The banking industry is undergoing a significant transformation, driven by the rapid growth of digital technologies, regulatory changes, and increasing customer expectations. According to WestMonroe, banks must lean into automation, AI, and data analytics to build a digital operating environment. In the age of digital disruption, one key component that has emerged as a game-changer for banks is data analytics. This powerful tool enables financial institutions to gain actionable insights from their vast data reserves, driving informed decision-making and maintaining a competitive edge.
This article will explore the concept of Big Data analytics in banking, discussing its benefits, challenges, and specific use cases. Additionally, we will delve into the differences between data analytics in banking and other industries. Our goal is to provide a comprehensive guide for prospective leads seeking to understand and harness the potential of data analytics in the financial sector.
Understanding Big Data analytics in banking
Data analytics is the process of examining, cleaning, transforming, and modeling raw data to extract valuable insights, draw conclusions, and support informed decision-making. In banking, data analytics involves systematically using data and quantitative analysis techniques to understand customer behavior, identify trends, mitigate risks, and uncover opportunities for growth.
Banks and fixancial institutions generate and process vast amounts of data daily, from customer transactions and account details to market trends and economic indicators. Data analytics plays a crucial role in transforming this raw data into actionable insights, enabling banks to:
- Enhance customer service and develop personalized offerings;
- Identify and manage risks, such as credit risk and fraud;
- Streamline operations and optimize resources;
- Adhere to regulatory requirements and monitor compliance;
- Drive strategic decision-making and maintain a competitive edge.
Read more: Big Data for financial services: benefits, challenges, and use cases
While data analytics is used across various industries, the banking sector presents unique challenges and opportunities. Some key differences include:
- Regulatory environment: Financial institutions face stricter regulations and compliance requirements than other industries. Data analytics solutions in banking must be designed to ensure adherence to these guidelines, such as Open Banking.
- Data sensitivity: The data handled by banks is often highly sensitive, including personal financial information. Big data analytics in banking requires a strong focus on data privacy and security to protect customer information and maintain trust.
- Complexity and variety of data: Banking data can come in numerous formats and from diverse sources, such as customer transactions, market data, and social media. Data analytics in banking and financial services demands robust data integration, preprocessing, and modeling techniques to handle this complexity.
- Risk management focus: Banking organizations must manage various risks, including credit, market, and operational threats. Data analytics in the banking sector often emphasizes risk identification, assessment, and mitigation as a core component of its applications.
- Real-time decision-making: In the fast-paced banking environment, real-time data analytics enables quick decisions, such as fraud detection and prevention or dynamic pricing.
You may also be interested in: How to mitigate your supply chain risks with Data Analytics
Data analytics use cases in banking
The banking industry is a prolific generator and collector of data on a daily basis. However, the question of how financial institutions can derive actionable insights from this data to make better decisions and improve their operations often remains unanswered. This is where the use of data analytics in banking proves to be invaluable. By utilizing advanced analytical tools and techniques, banks are able to extract valuable insights into customer behavior, market trends, and internal processes. Let’s explore some of the most common use cases of data analytics in the banking industry and examine how these applications are driving innovation, enhancing efficiency, and improving overall customer experiences.
Fraud detection and prevention
Fraud is a significant concern in the banking sector, and data analytics can play a critical role in detecting and preventing fraudulent activities. Advanced Machine Learning algorithms can analyze vast amounts of transaction data in real-time, identifying patterns and anomalies indicative of fraud. Banks can then use these insights to flag suspicious transactions, prevent unauthorized access, and reduce reputational and financial losses related to fraud.
Credit risk assessment
Thorough credit risk assessment is vital for maintaining a healthy loan portfolio and minimizing defaults. Data analytics can help banks develop sophisticated credit scoring models by incorporating a broader range of data, such as credit history, income, spending patterns, and social media activity. This comprehensive approach can lead to more accurate risk assessments and better-informed lending decisions.
Customer segmentation and personalized marketing
Data analytics allow banks to segment their customers based on various factors, such as demographics, spending habits, and financial goals. This segmentation enables banks to develop targeted marketing campaigns and personalized product offerings, ultimately driving customer engagement and loyalty. By leveraging data analytics, banks can ensure that their marketing efforts are more effective and generate a higher return on investment.
Predictive maintenance for ATMs and other equipment
Banks rely on various equipment, such as ATMs, servers, and security systems, to provide essential services to their customers. Data analytics can be used to monitor the performance of this equipment and predict maintenance requirements, reducing downtime and optimizing performance. By proactively addressing maintenance issues, banks can improve customer experience and reduce the costs associated with equipment failures.
Optimizing branch performance and resource allocation
Data analytics can help banks analyze the performance of their branches, identifying areas for improvement and opportunities for growth. Banks can make data-driven decisions regarding branch staffing, resource allocation, and expansion plans by reviewing factors such as foot traffic, transaction volumes, and customer demographics. This optimization can lead to more efficient operations and improved profitability.
Benefits of data analytics in banking and financial services
Data analytics helps banks to understand their customers' needs, preferences, and behavior patterns. Banks can develop personalized products and improve customer service by analyzing transactional data and other touchpoints, ultimately enhancing the overall customer experience. Some of the most remarkable advantages of leveraging Big Data analytics in banking are:
Improved risk management
If we speak about the banking industry, effective risk management is essential. Data analytics helps banks identify, assess, and mitigate various risks, such as credit, market, and fraud. By utilizing advanced algorithms and predictive models, banks can gain insights into potential threats and take proactive measures to minimize their impact.
Streamlined operations and cost savings
Data analytics can drive efficiency by automating manual processes, identifying bottlenecks, and optimizing resource allocation. For example, banks can use analytics to predict ATM maintenance requirements, optimize branch operations, or improve the effectiveness of their call centers. These improvements can lead to significant cost savings and increased operational efficiency.
