What happens when a company invests in analytics tools, but still can't get a consistent view of its customers? Or when different teams report different numbers for the same KPI? Data initiatives often launch in isolation, undertaken by individual departments or technology teams, without a shared roadmap that links business priorities, governance, architecture, and execution. It's the lack of a clear, structured plan that connects data capabilities to real business goals.
Developing a strategy is a foundational step. But for that strategy to deliver value, it must be backed by a detailed and realistic roadmap: one that is documented, communicated, and executed. A data strategy roadmap provides all of that. It brings context to enterprise data efforts. It defines what needs to be built, why it matters, who owns it, and how it will scale across systems, teams, and time.
Data strategy consulting takes the lead and brings the structure, expertise, and alignment to cross-department operations. This article offers a detailed, practical view of what a data strategy roadmap includes and how enterprise leaders can create one.
What is data strategy roadmap?
A data strategy roadmap is developed to support the organization's broader vision and long-term business goals. It is a detailed plan for how data will be acquired, integrated, secured, stored, managed, monitored, governed, analyzed, and used across the enterprise. It outlines the operational and technical methods, services, and architectures needed to support these efforts.
The enterprise data strategy roadmap focuses on execution. It breaks down the broader strategic vision into specific, actionable steps with defined timelines and deliverables. The roadmap operationalizes the framework by detailing how initiatives will be prioritized, resourced, and tracked over time.
The roadmap defines what needs to be changed, why it matters, and in what order it should happen. From there, a data strategy roadmap typically addresses goals such as:
- Data lifecycle management from acquisition to archiving
- Elimination of duplicated efforts and fragmented technology investments
- Establishment of governance standards that support compliance and auditability
- Implementation of a scalable, secure architecture aligned with future business needs
- Consistent use of data as a foundation for enterprise-wide decision-making
Foundation of a data strategy roadmap: strategy and vision
At the core of any data management strategy roadmap is a structured blueprint that aligns data initiatives with business priorities, capabilities, and long-term transformation goals. A mature roadmap should yield five essential deliverables. Each addresses a foundational question that guides both vision and execution:
Data vision
This defines how data will transform your business model, customer engagement, operations, and decision-making processes. It contextualizes your strategy within industry shifts, whether it's regulatory evolution, platform competition, or the acceleration of AI, and identifies where data can become a strategic enabler or a barrier if left unaddressed. This vision must also account for external pressures: what new digital-native competitors are emerging, and how do they weaponize data differently?
Competitive advantage assessment
This component analyzes how your current data capabilities affect your market position. Where does your organization benefit from strong data maturity, integration, or insight velocity and where do you lag? Whether it's data latency in decision workflows or the absence of enterprise-wide data literacy, this step surfaces structural strengths and weaknesses that directly affect business performance.
Prioritized portfolio of data use cases
Not every data initiative holds equal value. This part of the roadmap identifies and prioritizes specific, business-aligned use cases that are feasible, high-impact, and consistent with your enterprise strategy. These "data bets" must be sequenced based on resource constraints, technical dependencies, and expected ROI. Use cases may include customer 360 platforms, predictive maintenance, AI-driven risk scoring, or real-time supply chain visibility, each anchored in a measurable business outcome.
Gap assessment
Once initiatives are prioritized, the roadmap must assess the organizational readiness to deliver them. This process requires reviewing data infrastructure, governance maturity, talent models, and integration with enterprise systems. Are foundational layers such as metadata, lineage, access control, and quality management in place? Are data stewards, product owners, and analytics teams aligned with delivery requirements? This analysis defines where targeted investment is needed to close capability gaps.
Roadmap and execution model
The final component is a phased, time-bound roadmap with clear initiatives, ownership structures, performance metrics, and governance checkpoints. It provides the framework for the delivery, what must be achieved in 6, 12, and 24 months, and aligns it with budget planning, change management, and enterprise steering mechanisms. It also outlines how to fund and sustain the journey, including cost distribution, benefit realization, and resource reallocation models.
