Supply chains worldwide are getting increasingly complex and volatile. According to Deloitte, 79% of companies with high-performing supply chains achieve significant revenue growth. However, managing these supply chains effectively is a growing challenge. Businesses are grappling with issues like demand fluctuations, logistical inefficiencies, and unpredictable disruptions, leading to increased operational costs and missed market opportunities.
The core of the problem lies in the traditional methods of supply chain management, which often need help to adapt to the rapid pace of change in the global market. These methods typically rely on historical data and linear forecasting models, which can be ineffective in predicting future trends and responding to real-time issues. As a result, companies need reliable generative AI solutions to avoid difficulties in inventory optimization, demand planning, and maintaining a resilient supply chain.
Let's explore how companies can address these challenges with generative AI applications for the supply chain industry.
Why adopt generative AI in supply chain?
Traditional AI in supply chains has been invaluable for parsing data, making decisions, and automating tasks based on established rules or patterns. In contrast, generative AI breaks new ground by not only analyzing and interpreting data but also creating content. It utilizes advanced technologies like deep learning, generative adversarial networks, and transformers, crafting solutions that are not pre-programmed.
Traditional AI engages statistical models and data analysis methods, including time series analysis, regression models, and machine learning algorithms, to identify trends and correlations in historical data. However, it can be limited by its reliance on large datasets of historical data and may not effectively adapt to sudden market shifts or account for new variables outside the historical scope.
Generative AI, in contrast, operates fundamentally differently. It focuses on creating new data that resembles the input data it was trained on. Utilizing advanced algorithms such as generative adversarial networks or Variational Autoencoders (VAEs), it comprehends and replicates the intrinsic patterns of the data to produce new, synthetic data points.
Additionally, the demand for generative AI in the supply chain proliferates as companies seek to optimize operations. With the global generative AI in the supply chain market size expected to rise at a CAGR of 45.62% during the forecast period from 2023 to 2032, the adoption of generative AI offers significant opportunities for supply chain innovation and efficiency.
With a clear understanding of the capabilities of generative AI, let's now shift our focus to the challenges encountered within the supply chain industry and explore how generative AI can offer transformative solutions.
Challenges of supply chain industry
The supply chain is a complex domain fraught with challenges that can impede operational efficiency and business success. Here’s a detailed overview of common challenges and pain points:
- Demand volatility: Fluctuating consumer demand makes it difficult to predict and plan for inventory needs, often leading to excess stock or shortages.
- Supply chain visibility: Lack of transparency across the supply chain hinders the ability to track and manage inventory effectively, resulting in inefficiencies and potential disruptions.
- Operational costs: Rising costs in logistics, labor, and materials put pressure on companies to optimize operations and maintain profitability. According to Accenture, generative AI could affect 43% of all working hours within end-to-end supply chain activities, either by automating tasks (29%) or significantly enhancing the productivity of human employees (14%).
- Complexity in supplier management: Managing multiple suppliers across different regions adds complexity, making it challenging to ensure quality, reliability, and timely delivery.
- Risk management: Supply chains are susceptible to various risks, including geopolitical issues and natural disasters, causing delays, financial losses, and market fluctuations, necessitating robust risk management strategies.
- Customer expectations: The modern consumer expects fast, reliable, and transparent service, requiring supply chains to be more responsive and agile.
- Manual analysis: Traditionally, data analysis in supply chains was manual, time-intensive, and susceptible to human error, often leading to inefficiencies and inaccuracies in decision-making.
Let’s discover how generative AI in supply chain can help to mitigate these challenges.
Key use cases of generative AI in supply chain
Generative AI has diverse applications in supply chain management, revolutionizing how companies plan, execute, and optimize their supply chain operations. Here's a deep dive into some of the key applications:
Demand forecasting
Generative AI synthesizes historical sales data, market trends, consumer behavior, and external factors like economic indicators to predict future demand accurately. This dynamic forecasting enables companies to adjust their production schedules, manage inventory more effectively, and respond proactively to market changes, ensuring supply aligns with demand while minimizing waste and stockouts.
