In the three months of fall 2023, Morgan Stanley, JPMorgan Chase, Citigroup, and Goldman Sachs all announced their custom internal generative AI tools. Although these institutions were the first to implement AI technology, the entire financial industry acknowledged its impact. The market for generative AI in banking is expected to double in the next three years [1], inadvertently changing how financial services are delivered.
Let's explore the applications of generative AI in banking and the obstacles preventing banks from leveraging its full potential.
Use cases of generative AI in banking
Based on the initiatives of the leaders of the American banking market, the first big change generative AI is bringing is automating report generation and document analysis. Automating critical but time-consuming tasks saves labor costs, improves accuracy, reduces processing times, and enhances overall quality. 37% of executives state that their organization is considering AI for report generation. Another third is exploring AI to improve customer experience, generate synthetic data for model training and cybersecurity, and create marketing materials. Yet, generative AI possesses an even larger capacity to revolutionize the industry. Here are the biggest current and future use cases of generative AI in banking:
Document processing
In banking, document processing involves handling diverse documents such as loan applications, KYC documents, contracts, and regulatory filings. Generative AI can revolutionize this process by automating the extraction, interpretation, and classification of information from these documents. It can swiftly identify relevant data points, evaluate document completeness, verify information against predefined criteria, and even detect anomalies or compliance issues. It can then communicate the information extracted in simple human language and even answer questions about it.
Furthermore, generative AI can automate the whole process of gathering data from various sources, analyzing it, and then producing insightful, coherent reports. These reports can cover financial statements, market analysis, risk assessments, and personalized customer financial advice. Generative AI can adapt the depth, tone, and format of these reports to meet specific stakeholder needs, ensuring they are precise and easily understandable.
Personal assistants and personalization
In the same way that document processing models assist employees, generative AI can be used to create sophisticated client-facing personal assistants. They can help with account management, transaction inquiries, product recommendations, and financial advice tailored to the individual's financial goals and needs. The personal touch enhances the customer experience, fostering loyalty and satisfaction. Unlike traditional bots or human assistants, AI-based chatbots can immediately consider all relevant information, be fast and intelligent simultaneously, and provide a reliable service 24/7.
The ability to analyze vast amounts of real-time data, including transaction history, browsing behavior, and personal preferences, makes generative AI in banking a core component of hyper-personalization. For instance, an AI assistant can suggest a credit card with benefits that align with a customer's spending habits or offer a loan with terms tailored to their financial history. According to Forrester, banks that have established hyper-personalization as a part of their strategy report impressive outcomes, including tripling their ability to cross-sell products and customer acquisition.
Synthetic data generation in model training and cybersecurity
Gen AI-powered synthetic data generation creates artificial data sets that mimic the statistical properties of real data to train financial or other models. When real customer data is used, there's a heightened risk of exposing sensitive information to unauthorized parties, resulting in severe legal and financial repercussions for the bank. By contrast, synthetic data generation addresses these risks by creating artificial datasets that mimic the statistical properties of real data without containing any actual customer information. It can then be adjusted to solve issues with censorship, truncation, or bias in data.
Additionally, synthetic data allows for the simulation of scenarios that may not be readily available in historical data. This includes testing systems against rare but potentially impactful financial conditions, fraud attempts, or operational stress scenarios, thereby ensuring that all systems are robust and can handle a wide range of challenges. For instance, Goldman Sachs has already implemented an AI-driven tool for automating test generation, replacing what was previously a manual and labor-intensive procedure.
Real-time fraud prevention
Generative AI is excellent at identifying complex patterns and anomalies in extensive datasets. It can recognize subtle shifts in customer behavior, transaction frequencies, amounts, or locations that differ from the norm. A key strength of generative AI in fraud prevention is its adaptability. As it encounters new types of fraud, the AI system updates its models to identify these patterns. This ensures that the bank's fraud detection capabilities improve continuously and stay ahead of the cybersecurity arms race.