Taken together, these five deliverables establish a comprehensive foundation for moving from strategic intent to scalable implementation. For enterprise leaders, they offer a vision of what the organization can become through data and a straightforward, disciplined approach for getting there.
How to build a data strategy roadmap: execution plan
Phase 1: Strategic framing and executive alignment
This phase establishes strategic clarity, aligns leadership, and mobilizes cross-functional collaboration. It sets the course for how data will drive business outcomes across the enterprise operating model. A robust execution plan begins here, ensuring that strategy, investment, and delivery are consistently linked. This is not a planning phase to be treated as static or siloed; it is the basis for scalable and sustainable value creation.
During this period, three interconnected outputs must be shaped:
1. Initial draft of the data strategy
A concise but structured articulation of how data supports business priorities. It outlines the strategic rationale, defines the role of data in enterprise transformation, and provides a directional foundation for architecture, governance, and execution planning.
2. Prioritized implementation roadmap
A high-level timeline that sequences initiatives based on business impact, technical readiness, and organizational feasibility. This is a structured prioritization logic that connects use cases to outcomes.
3. Preliminary business case
An initial investment rationale that quantifies costs and expected value across short- and mid-term horizons. It outlines value levers, resource requirements, and how data initiatives will fit within existing planning and funding cycles.
A credible data strategy specifies the structure, functions, and capabilities required to activate and sustain a data-driven operating model. It translates enterprise goals into system-level data mandates. Key components include:
- Business alignment is established by identifying areas where data can deliver a measurable impact, such as increasing customer lifetime value, improving forecasting accuracy, or automating compliance reporting.
- Operating model design clarifies whether data and analytics will be structured as a centralized, federated, or hybrid function and how this structure will interact with broader enterprise IT, business lines, and transformation initiatives.
- Architecture direction provides a reference model for the target-state data infrastructure, typically encompassing data lakehouses, real-time streaming pipelines, API orchestration layers, and distributed storage systems. Transitional states and phased modernization plans are outlined to bridge current capabilities with future requirements.
- Governance structure includes allocating stewardship responsibilities, defining data access policies, data lifecycle practices, and compliance enforcement mechanisms, spanning technology and business ownership.
- Platform capabilities are assessed to determine the foundational elements required to meet near-term priorities and long-term scale, such as data catalogs, lineage tracking, semantic layers, master data management (MDM), observability tooling, and feature stores.
- Organization and team roles are reviewed to surface current capability gaps. This involves mapping existing teams and defining future-state roles, including data product managers, platform leads, solution architects, domain data owners, and stewards.
- Dependencies and constraints are documented to account for regulatory requirements, technical debt, vendor timelines, integration with legacy systems, and dependencies on parallel initiatives across the organization.
Take 7 steps to design a winning data governance strategy—get the guide now!