Generative AI enables the analysis of real-time data streams, allowing supply chain managers to make immediate adjustments in response to changing conditions, thereby optimizing operations continuously.
For example, Walmart employs Generative AI to tailor inventory management, accurately forecasting customer demand for different products and optimizing stock levels. The company tested a generative AI-powered negotiation bot from Pactum AI, achieving cost savings of about 3% on contracts. Interestingly, most suppliers preferred negotiating with the AI bot over human negotiators.
Inventory management
Generative AI identifies the best strategies for distribution and storage, factoring in delivery times, transport costs, and demand variability. The outcome is heightened operational efficiency and significant cost savings. The technology recommends reordering points and safety stock levels, aiding in superior warehouse management. This leads to fewer product shortages, reduced surplus inventory, and lower storage costs.
AI algorithms continuously assess sales data and demand patterns, recommending real-time adjustments to inventory levels for different products to align with market demand. Moreover, these models determine the optimal safety stock levels, considering demand variability, seasonal trends, and market dynamics to prevent stockouts of popular items. Generative AI simulates potential market scenarios, such as sudden demand spikes or supply chain disruptions, enabling companies to prepare and implement effective restocking strategies. By identifying slow-moving items that incur high holding costs, generative AI suggests actions like pricing strategies or targeted marketing to enhance product turnover. It devises efficient storage and distribution methods tailored to different product categories.
A real-life example is Amazon's use of Generative AI in its fulfillment centers showcases how the technology optimizes product placement, streamlining order processing processes, and reducing storage costs.
Procurement and supplier management
Generative AI enhances supply chain resilience by analyzing vast datasets to pinpoint optimal suppliers. It evaluates performance metrics, quality assessments, and cost factors, enabling effective supplier relationship management. Through analysis of historical interactions, contracts, and performance evaluations, generative AI identifies risks and opportunities for improvement, supporting proactive supplier management and fostering strong partnerships.
ASOS uses Generative AI to handle returns, analyzing return data to identify common causes and implement strategies to decrease return rates, thereby enhancing customer satisfaction and operational efficiency.
Logistics and distribution
Generative AI for supply chains enables greater transportation efficiency by analyzing traffic flows, weather conditions, vehicle capacities, and customer demands to optimize delivery routes, ensuring fast and cost-effective paths. For example, logistics companies can use generative AI to manage delivery truck fleets, continuously gathering data from GPS traffic updates, weather forecasts, and current locations.
AI models provide real-time monitoring and re-routing capabilities during transit to circumvent delays caused by traffic congestion, accidents, or other disruptions, enhancing on-time delivery rates.
Generative AI streamlines reverse logistics by evaluating data related to product returns, repairs, and refurbishment, optimizing the pathways for returned items, and deciding on the most efficient and effective methods for repair, recycling, or disposal. It assists in managing the inventory of refurbished goods, ensuring efficient redistribution and reducing waste.
Risk management
Generative AI in supply chain identifies and manages risks within the supply chain by analyzing historical and external data, including weather patterns, geopolitical situations, and supplier disruptions. These AI models, capable of processing extensive datasets, can detect trends and patterns related to supplier reliability and forecast potential supply chain interruptions.
By doing so, businesses can evaluate supplier dependability, anticipate disruptions, and implement risk management strategies, such as diversifying suppliers or developing contingency plans, thereby fortifying supply chain resilience.
For managing returned goods, AI evaluates aspects like transportation costs, product condition, and market demand for refurbished items. This analysis aids in deciding whether a returned product should be repaired, refurbished, recycled, or disposed of, optimizing cost-efficiency and waste reduction.
Sustainability impact
Generative AI promotes sustainable supply chain practices by optimizing transportation routes to reduce fuel consumption and emissions, improving packaging material usage to minimize waste, and advocating for eco-friendly practices. It analyzes data on traffic, vehicle capacities, delivery schedules, packaging alternatives, and resource utilization.
