The ecommerce sector has long been at the forefront of embracing technologies. As an advanced form of machine learning, Generative AI has captured the attention of key stakeholders within the industry.
From crafting unique client experiences to refining product design, optimizing inventory management, and streamlining supply chain operations, generative AI in ecommerce is reshaping the industry in unprecedented ways.
This surge in interest and investment signifies a pivotal moment as generative AI begins to deliver tangible benefits in user experience, prompting widespread adoption among consumers. There is a unique opportunity to shape customer journeys and implement generative AI to drive positive outcomes for both customers and employees.
So, let’s take a closer look at possibilities with generative AI consulting services and address your specific business challenges, empowering you to achieve growth and efficiency.
What is generative AI in ecommerce?
Ecommerce enterprises constantly strive to enhance customer experiences, as it leads to increased conversion rates, customer reFtention, and growth. They rely on various elements, including compelling messaging, captivating visuals, prompt deliveries, and responsive customer support.
Generative AI presents opportunities to streamline operations. For instance, customers seeking assistance with product inquiries, tracking deliveries, or initiating returns can engage with AI-powered chatbots for seamless support.
While the use cases for generative AI in ecommerce continue to evolve. Forty-eight percent of businesses leveraging this technology have enhanced customer experiences by providing tailored product recommendations based on data and preferences. Moreover, generative AI enables hyper-personalized experiences at scale, meeting the growing expectation for better personalization among 73% of customers.
Generative AI in ecommerce: use cases
Below, we delve into key applications and use cases where generative AI demonstrates its prowess in enhancing efficiency, personalization, and customer engagement within the ecommerce industry.
Customized product recommendations
Generative AI algorithms analyze customer data to gain deep insights into individual preferences and behaviors. This includes previous purchases, items viewed, time spent on product pages, and interactions with marketing emails. By understanding these patterns, generative AI can generate highly personalized product recommendations that resonate with each customer on a one-to-one level. According to a study, 80% of customers are more likely to purchase when brands provide personalized experiences.
Furthermore, generative AI enables enterprises to incorporate contextual factors, such as current trends, seasonal preferences, and inventory levels, into product recommendations. This ensures that recommendations are not only personalized to individual customers but also relevant and timely based on external factors influencing purchasing decisions.
Dynamic pricing strategies
Ecommerce platforms can analyze vast amounts of data in real-time, including historical sales data, competitor pricing, customer behavior, and market trends. This comprehensive analysis enables businesses to identify patterns and correlations that traditional pricing models may overlook.
Generative AI algorithms can predict future demand fluctuations with remarkable accuracy, allowing companies to adjust prices dynamically to maximize revenue and profitability. For instance, during peak seasons like holidays or special events, generative AI can suggest strategic pricing adjustments such as personalized discounts or promotions to drive customer engagement and boost sales.
Virtual shopping assistants
Virtual shopping assistants are designed to engage with customers in real-time, guiding them through purchasing, answering questions, providing product recommendations, and assisting with decision-making. Slow responses to customer inquiries can lead to missed sales opportunities, while unavailability may convey disinterest from the brand. The response speed is important, with 90% of customers emphasizing the importance of immediate assistance, according to HubSpot Research. Unlike traditional human sales associates limited by time and resources, virtual assistants can interact with multiple customers simultaneously, providing personalized recommendations and support to each individual.
Moreover, virtual shopping assistants can be integrated across multiple channels and touchpoints, including websites, mobile apps, social media platforms, and messaging applications. This omnichannel presence ensures that customers can access personalized assistance wherever and whenever needed, enhancing convenience and accessibility.
Read more: Applications of generative AI in retail
Automated content creation
Another use case for generative AI in ecommerce is to enhance sales through content creation. This includes generating engaging and tailored content for various channels such as websites, social media, and email marketing.
Enterprises can automate the creation of product descriptions, headlines, captions, and reviews tailored to their target audience, keywords, and brand tone. These algorithms can extract key features and attributes of each product and use natural language generation techniques to craft coherent descriptions.
Product images
Generative Adversarial Networks (GANs) enable the creation of new product images to produce lifelike product visuals for ecommerce or advertising purposes. By training GANs on existing product image datasets, these networks can generate new, realistic product images suitable for advertising campaigns. This approach significantly reduces the time and resources required for product photography and image editing.
Product search
Generative AI can enhance the discovery of your product by leveraging user preferences, behavior, and purchase history to offer personalized recommendations. 82% of businesses benefiting AI for ecommerce report noticeable improvements in product discovery.
For instance, Zalando’s virtual assistant enhances product discoverability by improving tagging accuracy and facilitating conversational search. Customers can now describe what they're seeking in their own words, allowing the smart assistant to make tailored recommendations.
Virtual try-on
Virtual try-on tools powered by Generative AI bridge the gap between online and in-store shopping experiences, enabling customers to visualize how clothing items will fit and look on different body types and skin tones. Additionally, style transfer techniques empower customers to experiment with new looks by blending self-portraits with style reference images. This approach enables users to visualize and personalize their desired styles with ease.