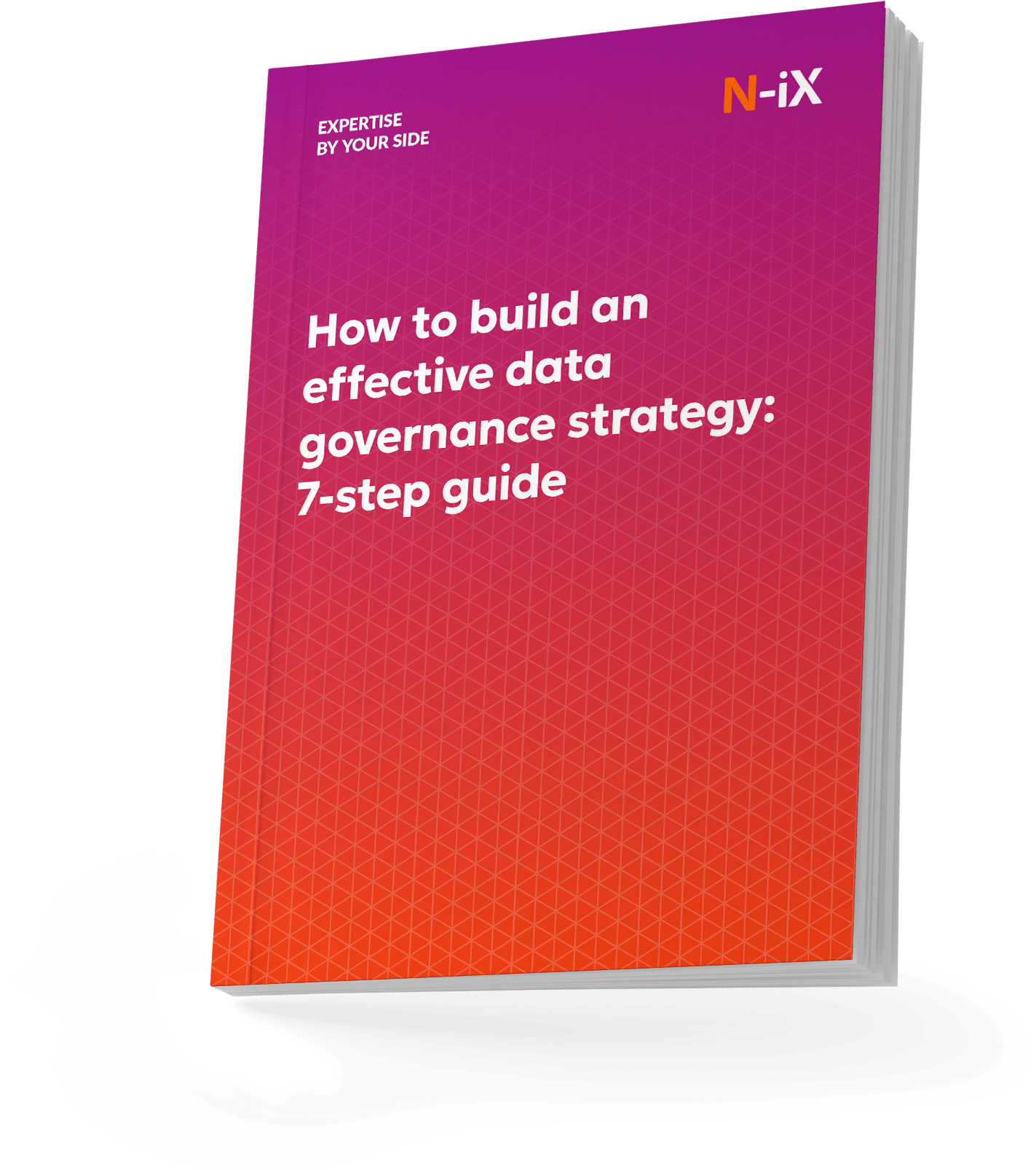
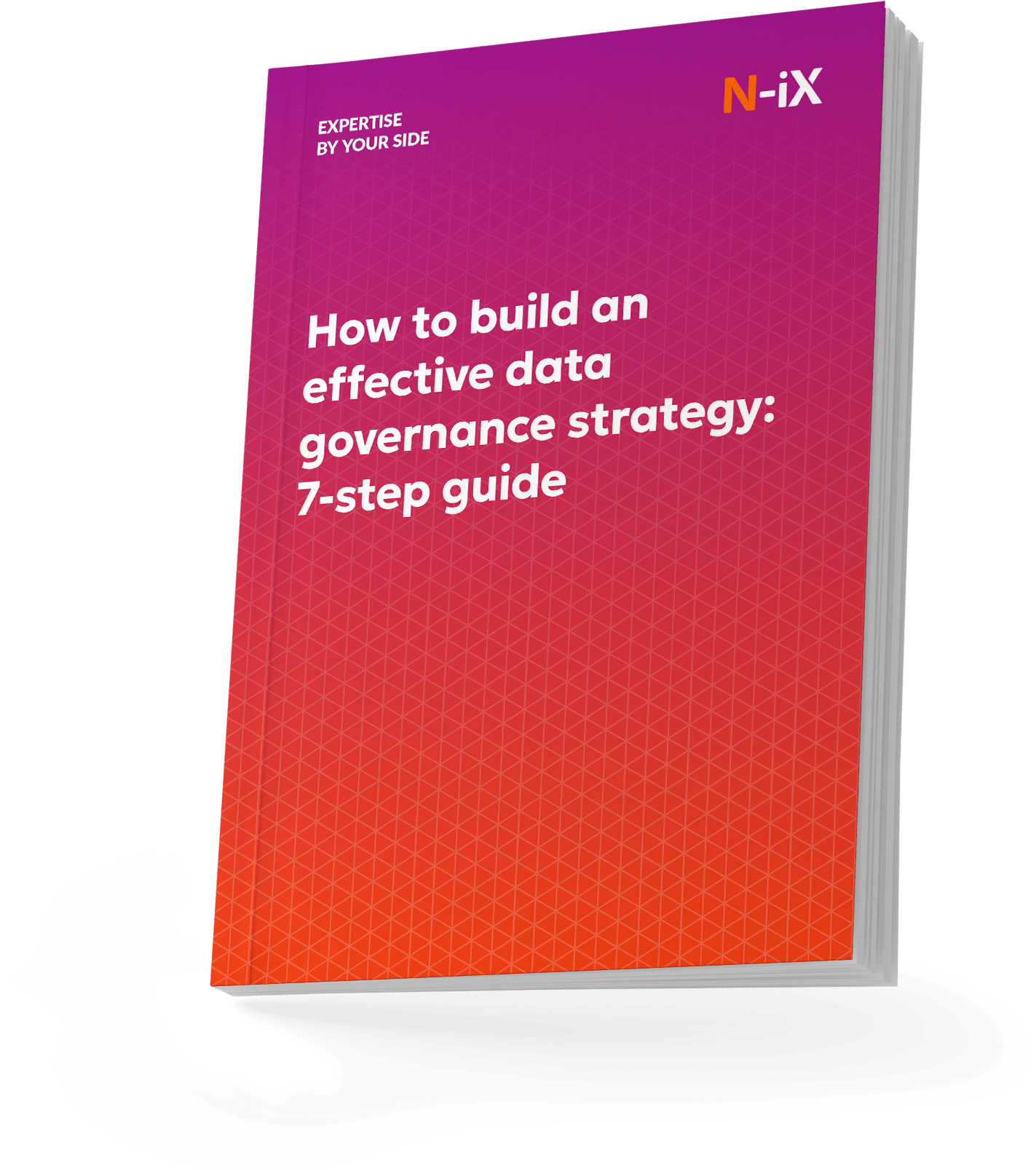
Success!
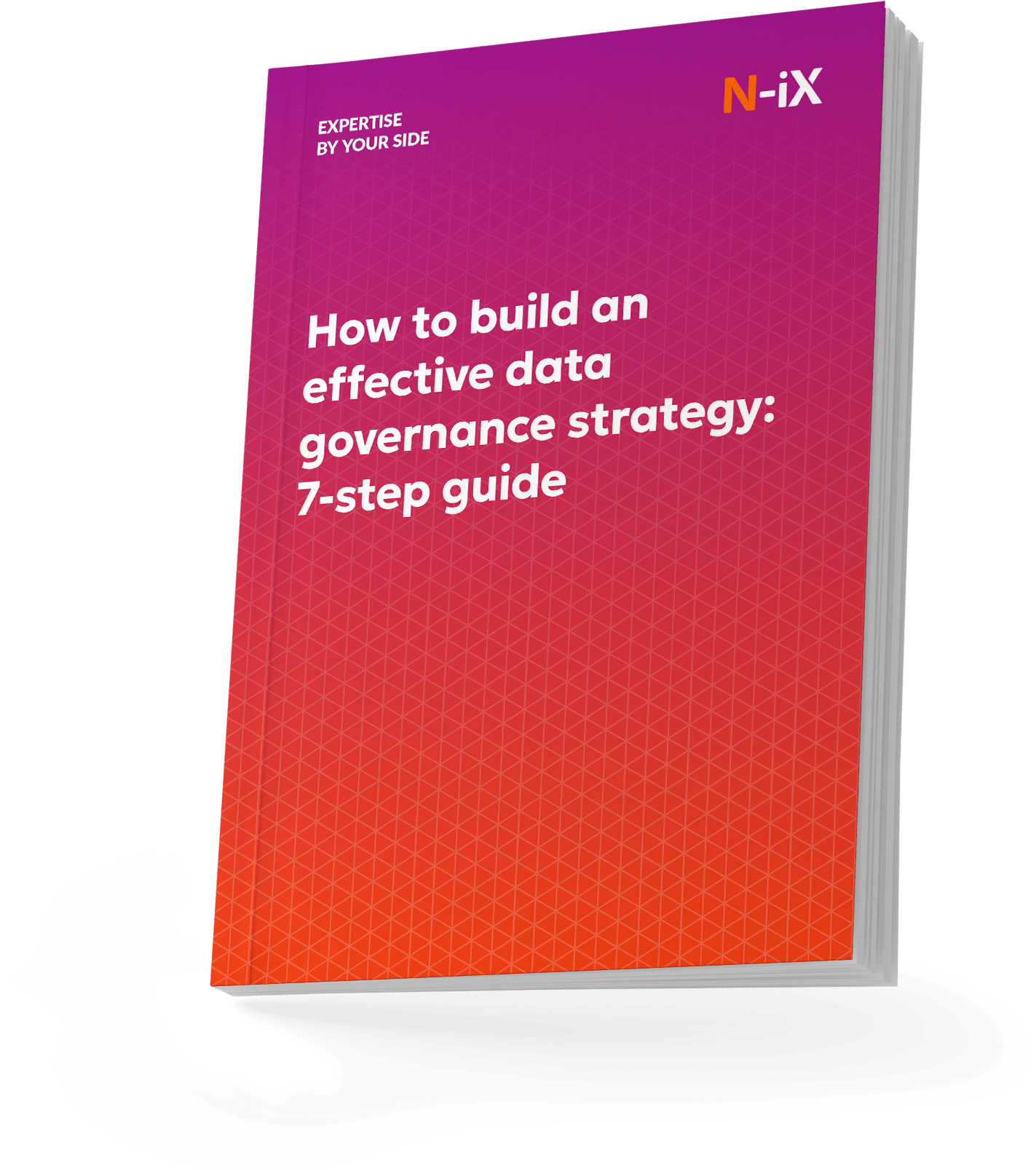
Phase 2: Designing and validating the execution
Following the initial 4-6 week phase of strategic definition and roadmap framing, the next step is translating high-level vision into an actionable delivery structure. This stage marks the shift from strategy formulation to practical validation, where planning assumptions are stress-tested, interdependencies surface, and the organization begins to evaluate whether it is operationally and technically prepared to deliver on its data ambitions.
At this point, the draft roadmap must evolve into a sequenced and time-bound execution plan. This requires mapping out dependencies across systems, data domains, and functions while aligning with existing transformation programs, budget planning cycles, and resource availability. Prioritization becomes increasingly pragmatic: foundational capabilities, such as core data integration, cataloging, lineage, or access controls, need to be addressed first, followed by enablers like quality monitoring, metadata management, or data product development. More advanced initiatives, including predictive models, self-service analytics, or generative AI pilots, are typically scheduled later in the sequence once the groundwork is in place.