For instance, in logistics, generative AI optimizes delivery routes and schedules for fuel efficiency, contributing to reduced greenhouse gas emissions. In packaging, it recommends designs that use fewer materials and generate less waste, aligning with sustainability goals and generating strategies for reducing carbon footprints.
Warehouse layout optimization
Generative AI enhances warehouse layout optimization in supply chains by dynamically adjusting layouts based on real-time operational data and predictive analytics. An important advantage is its ability to adapt layouts on the fly to meet changing operational needs. Unlike traditional static layouts, which are based on historical data and assumptions, Generative AI continuously analyzes incoming data streams to identify opportunities for improvement. This dynamic approach ensures that warehouse layouts remain optimized in response to fluctuations in demand, inventory levels, and other factors.
Furthermore, Generative AI can simulate various layout configurations and scenarios to identify the most efficient arrangement. For example, it can analyze historical order data to identify frequently accessed items and strategically place them closer to packing stations or shipping docks. Businesses can improve order fulfillment speed and customer satisfaction by reducing the distance traveled by warehouse workers and minimizing picking times.
AI models can simulate various layout configurations to identify the most efficient arrangement. It considers product dimensions, order frequency, and picking paths to optimize the layout for maximum throughput and storage capacity.
Why choose N-iX for generative AI implementation
When the time comes to implement Generative AI within your supply chain operations, selecting the right technology partner can spell the difference between success and failure. N-iX is a reliable partner with a proven track record in implementing generative AI in supply chain management. Here are a few reasons why you should entrust this task to our tech experts:
- With over 21 years of experience in the tech industry, N-iX has delivered 30+ successful data science and AI projects. We have a team of 200 data, AI, and ML experts who are well-versed in various verticals.
