fabric can handle large data sets, too, but may require more centralized resources to manage growing complexity.
- How diverse are your data sources and types?
Suppose you have data from various systems, IoT devices, third-party APIs, and other sources. In that case, the data fabric’s robust integration capabilities can create a unified environment for disparate data types.
Scalability and growth
- How quickly is your data landscape growing?
Data mesh allows each domain to scale its data independently, making the architecture inherently scalable. It is advantageous if different departments within your organization are growing at different rates.
Governance and compliance
- What are your data governance and compliance needs?
If you require strict data governance and compliance measures, centralized control in a data fabric can be beneficial. In a data mesh, these responsibilities are distributed, requiring a more robust, organization-wide commitment to governance.
Download your 7-step guide to building a robust data governance strategy
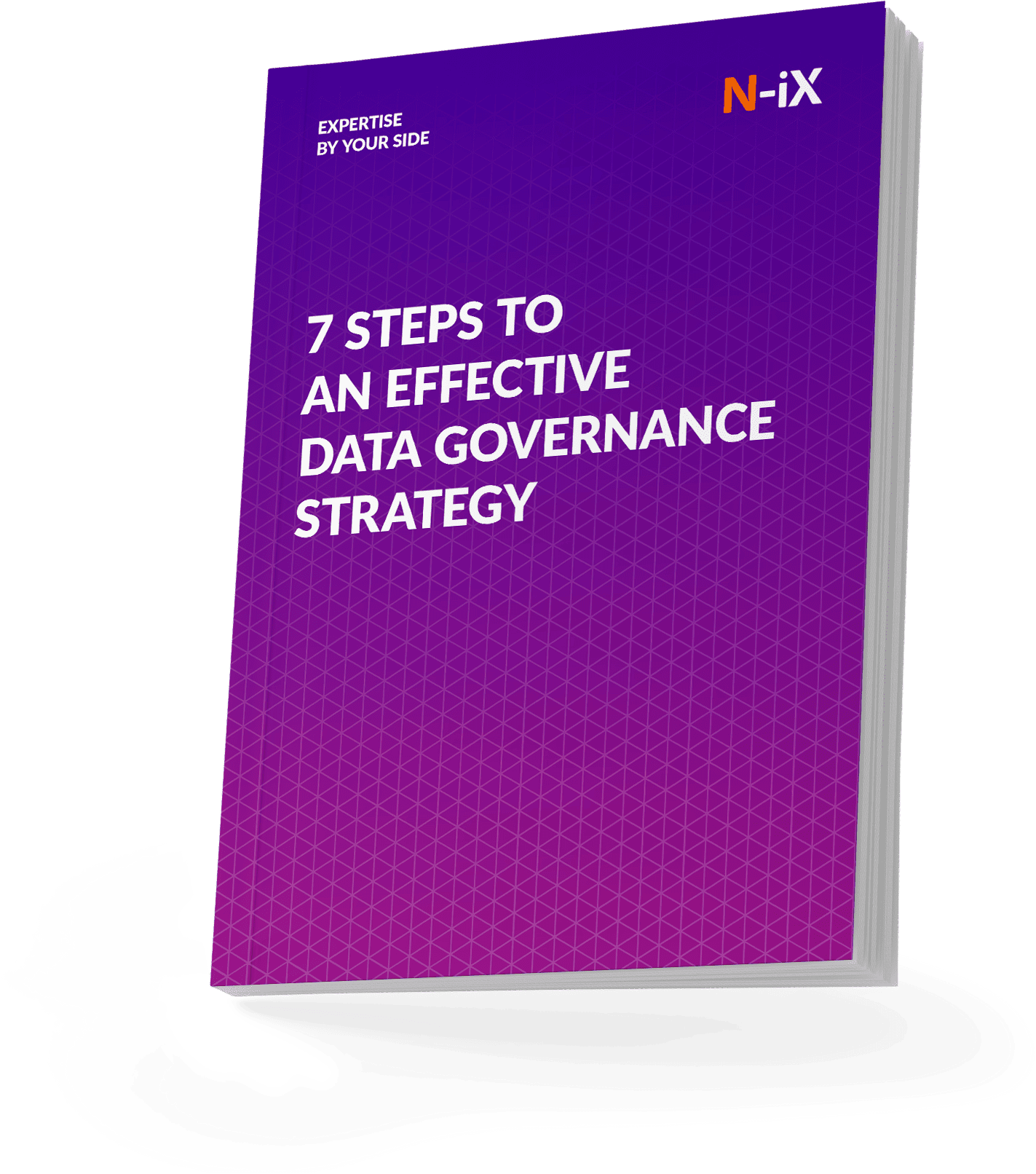
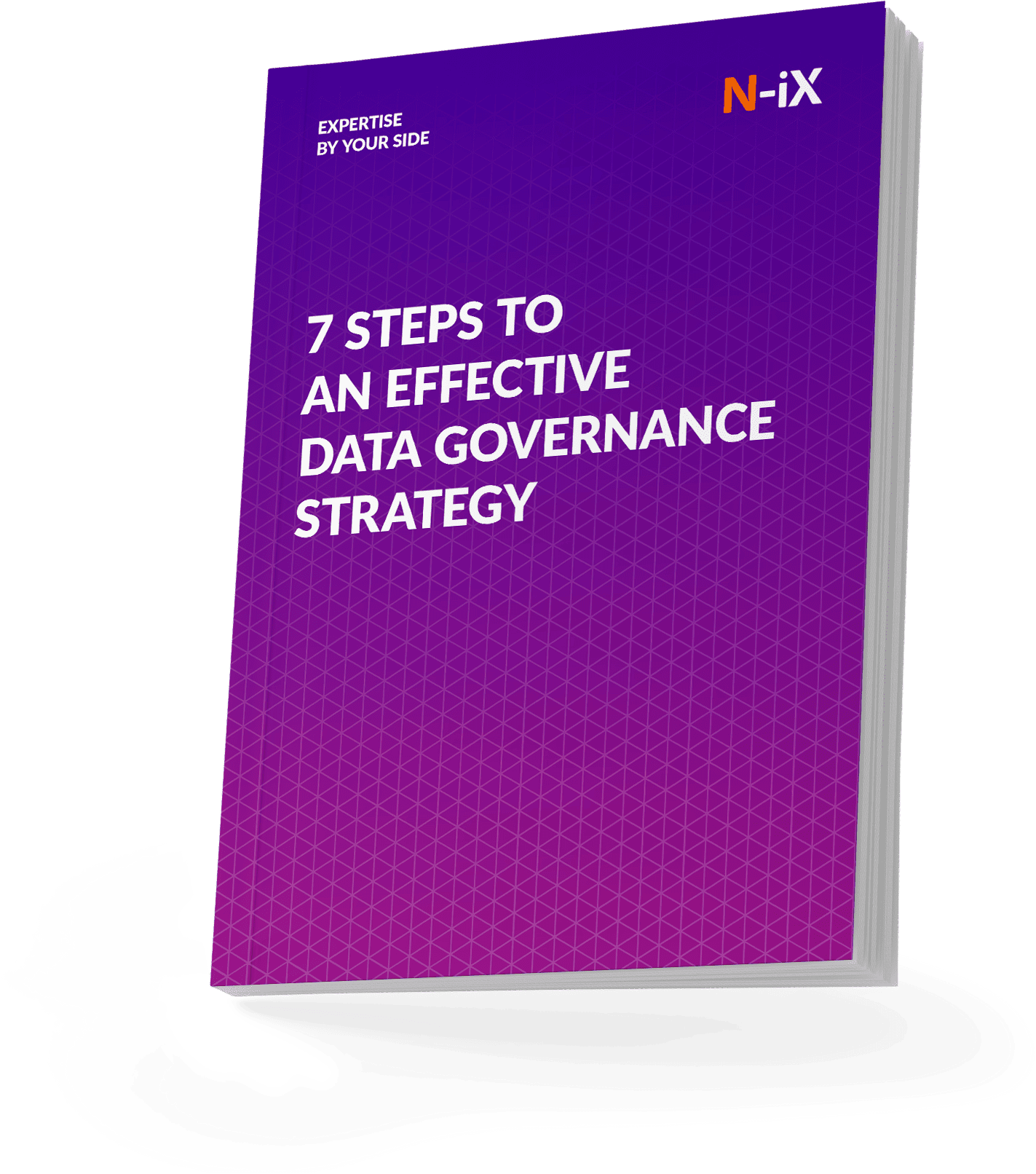
Success!