Refining the roadmap also means identifying pilot initiatives that can be executed with the current architecture and talent model. These pilot efforts serve multiple purposes: they demonstrate value early, validate key elements of the operating model, test integration points, and provide feedback loops for adjusting future phases. Pilots are carefully selected based on organizational priorities, data readiness, and cross-functional alignment. Each pilot should include:
- Direct alignment with business priorities and stakeholder goals
- Clear success metrics tied to operational or financial outcomes
- Defined data inputs, data flows, and required governance measures
- A feedback mechanism to capture lessons learned and refine execution
- A scaling plan if the pilot proves successful
During this phase, coordination between delivery and governance becomes essential. The data governance program, often informal or loosely defined during the planning phase, must begin to take shape. This involves clarifying data ownership, establishing decision rights, and implementing oversight mechanisms. Organizations typically formalize cross-functional steering groups at this stage to provide governance continuity across workstreams, manage risks, and ensure business units remain engaged in roadmap execution.
The operating model is also pressure-tested. For organizations with federated structures, this phase reveals where central enablement must support distributed execution. More centralized models clarify which responsibilities must shift toward business units to enable scale and agility. Any gaps in capabilities, whether in skills, tooling, or organizational support, become more visible and must be addressed in parallel.
By the end of this phase, the organization should have a validated execution model, a sequenced roadmap for the next 6-24 months, and the foundational governance and resourcing mechanisms in place to sustain delivery.
Phase 3: Scaling and institutionalizing
Execution at scale begins with solidifying the operating model. Indeed, it means institutionalizing data ownership, stewardship, and quality management into business functions. What may have started as centralized governance or a handful of pilots must now be translated into defined responsibilities across departments, reinforced by transparent processes and enterprise-aligned KPIs.
Each business unit should adopt a tailored data stewardship model supported by enterprise standards. Federated ownership models become more prominent, allowing business-aligned teams to manage local data initiatives while a central function continues to guide architecture, interoperability, and compliance.
In practice, this phase often includes:
- Formalizing data stewardship roles within business domains
- Integrating KPIs from the roadmap into business unit performance tracking
- Scaling agile delivery for data and AI use cases
- Ensuring continued adherence to enterprise data architecture principles
With the delivery engine functioning and governance embedded, the roadmap must support continuous innovation. New use cases, such as advanced analytics, AI agents, customer intelligence platforms, and automation, should build on existing capabilities, not bypass them. Data lineage, metadata, access control, and quality standards must be maintained across all initiatives. Innovation should build on the data governance foundation, not operate in isolation.
At the same time, lessons from previous phases must be formalized. Playbooks, design patterns, and reusable components reduce friction and improve speed for new initiatives. Business and IT planning cycles should also be aligned to ensure synchronization between operational objectives and the data analytics strategy roadmap.
Discover more about: Big Data strategy: How to achieve data-driven success
What deliverables are expected from a data strategy roadmap?
A well-developed roadmap is a structured portfolio of interconnected deliverables that collectively translate strategic intent into executable reality. These data strategy roadmap examples ensure alignment across business, technology, and governance layers, clarifying where the organization is headed, how it will get there, and what success will look like.
Governance framework
A structured model for decision rights, data ownership, stewardship, access control, and policy enforcement. It establishes accountability across business and IT functions, details data quality, privacy, and compliance mechanisms, and supports operational scalability as data maturity evolves.
Capability maturity assessment
An objective evaluation of current-state capabilities across architecture, governance, analytics, integration, and organizational design. This baseline informs data roadmap prioritization, highlights capability gaps, and defines what capabilities must be developed to support future-state use cases.
Prioritized implementation backlog
A sequenced list of initiatives aligned to business value, technical feasibility, and strategic dependencies. This backlog reflects short-, mid-, and long-term priorities and offers transparency into why each item exists, what it will deliver, and how it connects to overarching goals.
Data architecture diagrams
Technical visualizations that capture the current state of the organization's data ecosystem and outline the target-state reference architecture. These diagrams in the data strategy roadmap template highlight infrastructure gaps, system dependencies, and the transitional states required to modernize platforms, integrate sources, and enable analytics at scale.
Execution plan
A time-bound delivery structure that maps initiatives to milestones, resource needs, and accountable owners. At the highest level, the roadmap should summarize the organization's data transformation agenda, highlighting critical outcomes, sequencing, focus areas, and expected timelines. This view helps leadership maintain alignment with strategic business objectives while tracking progress at a glance.