Data-driven decision-making
Banks must make well-informed, strategic decisions to stay competitive in a rapidly evolving industry. Data analytics provides actionable insights that help financial institutions make data-driven choices related to product development and investment strategies or risk management. This data-driven approach can lead to better business outcomes and a sustainable competitive advantage.
Compliance and regulatory monitoring
The banking sector faces stringent regulatory requirements, and non-compliance can result in fines and reputational damage. Data analytics helps banks monitor compliance with regulations, identify potential issues, and take corrective actions. Additionally, analytics can assist in meeting reporting requirements and streamlining the audit process.
Read more: How to improve your business with efficient financial data management
Challenges of implementing Big Data analytics in the banking sector
While the potential benefits of Big Data analytics in the banking industry are significant, implementing these technologies presents its own set of challenges. From the complexities of data integration to data security and privacy, banks face various obstacles when adopting these advanced analytics techniques. Let’s review these challenges individually and find out how partnering with a data analytics vendor can solve such hurdles of successful adoption and integration.
Banks handle vast amounts of sensitive customer data, which makes data privacy and security paramount. Implementing data analytics in banking requires stringent measures to protect against data breaches, unauthorized access, and misuse. Banks must adhere to various regulations, such as GDPR, Open Banking, and CCPA while ensuring customer data's secure storage and processing.
The banking sector generates diverse and complex data from multiple sources, such as transactional data, customer profiles, and market data. Integrating and consolidating this data for analysis can be challenging. Ensuring data quality is also essential, as inaccurate or incomplete data can lead to misleading insights and poor decision-making in the long run. Banks can streamline the consolidation process and improve overall data quality by implementing robust data integration and validation tools.
Banks operate in a highly regulated environment and must comply with various rules and guidelines. Implementing data analytics solutions in banking involves ensuring that the solutions adhere to these regulations, including data privacy, risk management, and reporting requirements. Navigating the complex regulatory landscape can be challenging and time-consuming. Utilizing compliance automation tools and working closely with regulatory experts can help banks efficiently navigate the regulatory environment and ensure compliance.
Data analytics in banking requires specialized skills, such as data science, machine learning, and programming expertise. There is a high demand for these skills across various industries, leading to a talent shortage and competition for qualified professionals. Banks must invest in training and development to bridge the skills gap or consider partnering with external service providers. By establishing strong relationships with credible technology vendors, banks can tap into a pool of skilled professionals and foster long-term talent development.
Introducing data analytics in a traditional banking environment may face resistance from employees who are accustomed to existing processes and systems. Change management plays a crucial role in overcoming this resistance, ensuring that employees understand the benefits of data analytics and are equipped with the necessary skills to embrace new technologies. Banks can solve this challenge by providing comprehensive training programs and ongoing support.
Criteria for selecting the right data analytics partner
Partnering with a global technology provider can be an effective solution for overcoming the challenges of implementing Big Data analytics in the banking industry. A well-rounded provider can deliver a custom-tailored data analytics solution specifically designed to meet the unique needs of a bank's operations, customer base, and overall business objectives. By leveraging the expertise and experience of an outsourcing vendor, banks are able to streamline the implementation process and ensure a smooth integration of their new analytics capabilities.
Selecting the right outsourcing partner for your data analytics needs is crucial for achieving success in the banking sector. Some key criteria to consider when evaluating potential partners include:
- Domain expertise: Look for a partner with a strong background in data analytics and a deep understanding of the banking industry, including its unique challenges and regulatory landscape;
- Proven track record: Seek a partner with a history of successful data analytics implementations in the banking sector, as evidenced by case studies and client testimonials;
- Technological capabilities: Ensure that the partner has the necessary technical expertise in areas such as data integration, Artificial Intelligence and Machine Learning, and data visualization;
- Security and compliance: Verify that the partner follows stringent data privacy and security measures and adheres to the relevant regulations and industry standards;
- Scalability and flexibility: Choose a partner with a significant development team of at least 1,000 professionals. This factor is crucial for a vendor to scale their services to meet your evolving needs and is flexible in their approach to accommodate your specific requirements. On top of that, a vendor with a larger number of specialists on board can ensure a quicker project development launch.
Why choose N-iX for your Data analytics project?
N-iX is a leading software development company with 21+ years of experience and 2,200 + professionals operating in 25 countries. The company has extensive experience in delivering data analytics solutions for global clients, including Fortune 500 companies. Our experts are well-versed in the unique challenges and opportunities presented by the financial sector, ensuring that we can develop tailored data analytics solutions to meet the specific needs in the banking industry and your enterprise in particular.
The key advantages of partnering with N-iX include:
- N-iX has multidimensional experience in designing and developing strategic plans regarding data analytics in banking, with 200+ experts on board;
- N-iX engineers are experienced in the latest Big Data technologies and trends, capable of developing AI-enabled data solutions, building predictive modeling systems, and implementing custom Machine Learning algorithms;
- The company's data unit is capable of providing comprehensive data analytics services, including designing and implementing data analytics strategies, data governance, legacy migration, cloud re-platforming, building and streamlining data and analytics platforms, and Data Science services, among others.
- The company adheres to industry-specific international regulations and standards, such as GDPR, ISO, and others. On top of that, N-IX has the Hellios Financial Services Qualification System (FSQS) certification, showcasing its reputation as a trusted and secure vendor for financial institutions.
Have a question?
Speak to an expert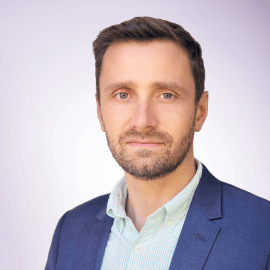