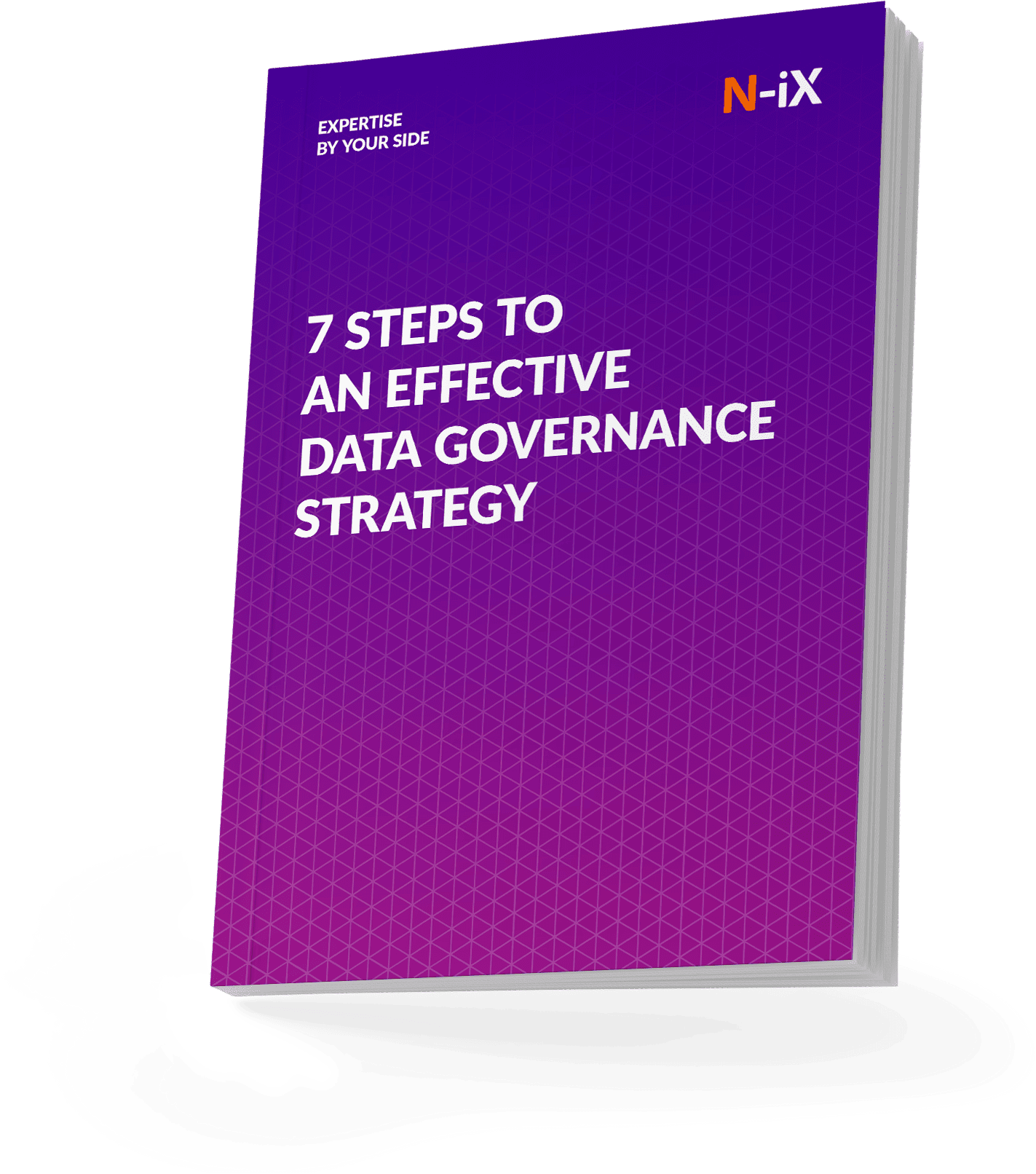
Technology stack
- What does your existing technology stack look like?