The next level of detail should define the interdependencies among initiatives, estimate durations, identify foundational enablers, and group efforts by key domains such as governance, analytics, infrastructure, and talent. For example:
- Sequencing: Activities like ingesting raw data into a lake must precede downstream efforts such as semantic modeling or AI enablement.
- Timeframes: Complex initiatives, such as advanced analytics on multimodal data, may require sustained development over 6+ months.
- Dependencies: Business-facing use cases, such as self-service dashboards, depend on foundational work like metadata management and data cataloging.
- Categorization: Each initiative should fall into strategic buckets (e.g., governance, architecture, reporting, business enablement), aiding prioritization and funding alignment.
Common challenges when creating a data strategy roadmap
Even with a clear vision, building a data analytics strategy roadmap presents execution risks, especially in enterprise environments where multiple teams, priorities, and legacy systems are involved. Many roadmaps fail not because of poor intent but because of gaps in ownership, structure, or business strategy, as well as alignment of data management efforts. Below are common challenges organizations face when creating and executing a roadmap:
- Overly technical or overly abstract roadmaps fail to bridge execution with value, resulting in solutions misaligned with business priorities or too conceptual to implement.
- Insufficient business ownership, when data roadmaps are built solely by IT, leads to poor adoption, fragmented execution, and a lack of enterprise-wide accountability.
- Initiatives untethered from measurable business outcomes result in data investments that consume resources without influencing revenue, cost optimization, or innovation capacity.
- Governance introduced late or not at all undermines trust in data, exacerbates compliance risk, and stalls the scaling of analytics and AI.
- Tool-first strategies without accompanying process or governance design create redundant investments, operational silos, and low-value deployment of platforms.
- Persistent misalignment between business and IT leadership erodes decision velocity, creates accountability gaps, and reinforces the perception of data as a cost center rather than a strategic asset.
- Lack of structured prioritization leads to initiative overload, diluted resource allocation, and delayed value realization across the portfolio.
How do we approach building a data strategy roadmap? N-iX embeds strategic alignment mechanisms throughout the roadmap development lifecycle. Our approach incorporates stakeholder co-creation workshops, value-stream mapping to tie data initiatives to quantifiable business metrics, and early-stage governance design aligned with industry regulations (e.g., GDPR, HIPAA, PCI DSS). We also operationalize roadmaps through iterative delivery frameworks and adaptive planning cycles, ensuring the strategy remains relevant and actionable as business conditions evolve.
Bottom line
If you see signs of stalled progress, such as siloed data efforts, slow time to value, or uncertainty about where to focus next, it's not too late to course correct. The key is to stop reacting and start planning with precision. A well-structured data roadmap brings focus, prioritization, and direction. It identifies which capabilities need to be developed, in what order, and how they contribute to long-term value creation.
N-iX works with organizations at every stage of data maturity. Whether starting from a fragmented data landscape or refining a long-term vision, our team helps you assess where you stand, identify what's missing, and chart a roadmap that increases value.
Have a question?
Speak to an expert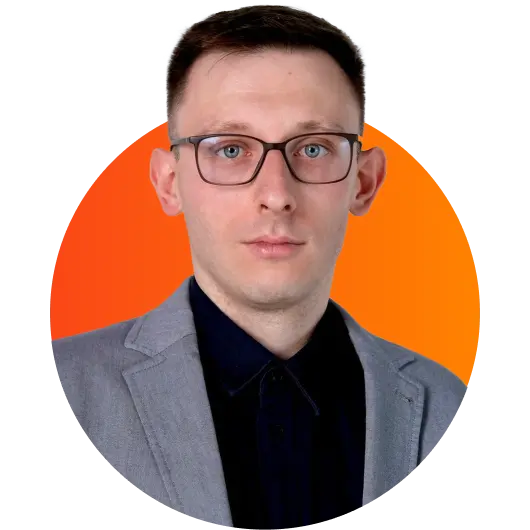