- We cover every process step, from the discovery phase, consulting, and end-to-end development to product release and post-production support.
- N-iX has been recognized globally for its outstanding services. We have been listed among the world's top 100 outsourcing service providers and consultants by IAOP and named a 2022 Solution Provider 500 leader by CRN.
- We adhere strictly to security protocols and regulatory frameworks, including ISO 27001:2013, PCI DSS, ISO 9001:2015, and GDPR, to guarantee the protection and integrity of data.
WHITE PAPER
Get your ultimate guide on Generative AI use cases and applications!
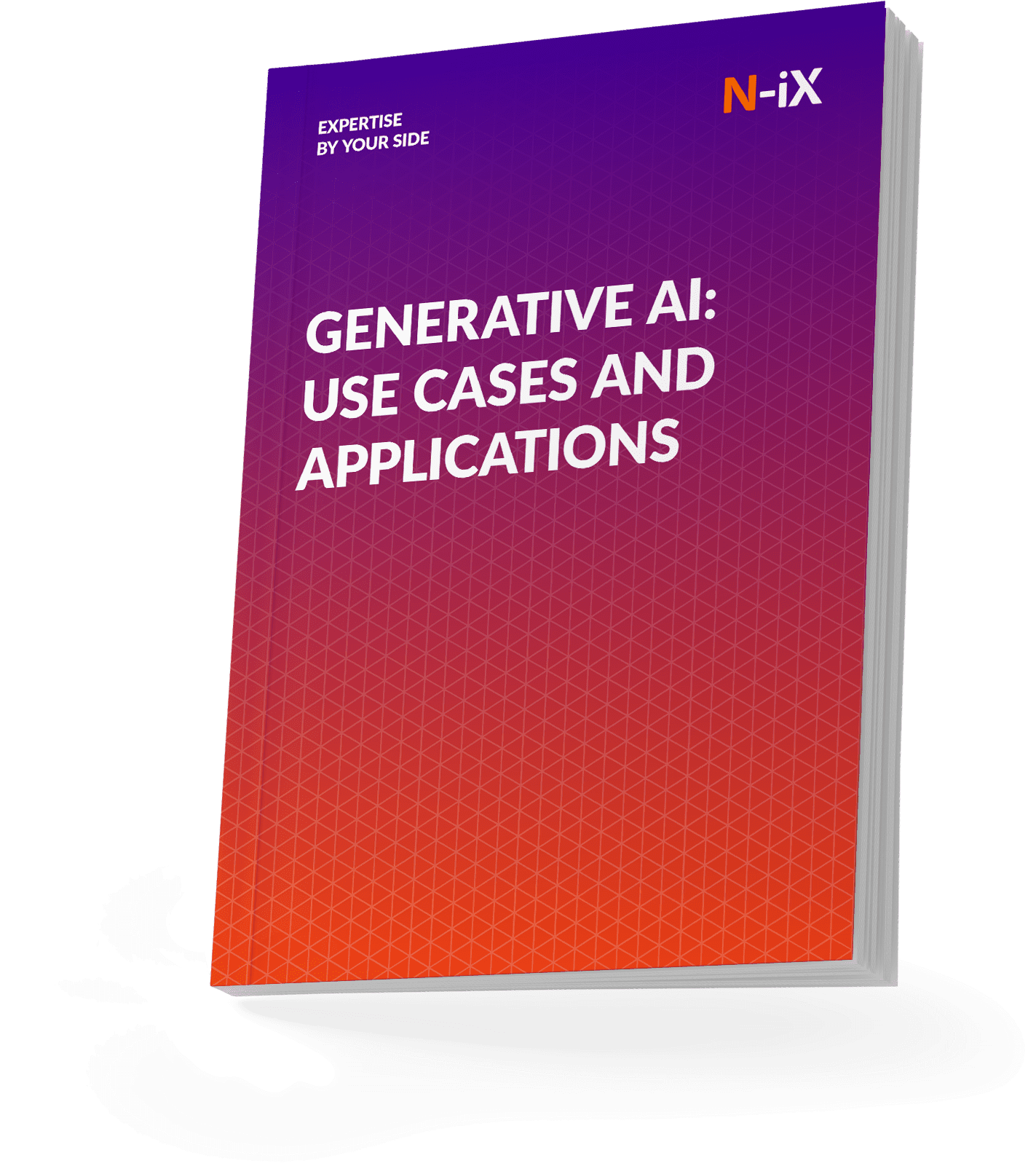
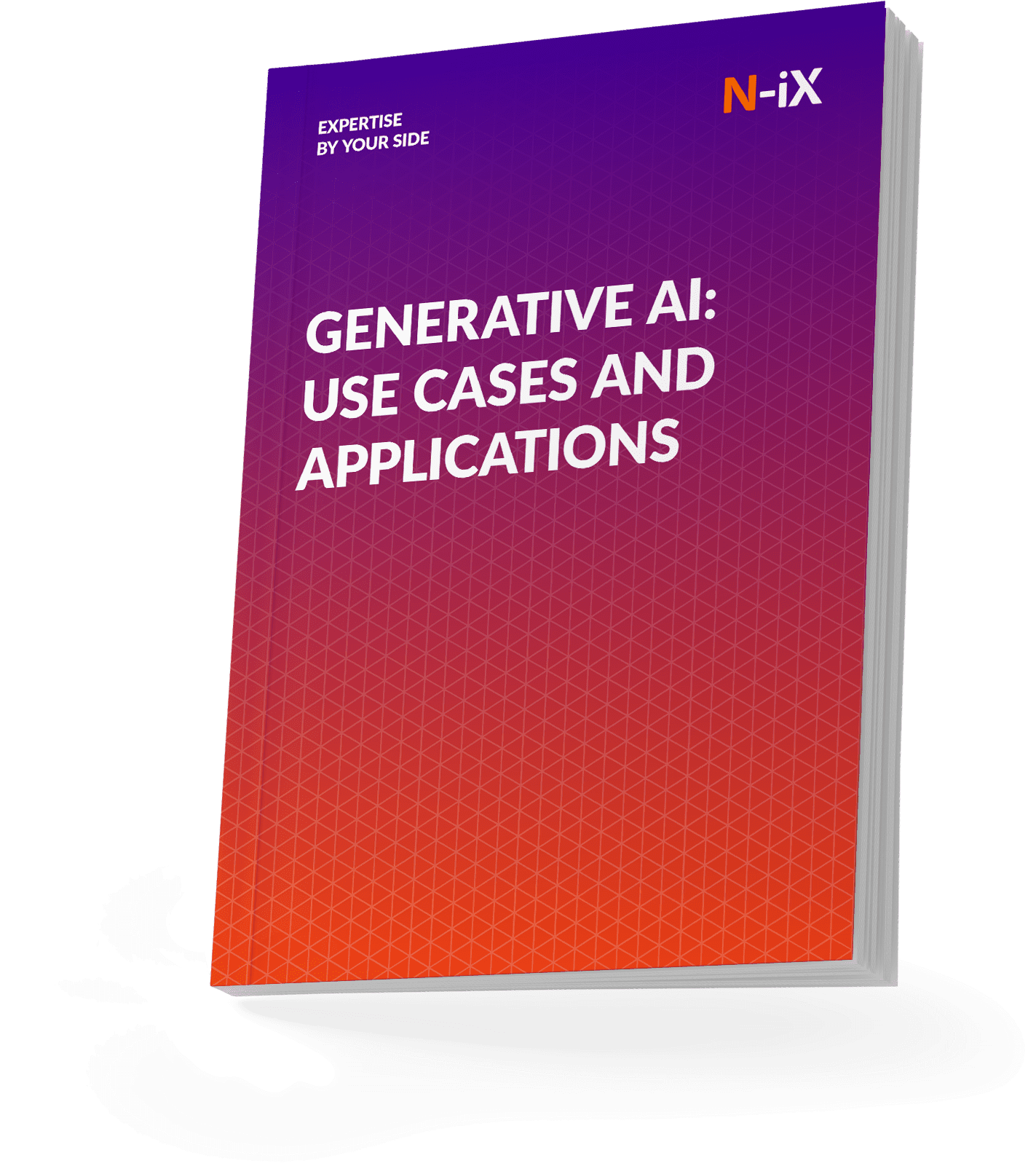
Success!
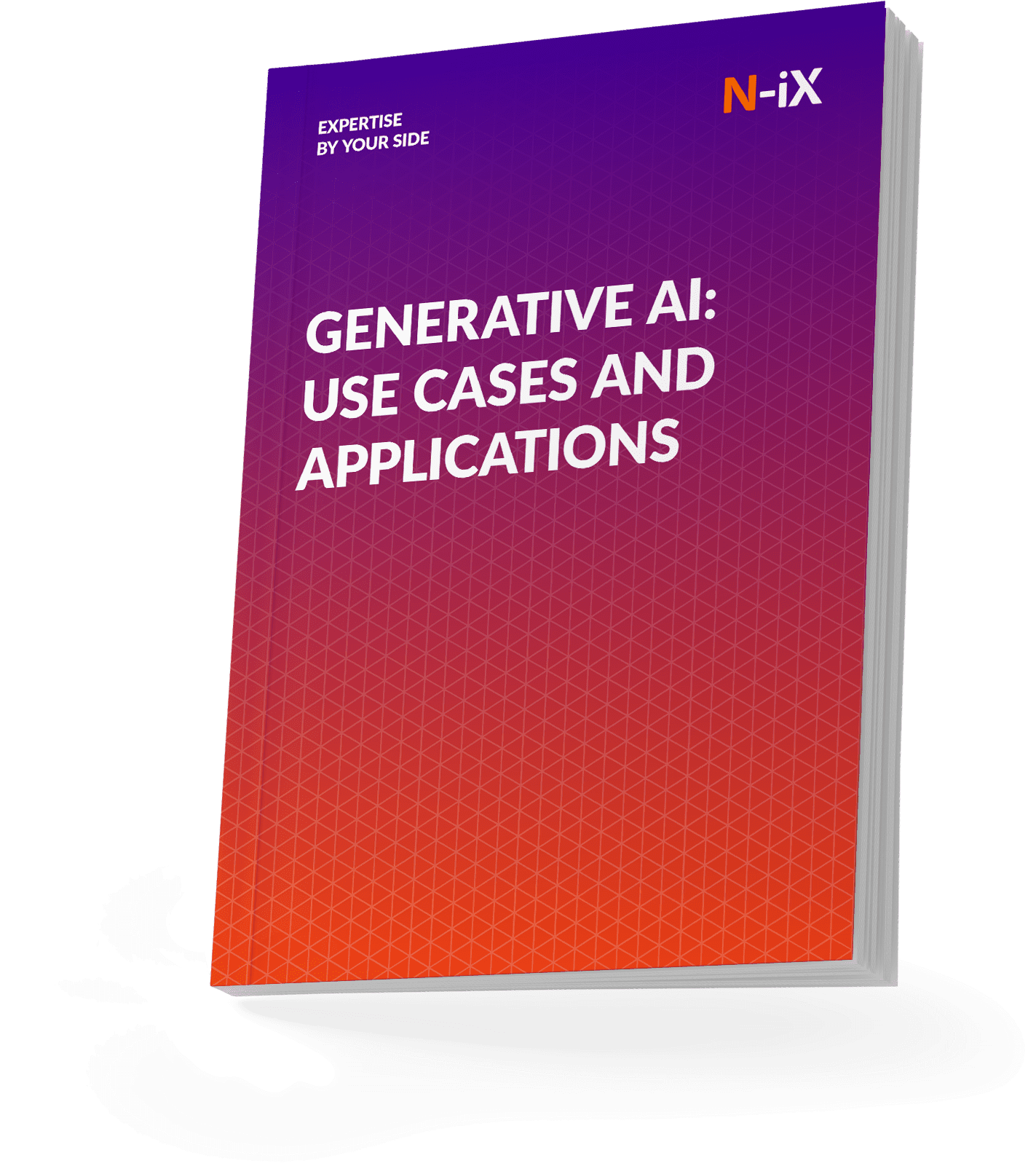
Have a question?
Speak to an expert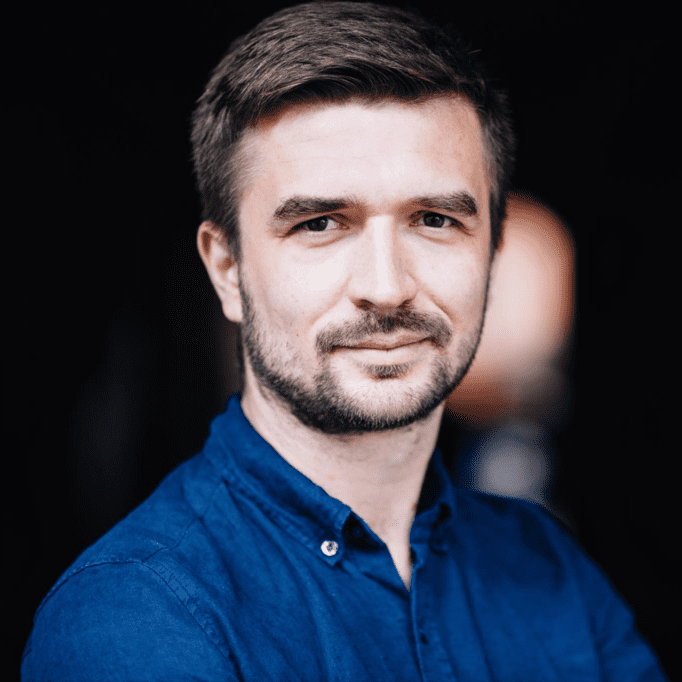