Supply chain and inventory management
Generative AI simplifies supply chain management by analyzing sales data, demand trends, and inventory levels. These tools enable retailers to predict demand, calculate safety stock levels, and identify slow-moving inventory. Additionally, generative AI assists in running what-if scenarios to prepare for supply chain disruptions, evaluating suppliers, optimizing logistics routes, and enhancing last-mile delivery.
Analytics
Generative AI is widely employed in ecommerce to enhance the efficiency of business operations, with one of its most valuable applications being data processing and analysis. Through automation, Generative AI can identify patterns, outliers, and missing data, resulting in cleaner datasets.
Read more: Generative AI use cases and applications
Generative AI in ecommerce: challenges
Generative AI presents immense potential for revolutionizing ecommerce, but it's not without its challenges. Let's discover the complexities and obstacles encountered when integrating generative AI into ecommerce operations. From data privacy concerns to algorithm biases, we'll explore the hurdles businesses must navigate to harness the full benefits of this innovative technology.
Data quality and quantity
Generative AI models rely heavily on high-quality data for effective training and accurate predictions. However, ecommerce datasets often exhibit challenges such as incompleteness, inconsistency, and bias. Only complete or accurate data can lead to good outcomes, as evidenced by a 2022 MIT study reporting that 85% of AI projects fail due to poor data quality.
Biased data can impose flawed model outputs, impacting the reliability and effectiveness of generative AI applications. Ecommerce businesses must invest resources in data cleaning, augmentation, and curation processes to ensure training data quality and diversity, thereby improving the performance and robustness of generative AI models.
Ethical considerations and bias mitigation
Generative AI models may potentially access, utilize, or expose sensitive or personal data belonging to the ecommerce business or its customers, including preferences, behaviors, identities, and financial information. This poses significant risks and raises apprehensions regarding privacy and security protocols.
Generative AI introduces ethical considerations regarding bias and fairness in the generated content. Biases present in training data can propagate into model outputs, resulting in discriminatory or misleading outcomes. Ecommerce businesses must implement robust bias detection, mitigation, and fairness evaluation mechanisms throughout the AI development lifecycle.
Integration complexity with existing systems
Ecommerce platforms often comprise a complex ecosystem of interconnected applications, databases, and infrastructure components. Ensuring seamless integration of generative AI functionalities requires compatibility with existing technologies, data interoperability, and synchronization across disparate systems. Companies must develop comprehensive integration strategies, leveraging APIs, middleware, and microservices architectures to facilitate interoperability and data exchange between generative AI systems and existing platforms.
Resource intensiveness
Generative AI models demand substantial computational resources and infrastructure for training and inference tasks. Training complex deep learning models on large-scale datasets requires high-performance computing clusters, specialized hardware accelerators (e.g., GPUs, TPUs), and scalable cloud infrastructure.
Ecommerce businesses must invest in robust computing resources and infrastructure provisioning to support the computational demands of generative AI algorithms. Optimizing model architectures, implementing distributed training techniques, and leveraging cloud-based services can help mitigate resource constraints and improve scalability and efficiency in generative AI deployments.
Wrapping up
84% of organizations that have fully integrated AI into their ecommerce operations report significant improvements. Companies like AWS, Sephora, Alibaba, and Amazon already leverage generative AI solutions to enhance their operations and improve customer experiences. With the proven success of generative AI in various industries, businesses should not hesitate to explore its potential for growth and innovation.
With a proven track record of over 2,200 engineers and 21 years on the market, N-iX offers tailored expertise to drive sustainable business growth and productivity in your company. Don't wait to contact N-iX—embrace innovation and unlock the future of ecommerce today.
Have a question?
Speak to an expert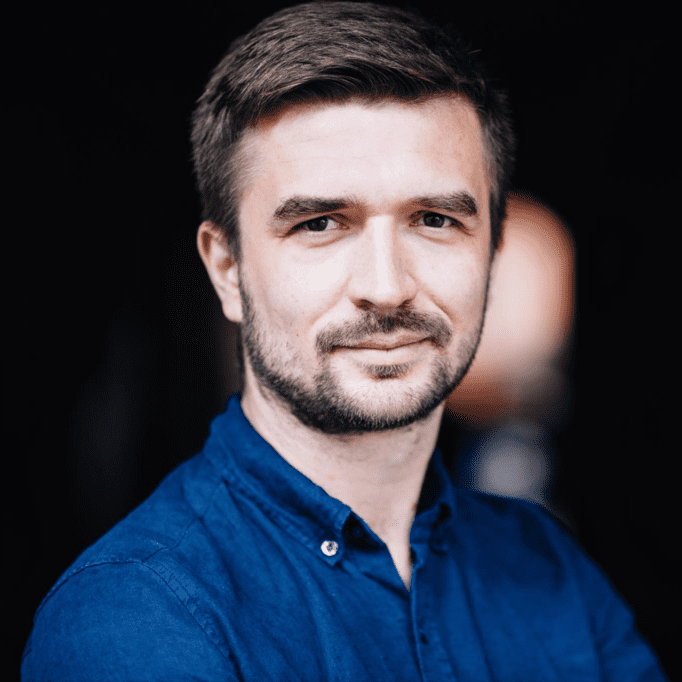