Data fabric is generally agnostic to the technologies you use, making it easier to integrate into your existing tech stack. Data mesh, being domain-centric, might require you to consider whether each domain's tech stack can support data product management.
- Are you looking for a technology-driven or a domain-driven approach?
Data fabric is often chosen for its ability to integrate multiple technologies into a unified data infrastructure. Data mesh, meanwhile, is more focused on aligning data with business domains.
Operational efficiency
- How crucial is operational efficiency for your data teams?
With data mesh, the workload of managing data is distributed across different domains, potentially reducing bottlenecks and making the overall data pipeline more efficient. Data fabric would centralize these responsibilities, creating a more streamlined and concentrated workload.
Resource availability
- Do you have the resources and expertise to manage data as a product?
Data mesh requires specialized skills in data product management within each domain team. If your organization doesn’t have these skills, you might need to invest in training or hiring, which could be costly and time-consuming.
Business objectives
- What key business objectives do you aim to achieve with the new architecture?
The choice between data mesh and data fabric should be aligned with what you aim to achieve, be it better data governance, more efficient analytics, or breaking down data silos.
Flexibility and future-proofing
- How vital is your architecture to be flexible and adaptable to future changes?
In the data mesh vs data fabric discussion, it’s worth noting that both architectures offer flexibility but in different ways. With its decentralized nature, data mesh can quickly adapt to changes or additions in business domains. Data fabric, being technology-agnostic, offers the flexibility to swap out or add new technologies as needed.
Each question helps you evaluate the most fitting architecture based on multiple facets of your organization, from culture and technology to governance and business goals. Moreover, it can also be helpful to involve stakeholders from various departments in this evaluation process to get a comprehensive view of your architecture decision.
Final remarks
Overall, the data mesh vs data fabric debate is crucial for forming a conclusive mind on the architectural frameworks for data management. Data mesh thrives in decentralized environments with a strong data culture, while data fabric excels in centralized governance and seamless integration. Choosing between these two should align with your organizational needs and long-term data objectives.
If you're navigating this complex decision, consider leveraging the expertise of N-iX. With a strong portfolio in Data Analytics, Cloud, and AI, we offer tailored solutions to help you effectively implement a data mesh or data fabric architecture. With N-iX as your partner, you can ensure a data strategy that meets technical requirements and aligns with your business goals.
Why partner with us?
- N-iX has over 22 years of experience establishing robust partnerships with leading global enterprises, such as Gogo and Lebara, and a range of Fortune 500 companies;
- Our dedicated Data Unit with over 200 skilled experts is ready to tackle the most complex challenges.
- At N-iX, our team of over 60 DevOps engineers has successfully executed more than 50 projects, ranging in complexity and scale.
- As an Advanced Amazon Partner, Microsoft Gold Partner, and Google Platform Partner, N-iX demonstrates a strong competency in cloud-native services, underscoring our expertise in this crucial tech domain.
Have a question?
Speak to an expert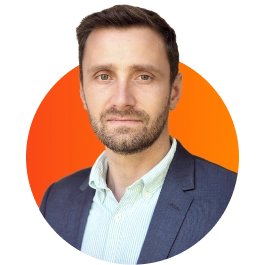