Applications in capital markets
Generative AI can significantly streamline the data analysis process involved in capital markets. A model can lead a client through onboarding, analyze their assets, research the market, develop an investment strategy, and service the assets. The most groundbreaking aspect of generative AI in capital markets is its potential to manage these processes completely independently, offering a level of speed, efficiency, and personalization that traditional manual processes cannot match.
This is not an exhaustive list, generative AI is revolutionizing banking by automating complex processes, enhancing customer experiences, and improving decision-making in every area imaginable. Among all use cases of generative AI in banking, 43% of executives report improved operational efficiency, and 42% report gaining a competitive advantage [2].
Why isn’t generative AI everywhere yet?
According to KPMG [3], 60% of US-based banks are not implementing generative AI because of the lack of talent, cost, and data privacy considerations. It is important to fully understand what AI technology entails and what measures should come together with generative AI implementation to ensure maximum efficiency and safety. Here are the biggest considerations for implementing generative AI in banking:
Bias and lack of explainability
The nature of AI models presumes that the statistical probabilities behind their decisions are inaccessible. For instance, if a bank employs AI algorithms for loan approvals, it could unintentionally discriminate against particular demographics because of biased training data. The absence of explainability can cause customers and stakeholders to be uncertain about the decision-making process, leading to a lack of trust in the system.
To reduce the risk of algorithmic bias, diversifying the training data and including fairness metrics in the model evaluation process are essential steps. Regular audits and updates based on feedback loops can help identify and correct biases, ensuring the AI systems operate more equitably over time. Additionally, educating users about the strengths and limitations of AI models can help set realistic expectations about their explainability.
Increased requirements for security and data governance
The integration of AI&ML models entails increased demands for security and data governance. With AI systems processing vast amounts of sensitive financial data, robust security measures are imperative to safeguard against cyber threats and data breaches. Additionally, stringent data governance practices are necessary to ensure compliance with regulations such as GDPR and CCPA, especially considering the potential risks associated with unauthorized access or misuse of data. Implementing comprehensive security protocols and adhering to strict data governance frameworks are essential to mitigate risks and maintain trust in AI-driven banking systems.
Privacy сoncerns
Privacy concerns surrounding generative AI can be exacerbated by the utilization of personal or sensitive data during model training, possibly leading to unintended consequences. For instance, if a financial institution employs AI algorithms trained on customer data to personalize services, there's a risk of unauthorized access or misuse of sensitive information. Integrating privacy considerations into the development process from the ground up, known as Privacy by Design, can help preemptively address potential privacy issues.
A successful generative AI implementation journey begins with developing an AI strategy. It should align with the overarching business objectives and operational workflows. Carefully planning and executing an AI strategy helps overcome the challenges associated with generative AI implementation and harness its potential.
How N-iX helped streamline operations in financial services
N-iX has created a custom generative AI solution for a prominent low-fee brokerage firm to enhance operational efficiency and increase productivity. Our engineers have developed a chatbot that can extract information from the combined database, which pulls information from the company's website, internal documents, security manuals, and Confluence. Our solution helped save employees’ time browsing diverse sources of information and provide the maximum relevant answer with speed and accuracy.
Read more: Streamlining operations and boosting efficiency in finance with generative AI
Wrap up
Since the general public first experienced pre-trained generative AI models, it has become clear that generative AI is a transformative force set to spur growth across many industries, including banking. Generative AI is redefining traditional banking paradigms by automating processes, providing personalized customer interactions, and improving security through advanced analytics. Its adoption signifies a change in banking operations and a larger shift towards more agile, customer-focused, and innovative financial services.
References:
- Generative AI in banking market projections, MarketResearch 2022
- State of AI in Financial Services, Nvidia 2024
- 2023 KPMG Generative AI Survey
Have a question?
Speak to an expert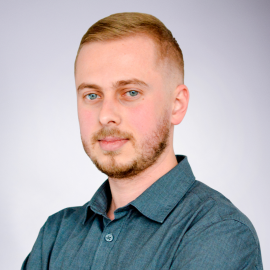